Organizing Committee
- Nan Jiang
University of Illinois Urbana-Champaign - Sanjay Shakkottai
University of Texas Austin - R. Srikant
University of Illinois at Urbana-Champaign - Mengdi Wang
Princeton
Abstract
There has been significant progress over the last few years in the theory and applications of Reinforcement Learning (RL). While RL theory and applications have had a rich history going back several decades, the major recent successes have occurred due to a successful marriage between deep learning approaches for function approximation embedded within a reinforcement learning framework for decision-making (Deep RL). On one hand, there has been a richer understanding of Stochastic Gradient Descent (SGD) for non-convex optimization, its impact in driving training error to zero in deep neural networks, and on the generalization ability of such networks for inference. On the other hand, there has been an explosion of research on iterative learning algorithms with strong statistical guarantees in the settings of reinforcement learning, stochastic approximation and multi-armed bandits.
This workshop aims to bring leading researchers from these two threads, with the goal of understanding and advancing research at their intersection. We will also explore other potential connections between deep learning and deep RL, including but not limited to: Understanding generalization in deep RL and how it is related to and/or different from generalization in deep learning; Connections between adversarial training in deep learning (e.g., Generative Adversarial Networks) and the optimization aspects of recent deep RL algorithms based on generalized moment matching in off-policy RL and imitation learning.
This workshop is fully funded by a Simons Foundation Targeted Grant to Institutes.
Note: There will be no poster session for this workshop. Thus, graduate students are NOT required to provide statements of support or poster session information during the application process.
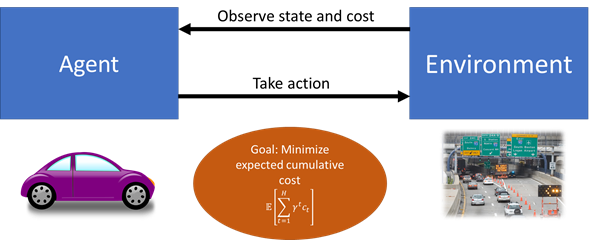
Confirmed Speakers & Participants
Talks will be presented virtually or in-person as indicated in the schedule below.
- Speaker
- Poster Presenter
- Attendee
- Virtual Attendee
-
Rebecca Adaimi
University of Texas at Austin
-
Saghar Adler
University of Michigan
-
Mohammad Afshari
University of Alberta
-
Alekh Agarwal
Microsoft
-
Naman Agarwal
Google
-
Shubham Aggarwal
University of Illinois Urbana Champaign
-
Priyank Agrawal
University of Illinois at Urbana Champaign
-
Abdullah Alawad
University of Illinois Urbana Champaign
-
Elie Alhajjar
US Military Academy
-
Awni Altabaa
Queen's University
-
Philip Amortila
University of Illinois at Urbana-Champaign
-
Andreas Aristotelous
The University of Akron
-
Dilip Arumugam
Stanford University
-
Debangshu Banerjee
Indian Institute of Science
-
Arundhati Banerjee
Carnegie Mellon University
-
Partha Basumallick
Saha Institute of Nuclear Physics
-
Mikhail Belkin
UCSD
-
Raja Ben Hajria
Faculty of sciences of Monastir
-
Emanuel Bendavid
US Census Bureau
-
Ghanshyam Bhatt
Tennessee State University
-
Jose Blanchet
Stanford University
-
Emma Brunskill
Stanford University
-
Yuheng Bu
MIT
-
Lucas Buccafusca
University of Illinois at Urbana-Champaign
-
Semih Cayci
University of Illinois at Urbana-Champaign
-
Gourab Chatterjee
Government College of Engineering and Ceramic Technology
-
Xiaohui Chen
University of Illinois at Urbana-Champaign
-
Jinglin Chen
University of Illinois at Urbana-Champaign
-
Yuguo Chen
University of Illinois at Urbana-Champaign
-
Zaiwei Chen
Georgia Institute of Technology
-
Yuxin Chen
Princeton University
-
Hongmei Chi
Florida A&M University
-
David Choi
Carnegie Mellon University
-
Anirudh Choudhary
University of Illinois Urbana-Champaign
-
Asaf Cohen
University of Michigan
-
Liam Collins
UT Austin
-
Hossein Dabirian
University of Michigan
-
Mehran Dibaji
MIT
-
Thinh Doan
Virginia Tech
-
Shanna Dobson
University of California at Riverside
-
Akash Doshi
University of Texas at Austin
-
Ryan Dreifuerst
The University of Texas at Austin
-
Yihao Feng
University of Texas at Austin
-
Cristina Garbacea
University of Michigan
-
Kunal Garg
University of California, Santa Cruz
-
Horacio Gomez-Acevedo
University of Arkansas for Medical Sciences
-
Pedro González Rodelas
University of Granada
-
Aditya Gopalan
Indian Institute of Science
-
Akshit Goyal
University of Minnesota
-
Christopher Grimm
University of Michigan
-
Adam Gronowski
Queen's University
-
Utkarsh Gupta
University of Michigan - Ann Arbor
-
Shikhar Gupta
University of Michigan
-
Shuo Han
University of Illinois at Chicago
-
Botao Hao
Deepmind
-
Elad Hazan
Princeton University
-
Niao He
ETH Zurich
-
Chaozhe He
University of Michigan
-
Nasimeh Heydaribeni
University of Michigan
-
Hassan Hmedi
The University of Texas at Austin
-
Boya Hou
University of Illinois at Urbana-Champaign
-
Eric Hsiung
Brown University
-
Haque Ishfaq
McGill University
-
Olaniyi Iyiola
Clarkson University
-
Shashwat Jain
Cornell University
-
Yajit Jain
Brown University
-
ARPIT JAISWAL
University of MICHIGAN
-
Nan Jiang
University of Illinois Urbana-Champaign
-
Sham Kakade
University of Washington
-
Stella Kampezidou
Georgia Institute of Technology
-
Joseph Kao
University of Michigan
-
Brendan Keith
Lawrence Livermore National Laboratory
-
Nouman Khan
University of Michigan, Ann Arbor
-
Rajiv Khanna
UC Berkeley
-
sajad khodadadian
Georgia Institute of Technology
-
KyungMin Ko
Purdue University
-
Murat Kocaoglu
Purdue University
-
Sanmi Koyejo
University of Illinois at Urbana-Champaign
-
Pankaj Kumar
Copenhagen Business School
-
Jeongyeol Kwon
University of Texas at Austin
-
Triet Le
The National Geospatial-Intelligence Agency
-
Kiyeob Lee
Texas A&M University
-
Kang-Ju Lee
Seoul National University
-
Jason Lee
Princeton
-
Alessandro Leite
INRIA
-
Ziniu Li
The Chinese University of Hong Kong, Shenzhen
-
Mingxuan Li
Columbia University
-
Yingru Li
The Chinese University of Hong Kong, Shenzhen
-
Yuchen Li
Carnegie Mellon University
-
Zexiang Liu
Control Systems
-
Bo Liu
the university of texas at austin
-
Kang Liu
University of Michigan
-
Xiuyuan Lucy Lu
Deep Mind
-
Siva Theja Maguluri
Georgia Institute of Technology
-
Gaurav Mahajan
University of California San Diego
-
Brendan Mallery
SUNY Albany
-
SAPTARSHI MANDAL
University of illinois, Urbana-Champaign
-
Weichao Mao
University of Illinois Urbana Champaign
-
Nikolai Matni
University of Pennsylvania
-
Dylan Miller
Heron Systems
-
Prabhat Kumar Mishra
University of Illinois at Urbana Champaign
-
Edward Mitchell
University of Tennessee- Knoxville
-
Muhammad Mobin
Trafix LLC
-
Aditya Modi
University of Michigan
-
Avi Mohan
Boston university
-
Mehrdad Moharrami
University of Illinois at Urbana Champaign
-
Aryan Mokhtari
University of Texas at Austin
-
Andrea Montanari
Stanford University
-
Jose Morales E.
UTSA
-
Robert Mueller
Technical University of Munich
-
Sayak Mukherjee
Pacific Northwest National Laboratory
-
Yashaswini Murthy
University of Illinois at Urbana-Champaign
-
Dheeraj Narasimha
Texas A & M University
-
Linda Ness
Rutgers University
-
Khai Nguyen
Hanoi University of Science and Technology
-
Aldo Pacchiano
UC Berkeley
-
Pavan Padmashali
SomaDetect Inc
-
Advait Parulekar
University of Texas at Austin
-
Gandharv Patil
McGill University/Mila
-
Anay Pattanaik
University of Illinois at Urbana Champaign
-
Majela Pentón Machado
University Federal of Bahia
-
Jian Qian
MIT
-
Chao Qin
Columbia University
-
Guannan Qu
Carnegie Mellon University
-
Kemmannu Vineet Venkatesh Rao
University of Michigan Ann-Arbor
-
Desik Rengarajan
Texas A&M University
-
Mardavij Roozbehani
Mass Institute of Tech
-
Arghyadip Roy
University of Illinois at Urbana-Champaign
-
Venkatesh Saligrama
Boston University
-
Akanksha Saran
UT Austin
-
Siddhartha Satpathi
UIUC
-
Jordan Schneider
University of Texas at Austin
-
Devavrat Shah
Massachusetts Institute of Technology
-
Vijay Shah
George Mason University
-
Sanjay Shakkottai
University of Texas Austin
-
Nihal Sharma
The University of Texas at Austin
-
Qin Sheng
Baylor University
-
Shanu Shwetank
CognitiveScale ltd
-
Hussein Sibai
University of Illinois at Urbana-Champaign
-
Karan Singh
Microsoft Research
-
Aarti Singh
Carnegie Mellon University
-
PIYUSH SINGH
University of Michigan Ann Arbor
-
Yuda Song
Carnegie Mellon University
-
Lin Song
UIUC
-
R. Srikant
University of Illinois at Urbana-Champaign
-
Varsha Srivastava
Quantum Integrators Group LLC
-
Vijay Subramanian
University of Michigan
-
Shunqiao Sun
The University of Alabama
-
Matus Telgarsky
University of Illinois Urbana-Champaign
-
Guy Tennenholtz
Technion
-
Saket Tiwari
Brown University
-
Matthew Trang
Virginia Tech
-
Caroline Uhler
MIT
-
Benjamin Van Roy
Stanford University
-
Sumanth Varambally
Indian Institute of Technology, Delhi
-
Sharan Vaswani
University of Alberta
-
Roberto Velho
Federal University of Rio Grande do Sul
-
Raj Kiriti Velicheti
UIUC
-
Richa Verma
IIT Madras
-
Francisco Verón Ferreira
Brandeis University
-
Daniel Vial
University of Texas at Austin
-
Pedro Vilanova Guerra
Stevens Institute of Technology
-
Trong-Linh Vu
Clarkson University
-
Haozhu Wang
University of Michigan
-
Mengdi Wang
Princeton
-
Weina Wang
Carnegie Mellon University
-
Zhaoran Wang
Northwestern University
-
Zizhao Wang
The university of Texas at Austin
-
Xiaojing Wang
University of Connecticut
-
Xupeng Wei
University of Michigan
-
Tomer Weiss
New Jersey Institute of Technology
-
Roy Welsch
M.I.T.
-
Joab Winkler
Sheffield University
-
Anna Winnicki
UIUC
-
Peng WU
Institute of Software, Chinese Academy of Sciences
-
XIAOYU XIE
Brown University
-
Tian Xu
Nanjing University
-
Masanao Yajima
Boston Universisty
-
Zixian Yang
University of Michigan
-
Yun Yang
UIUC
-
Erdal Yilmaz
Analog Devices Inc.
-
Ming Yin
University of California, Santa Barbara
-
Hwei-Jang Yo
National Cheng-Kung University
-
Haiyan Yu
Penn State University
-
Muhammad Aneeq uz Zaman
University of Illinois Urbana-Champaign
-
Yulin Zhang
UT Austin
-
ZIYI ZHANG
University of Michigan
-
Xuezhou Zhang
Princeton
-
Yili Zhang
University of Michigan
-
Kaiqing Zhang
MIT
-
Yi Zhang
The University of Texas at Austin
-
Jian-Zhou Zhang
Sichuan University
-
Xiaoning Zheng
Jinan University
-
Xueyu Zhu
University of Iowa
-
Martin Zubeldia
Georgia Institute of Technology
Workshop Schedule
Monday, August 2, 2021
-
10:00 - 10:45 am EDTGathertown Morning CoffeeCoffee Break - Virtual
-
10:45 - 11:00 am EDTWelcomeVirtual
- Brendan Hassett, ICERM/Brown University
-
11:00 - 11:30 am EDTA Boosting Approach to Reinforcement LearningVirtual
- Speaker
- Elad Hazan, Princeton University
- Session Chair
- Aditya Gopalan, Indian Institute of Science (Virtual)
Abstract
We will describe an algorithmic approach for learning in large Markov decision processes whose complexity is independent of the number of states. This task is in general computationally hard. We will present a boosting-inspired methodology that gives rise to provably efficient methods under certain weak learning conditions. No background in boosting or reinforcement learning is required for the talk.
-
11:45 am - 12:15 pm EDTReinforcement Learning in High Dimensional Systems (and why "reward" is not enough...)Virtual
- Speaker
- Sham Kakade, University of Washington
- Session Chair
- Aditya Gopalan, Indian Institute of Science (Virtual)
Abstract
A fundamental question in the theory of reinforcement learning is what properties govern our ability to generalize and avoid the curse of dimensionality. With regards to supervised learning, these questions are well understood theoretically, and, practically speaking, we have overwhelming evidence on the value of representational learning (say through modern deep networks) as a means for sample efficient learning. Providing an analogous theory for reinforcement learning is far more challenging, where even characterizing the representational conditions which support sample efficient generalization is far less well understood. This talk will highlight recent advances towards characterizing when generalization is possible in reinforcement learning, focusing on both lower bounds (addressing issues of what constitutes a good representation) along with upper bounds (where we consider a broad set of sufficient conditions).
-
12:30 - 1:30 pm EDTLunch/Free TimeVirtual
-
1:30 - 2:00 pm EDTTowards a Theory of Representation Learning for Reinforcement LearningVirtual
- Speaker
- Alekh Agarwal, Microsoft
- Session Chair
- Murat Kocaoglu, Purdue University (Virtual)
Abstract
Provably sample-efficient reinforcement learning from rich observational inputs remains a key open challenge in research. While impressive recent advances have allowed the use of linear modelling while carrying out sample-efficient exploration and learning, the handling of more general non-linear models remains limited. In this talk, we study reinforcement learning using linear models, where the features underlying the linear model are learned, rather than apriori specified. While the broader question of representation learning for useful embeddings of complex data has seen tremendous progress, doing so in reinforcement learning presents additional challenges: good representations cannot be discovered without adequate exploration, but effective exploration is challenging in the absence of good representations. Concretely, we study this question in the context of low-rank MDPs [Jiang et al., 2017, Jin et al., 2019, Yang and Wang, 2019], where the features underlying a state-action pair are not assumed to be known, unlike most prior works. We develop two styles of methods, model-based and model-free. For the model-based method, we learn an approximate factorization of the transition model, plan within the model to obtain a fresh exploratory policy and then update our factorization with additional data. In the model-free technique, we learn features so that quantities such as value functions at subsequent states can be predicted linearly in those features. In both approaches, we address the intricate coupling between exploration and representation learning, and provide sample complexity guarantees. More details can be found at https://arxiv.org/abs/2006.10814 and https://arxiv.org/abs/2102.07035. [Based on joint work with Jingling Chen, Nan Jiang, Sham Kakade, Akshay Krishnamurthy, Aditya Modi and Wen Sun]
-
2:15 - 2:45 pm EDTFrank-Wolfe Methods in Probability SpaceVirtual
- Speaker
- Jose Blanchet, Stanford University
- Session Chair
- Murat Kocaoglu, Purdue University (Virtual)
Abstract
We study the problem of minimizing a smooth convex functional of a probability measure. This formulation can be used to encompass a wide range of problems and algorithms of interest in diverse areas such as reinforcement learning, variational inference, deconvolution and adversarial training. We introduce and study a class of Frank-Wolfe algorithms for solving this problem together with associated convergence guarantees which match finite dimensional optimization results. We illustrate our results in the context of Wasserstein barycenter relaxations with unconstrained support, optimal deconvolution, among others.
-
3:00 - 3:30 pm EDTBreakCoffee Break - Virtual
-
3:30 - 4:00 pm EDTPreference based RL with finite time guaranteesVirtual
- Speaker
- Aarti Singh, Carnegie Mellon University
- Session Chair
- Thinh Doan, Virginia Tech (Virtual)
Abstract
As reinforcement learning is used for solving increasingly complex problems, eliciting meaningful labels and rewards for supervision is becomes challenging. Preferences in the form of pairwise comparisons have emerged as an alternate feedback mechanism that are often easier to elicit and more accurate. Despite promising results in applications, the theoretical understanding of preference based RL is still in its infancy. This talk will outline our efforts in understanding the fundamental limits of learning when given access to both preferences and labels, algorithms that achieve those limits and some open questions.
-
4:15 - 4:45 pm EDTMinimum complexity interpolation in random features modelsVirtual
- Speaker
- Andrea Montanari, Stanford University
- Session Chair
- Thinh Doan, Virginia Tech (Virtual)
Abstract
Despite their many appealing properties, kernel methods are heavily affected by the curse of dimensionality. For instance, in the case of inner product kernels in ℝd, the Reproducing Kernel Hilbert Space (RKHS) norm is often very large for functions that depend strongly on a small subset of directions (ridge functions). Correspondingly, such functions are difficult to learn using kernel methods. This observation has motivated the study of generalizations of kernel methods, whereby the RKHS norm -- which is equivalent to a weighted ℓ2 norm -- is replaced by a weighted functional ℓp norm, which we refer to as p norm. Unfortunately, tractability of these approaches is unclear. The kernel trick is not available and minimizing these norms requires to solve an infinite-dimensional convex problem. We study random features approximations to these norms and show that, for p>1, the number of random features required to approximate the original learning problem is upper bounded by a polynomial in the sample size. Hence, learning with p norms is tractable in these cases. We introduce a proof technique based on uniform concentration in the dual, which can be of broader interest in the study of overparametrized models
-
4:45 - 5:30 pm EDTGathertown ReceptionReception - Virtual
Tuesday, August 3, 2021
-
10:30 - 11:00 am EDTGathertown Morning CoffeeCoffee Break - Virtual
-
11:00 - 11:30 am EDTPlanning and Learning from InterventionsVirtual
- Speaker
- Caroline Uhler, MIT
- Session Chair
- Botao Hao, Deepmind (Virtual)
Abstract
Massive data collection holds the promise of a better understanding of complex phenomena and ultimately, of better decisions. An exciting opportunity in this regard stems from the growing availability of perturbation / intervention data (manufacturing, advertisement, education, genomics, etc.). In order to obtain mechanistic insights from such data, a major challenge is the development of a framework that integrates observational and interventional data. I will present such a causal framework and discuss how it allows predicting the effect of yet unseen interventions and identifying the optimal interventions to perform.
-
11:45 am - 12:15 pm EDTBatch Value Function TournamentVirtual
- Speaker
- Nan Jiang, University of Illinois Urbana-Champaign
- Session Chair
- Botao Hao, Deepmind (Virtual)
Abstract
Offline RL has attracted significant attention from the community as it offers the possibility of applying RL when active data collection is difficult. A key missing ingredient, however, is a reliable model-selection procedure that enables hyperparameter tuning, and reduction to off-policy evaluation either suffer exponential variance or relies on additional hyperparameters, creating a chicken-and-egg problem. In this talk I will discuss our recent progress on a version of this problem, where we need to identify Q* from a large set of candidate functions using a polynomial-sized exploratory dataset. The question is also a long-standing open problem about the information-theoretic nature of batch RL, and many suspected that the task is simply impossible. In our recent work, we provide a solution to this seemingly impossible task via (1) a tournament procedure that performs pairwise comparisons, and (2) a clever trick that partitions the large state-action space adaptively according to the compared functions. The resulting algorithm, BVFT, is very simple and can be readily applied to cross validation, with preliminary empirical results showing promising performance.
-
12:30 - 1:30 pm EDTLunch/Free TimeVirtual
-
1:30 - 2:00 pm EDTA Lyapunov approach for finite-sample convergence bounds with off-policy RLVirtual
- Speaker
- Sanjay Shakkottai, University of Texas Austin
- Session Chair
- Shuo Han, University of Illinois at Chicago (Virtual)
Abstract
In this talk, we derive finite-sample bounds for Markovian Stochastic Approximation, using the generalized Moreau envelope as a Lyapunov function. This result, we show, enables us to derive finite-sample bounds for a large class of value-based asynchronous reinforcement learning (RL) algorithms. Specifically, we show finite-sample mean-square convergence bounds for asynchronous RL algorithms such as Q-learning, n-step TD, TD(lambda), and off-policy TD algorithms including V-trace. As a by-product, by analyzing the convergence bounds of n-step TD and TD(lambda), we provide theoretical insights into the bias-variance trade-off, i.e., efficiency of bootstrapping in RL. Based on joint work with Zaiwei Chen, Siva Theja Maguluri and Karthikeyan Shanmugam.
-
2:15 - 2:45 pm EDTReinforcement learning with factorizationVirtual
- Speaker
- Devavrat Shah, Massachusetts Institute of Technology
- Session Chair
- Shuo Han, University of Illinois at Chicago (Virtual)
Abstract
In the setup of a single-agent reinforcement learning viewed through the framework of Markov Decision Process, typically there are two primary challenges: (a) given access to the model or simulator), learning a good or optimal policy, and (b) identifying the model, using limited observed data potentially generated under sub-optimal and unknown policy.
Like the singular value decomposition of a matrix, the spectral decomposition or factorization of a “nice” multi-variate function suggests that it can be represented as a finite or countably infinite sum of product of functions of individual variables. In this talk, we shall discuss how factorization of Q-function can help design sample efficient learning with access to model simulator, and how the factorization of transition kernel can help learn the model from a single trajectory per agent in the setting of offline reinforcement learning with heterogenous agents.
This is based on joint works:
Q-function learning: https://arxiv.org/abs/2006.06135 Offline personalized model learning: https://arxiv.org/abs/2102.06961 " -
3:00 - 3:30 pm EDTBreakCoffee Break - Virtual
-
3:30 - 4:00 pm EDTAlgorithms for metric elicitation via the geometry of classifier statistics.Virtual
- Speaker
- Sanmi Koyejo, University of Illinois at Urbana-Champaign
- Session Chair
- Jason Lee, Princeton (Virtual)
Abstract
Selecting a suitable metric for real-world machine learning applications remains an open problem, as default metrics such as classification accuracy often do not capture tradeoffs relevant to the downstream decision-making. Unfortunately, there is limited formal guidance in the machine learning literature on how to select appropriate metrics. We are developing formal interactive strategies by which a practitioner may discover which metric to optimize, such that it recovers user or expert preferences. I will outline our current work on metric elicitation, including some open problems.
-
4:15 - 4:45 pm EDTOn The Convergence Rate Of Entropy-regularized Natural Policy Gradient With Linear FunctionVirtual
- Speaker
- R. Srikant, University of Illinois at Urbana-Champaign
- Session Chair
- Jason Lee, Princeton (Virtual)
Abstract
We study the convergence rate of entropy-regularized Natural Policy Gradient (NPG) algorithms with linear function approximation. We show that NPG exhibits O(1/T) within an approximation error under mild assumptions on the distribution mismatch and the representation power of the feature vectors, and linear convergence under stronger assumptions. Joint work with Semih Cayci and Niao He.
-
4:45 - 5:30 pm EDTGathertown ReceptionReception - Virtual
Wednesday, August 4, 2021
-
10:30 - 11:00 am EDTGathertown Morning CoffeeCoffee Break - Virtual
-
11:00 - 11:30 am EDTOn the effectiveness of nonconvex policy optimizationVirtual
- Speaker
- Yuxin Chen, Princeton University
- Session Chair
- Vijay Subramanian, University of Michigan (Virtual)
Abstract
Recent years have witnessed a flurry of activity in solving reinforcement learning problems via nonconvex optimization. While conventional wisdom often takes a dim view of nonconvex optimization algorithms due to their susceptibility to spurious local minima, simple first-order optimization methods have been remarkably successful in practice. The theoretical footings, however, had been largely lacking until recently. In this talk, we make progress towards understanding the efficacy of policy gradient type algorithms with softmax parameterization --- a family of nonconvex policy optimization algorithms widely used in modern reinforcement learning. On the one hand, we demonstrate that softmax policy gradient methods can take (super)-exponential time to converge, even in the presence of a benign initialization and an initial state distribution amenable to optimization. On the other hand, we show that employing natural policy gradients and enforcing entropy regularization allow for nearly dimension-free global linear convergence.
-
11:45 am - 12:15 pm EDTThe dual of the margin in classification problems.Virtual
- Speaker
- Matus Telgarsky, University of Illinois Urbana-Champaign
- Session Chair
- Vijay Subramanian, University of Michigan (Virtual)
Abstract
Softmax mappings appear in many places in RL: e.g., to choose actions, as with any softmax policy, or even to weight both states and actions, as in the O-REPS method for adversarial RL settings. The purpose of this talk is to survey the effectiveness of a related softmax that arises outside of RL, namely in classification problems: certain dual variables are obtained via a softmax mapping, and analyzing this primal-dual connection leads both to improved analyses of existing algorithms, and to new algorithms. Concretely, this perspective leads to 1/t and 1/t^2 optimization rates for the (nonsmooth) margin objective of linear predictors (despite the 1/sqrt{t} lower bound for general nonsmooth problems), and to a general abstract convergence guarantee for homogeneous deep networks which is outside the NTK and other local analyses. Joint work with Ziwei Ji and Nati Srebro.
-
12:30 - 1:30 pm EDTLunch/Free TimeVirtual
-
1:30 - 2:00 pm EDTIs Pessimism Provably Efficient for Offline RL?Virtual
- Speaker
- Zhaoran Wang, Northwestern University
- Session Chair
- Siva Theja Maguluri, Georgia Institute of Technology (Virtual)
Abstract
We study offline reinforcement learning (RL), which aims to learn an optimal policy based on a dataset collected a priori. Due to the lack of further interactions with the environment, offline RL suffers from the insufficient coverage of the dataset, which eludes most existing theoretical analysis. In this paper, we propose a pessimistic variant of the value iteration algorithm (PEVI), which incorporates an uncertainty quantifier as the penalty function. Such a penalty function simply flips the sign of the bonus function for promoting exploration in online RL, which makes it easily implementable and compatible with general function approximations.
Without assuming the sufficient coverage of the dataset, we establish a data-dependent upper bound on the suboptimality of PEVI for general Markov decision processes (MDPs). When specialized to linear MDPs, it matches the information-theoretic lower bound up to multiplicative factors of the dimension and horizon. In other words, pessimism is not only provably efficient but also minimax optimal. In particular, given the dataset, the learned policy serves as the ""best effort"" among all policies, as no other policies can do better. Our theoretical analysis identifies the critical role of pessimism in eliminating a notion of spurious correlation, which emerges from the ""irrelevant"" trajectories that are less covered by the dataset and not informative for the optimal policy. -
2:15 - 2:45 pm EDTReinforcement Learning, Bit by BitVirtual
- Speaker
- Xiuyuan Lucy Lu, Deep Mind
- Session Chair
- Siva Theja Maguluri, Georgia Institute of Technology (Virtual)
Abstract
Data efficiency poses an impediment to carrying the success of reinforcement learning agents over from simulated to real environments. The design of data-efficient agents calls for a deeper understanding of information acquisition and representation. I will discuss concepts and a regret bound that together offer principled guidance. The bound sheds light on questions of what information to seek, how to seek that information, and what information to retain. To illustrate concepts, I will also share results generated by simple agents that build on them.
-
3:00 - 3:30 pm EDTBreakCoffee Break - Virtual
-
3:30 - 4:00 pm EDTCareful PessimismVirtual
- Speaker
- Emma Brunskill, Stanford University
- Session Chair
- Rajiv Khanna, UC Berkeley (Virtual)
Abstract
Pessimism with respect to quantified uncertainty has received significant recent interest in offline batch RL methods. I’ll present some of our recent work in this direction such as model free and policy search offline RL.
-
4:15 - 4:45 pm EDTOptimization for over-parameterized systems: from convexity to PL* conditionVirtual
- Speaker
- Mikhail Belkin, UCSD
- Session Chair
- Rajiv Khanna, UC Berkeley (Virtual)
Abstract
The success of deep learning is due, to a large extent, to the remarkable effectiveness of gradient-based optimization methods applied to large neural networks. In this talk I will discuss some general mathematical principles allowing for efficient optimization in over-parameterized non-linear systems, a setting that includes deep neural networks. I will discuss that optimization problems corresponding to these systems are not convex, even locally, but instead satisfy the Polyak-Lojasiewicz (PL) condition on most of the parameter space, allowing for efficient optimization by gradient descent or SGD. We connect the PL condition of these systems to the condition number associated to the tangent kernel and show how a non-linear theory for those systems parallels classical analyses of over-parameterized linear equations. In a related but conceptually separate development, I will discuss a new perspective on the remarkable recently discovered phenomenon of transition to linearity (constancy of NTK) in certain classes of large neural networks. I will show how this transition to linearity results from the scaling of the Hessian with the size of the network. Combining these ideas, I will show how the transition to linearity can be used to demonstrate the PL condition and convergence for a large class of wide neural networks. Joint work with Chaoyue Liu and Libin Zhu
-
4:45 - 5:00 pm EDTClosing RemarksVirtual
All event times are listed in ICERM local time in Providence, RI (Eastern Daylight Time / UTC-4).
All event times are listed in .
ICERM local time in Providence, RI is Eastern Daylight Time (UTC-4). Would you like to switch back to ICERM time or choose a different custom timezone?