Organizing Committee
- Issa Dahabreh
Harvard University - Jon Steingrimsson
Brown University - Elizabeth Stuart
Johns Hopkins Bloomberg School of Public Health
Abstract
Estimators of various causal or statistical quantities are usually constructed with a particular target population in mind, that is, the population about which the investigators intend to draw inferences (e.g., decide on the implementation of a treatment strategy or use algorithm-derived predictions). Typically, however, the data used for estimation comes from a population that differs from the target population. How to ensure or evaluate whether the estimates generalize to the target population is a question that has received substantial attention in many scientific disciplines, but with the fields not always connecting with one another on overlapping challenges and solutions. This workshop will bring together experts from different disciplines to present state-of-the-science methods to address generalizability and discuss key challenges, and open problems.
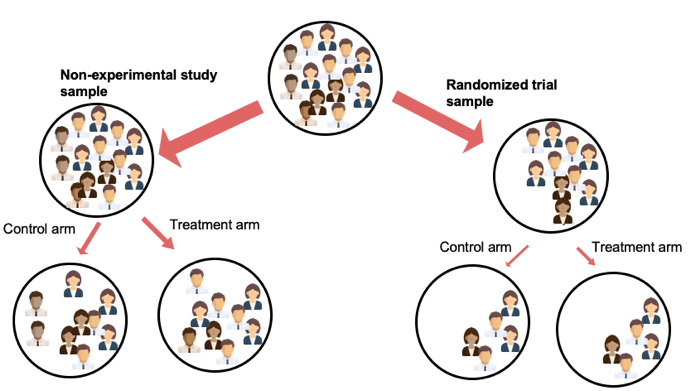
Confirmed Speakers & Participants
Talks will be presented virtually or in-person as indicated in the schedule below.
- Speaker
- Poster Presenter
- Attendee
- Virtual Attendee
-
Abdullah Abdelaziz
UIC
-
Mahesh Agarwal
University of Michigan-Dearborn
-
Azza Ahmed
TU Delft
-
Rubiya Akter
McGill University
-
Daniel Antiporta
Johns Hopkins University
-
Amir Asiaee
Vanderbilt University Medical Center
-
Monica Aswani
University of Alabama at Birmingham
-
Nir Aviv
Tel Aviv University
-
Luis Azevedo
Faculty of Medicine, University of Porto
-
Yihan Bao
Yale University
-
David Barker
Alpert Medical School of Brown University
-
Sungho Bea
Sungkyunkwan University
-
Nrupen Bhavsar
Duke University School of Medicine
-
Turki Bin Hammad
Saudi Food and Drug Authority
-
Ahmed Boughdiri
INRIA
-
Jeremy Brown
Harvard University
-
Ashley Buchanan
University of Rhode Island
-
Maddalena Centanni
Uppsala University
-
Amy Chang
University of Sheffield
-
Arthur Chatton
Université de Montréal
-
Guanhua Chen
University of Wisconsin-Madison
-
Hongmei Chi
Florida A&M University
-
Felicia Chi
Kaiser Permanente Northern California Division of Research
-
Yu-Han Chiu
Penn State College of Medicine
-
Oscar Clivio
University of Oxford
-
Issa Dahabreh
Harvard University
-
Elena Dal Torrione
University of Rome Tor Vergata
-
Biswa Datta
Brainware University, Kolkata-700125
-
Philip Dawid
University of Cambridge
-
Irina Degtiar
Mathematica Policy Research
-
Allison DeLong
Brown University School of Public Health
-
Michael Denly
Texas A&M University
-
Ivan Diaz
NYU
-
Elizabeth Eisenhauer
Westat
-
Michael Elliott
University of Michigan School
-
Ana Lucia Espinosa Dice
Harvard TH Chan School of Public Health
-
Fei Fang
Yale University
-
Timothy Feeney
UNC Chapel Hill
-
Noam Finkelstein
Unaffiliated
-
Laura Forastiere
Yale University
-
Omar Galarraga
Brown University School of Public Health
-
Jason Gantenberg
Brown University
-
Ilana Gareen
Brown University
-
Constantine Gatsonis
Brown University School of Public Health
-
Milena Gianfrancesco
University of California, San Francisco
-
Mauro Giuffrè
Yale School of Medicine
-
Larry Han
Northeastern University
-
Harrison Hansford
UNSW
-
Mohammad Sazzad Hasan
McGill University
-
Eleanor Hayes-Larson
UCLA
-
William Hoffmann
Northwestern University
-
Tiffany Hsieh
Brown University
-
Chen Hu
Johns Hopkins
-
Yi Huang
University of Maryland, Baltimore County
-
Andrew Huang
Brown University
-
Melody Huang
Harvard University
-
Ta-Wei Huang
Harvard Business School
-
Jared Huling
University of Minnesota
-
Ajmery Jaman
Mcgill University
-
Bohang Jiang
Massachusetts General Hospital
-
Eloise Kaizar
Ohio State University
-
Rickard Karlsson
Delft University of Technology
-
Hussein Khalil
Purdue university
-
Anthony Kityo
Kangwon National University
-
May Frauke Kreuter
University of Maryland
-
Hüseyin Küçükali
Queen's University Belfast
-
Sandi Kwee
Univeristy of hawaii
-
Nguyen Le
University of Texas at Austin
-
Tae Yoon Lee
University of British Columbja
-
Chanhwa Lee
University of North Carolina at Chapel Hill
-
Ignacio Leiva
Universitätsklinikum Heidelberg
-
Yi Li
McGill University
-
Fan Li
Yale School of Public Health
-
Mavis Liang
Brown University School of Public Health
-
Yuanfei Liu
Cambridge
-
Bolun Liu
Johns Hopkins University
-
Chunnan Liu
Brown university
-
Fangyu Liu
Johns Hopkins Bloomberg School of Public Health
-
Jun Lu
University of Illinois Chicago
-
Ivana Malenica
Harvard University
-
Emma McGee
Harvard University
-
Vishwali Mhasawade
New York University
-
Shilpi Misra
Johns Hopkins University
-
Hal Morgenstern
University of Michigan
-
Philani Mpofu
Flatiron Health
-
Daniel Nevo
Tel Aviv University
-
Yunha Noh
McGill University
-
Sharon-Lise Normand
Harvard Medical School
-
In-Sun Oh
McGill University
-
Rohit Ojha
JPS Health Network
-
Camila Olarte Parra
Karolinska Institutet
-
OLUWADAMILOLA OLAYEMI
MEDIBETH GLOBAL HEALTH CENTRE, NIGERIA
-
Caglar Onal
Northwestern University
-
Itsuki Osawa
Columbia University
-
Qing Pan
George Washington University
-
George Papandonatos
Brown University
-
Harsh Parikh
Johns Hopkins Bloomberg School of Public Health
-
Jonas Peters
ETH Zurich
-
Michelle Qin
Johns Hopkins University
-
Sophia Rein
Harvard University
-
Haoyu Ren
University of Maryland, Baltimore County
-
Anke Richters
The Netherlands Comprehensive Cancer Organisation
-
Ado Rivera
Kaiser Permanente Southern California Department of Research and Evaluation
-
Sarah Robertson
Harvard University
-
James Rogers
Metrum Research Group
-
Rachael Ross
Columbia University
-
Kara Rudolph
Columbia University
-
Rienna Russo
Harvard University
-
Cyrus Samii
New York University
-
Mariia Samoilenko
Université de Montréal
-
Amit Sasson
Bell Statistics
-
Christopher Schmid
Brown University
-
Bonnie Shook-Sa
University of North Carolina at Chapel Hill
-
Louisa Smith
Northeastern University
-
Boris Sobolev
University of British Columbia
-
Yang Song
BIDMC
-
Lei Song
George Washington University
-
Jon Steingrimsson
Brown University
-
Florian Stijven
KU Leuven
-
Elizabeth Stuart
Johns Hopkins Bloomberg School of Public Health
-
Kenneth Taylor
Komodo Health
-
Elizabeth Tipton
Northwestern University
-
Khaled Toffaha
Khalifa University
-
Iris Tong
Stanford University
-
Thomas Trikalinos
Brown University
-
Bang Truong
AbbVie Inc
-
Erica Twardzik
Johns Hopkins University
-
Lawson Ung
Harvard T. H. Chan School of Public Health
-
Ruoyu Wang
HSPH
-
Guanbo Wang
Harvard University
-
Ming-Jer Wang
Richard J. Daley College
-
Xuerong Wen
University of Rhode Island
-
Tian An Wong
University of Michigan-Dearborn
-
Zach Wood-Doughty
Northwestern University
-
Yingyan Wu
University of California, Los Angeles
-
Jay Xu
University of California, Los Angeles
-
Lei Yan
Yale University
-
Xingchi Yan
Harvard University
-
Shu Yang
Department of Statistics, North Carolina State University
-
Zenas Yiu
University of Manchester
-
Zhenghao Zeng
Carnegie Mellon University
-
Guoqiang Zhang
Karolinska Institutet
-
Yi Zhang
Harvard University
-
Jie Zhang
University
-
Yuping Zhang
University of Connecticut
-
Yuqing Zhang
Massachusetts General Hospital
-
Jiwei Zhao
University of Wisconsin-Madison
-
Yuan Zhao
New York Univeristy
-
Yi Zhao
Tufts University
-
Xin Zhou
Yale School of Public Health
-
Baijun Zhou
Massachusetts General Hospital
-
Paul Zivich
University of North Carolina at Chapel Hill
-
Andrew Zullo
Brown University
Workshop Schedule
Friday, November 17, 2023
-
8:30 - 8:50 am ESTCheck In11th Floor Collaborative Space
-
8:50 - 9:00 am ESTICERM WelcomeWelcome - 11th Floor Lecture Hall
- Brendan Hassett, ICERM/Brown University
-
9:00 - 9:15 am ESTOrganizer WelcomeWelcome - 11th Floor Lecture Hall
- Issa Dahabreh, Harvard University
- Jon Steingrimsson, Brown University
- Elizabeth Stuart, Johns Hopkins Bloomberg School of Public Health
-
9:15 - 9:45 am ESTEvaluating Ex Ante Counterfactual Predictions Using Ex Post Causal Inference11th Floor Lecture Hall
- Virtual Speaker
- Cyrus Samii, New York University
- Session Chair
- Jon Steingrimsson, Brown University
Abstract
We derive a formal, decision-based method for comparing the performance of counterfactual treatment regime predictions using the results of experiments that give relevant information on the distribution of treated outcomes. Our approach allows us to quantify and assess the statistical significance of differential performance for optimal treatment regimes estimated from structural models, extrapolated treatment effects, expert opinion, and other methods. We apply our method to evaluate optimal treatment regimes for conditional cash transfer programs across countries where predictions are generated using data from experimental evaluations in other countries and pre-program data in the country of interest.
-
10:05 - 10:20 am ESTCoffee Break11th Floor Collaborative Space
-
10:20 - 10:50 am ESTEfficiently transporting average treatment effects using a sufficient subset of effect modifiers11th Floor Lecture Hall
- Speaker
- Kara Rudolph, Columbia University
- Session Chair
- Jon Steingrimsson, Brown University
Abstract
We develop flexible and nonparametric estimators of the average treatment effect (ATE) transported to a new population that offer potential efficiency gains by incorporating only a sufficient subset of effect modifiers that are differentially distributed between the source and target populations into the transport step. We develop both a one-step estimator when this sufficient subset of effect modifiers is known and a collaborative one-step estimator when it is unknown. We discuss when we would expect our estimators to be more efficient than those that assume all covariates may be relevant effect modifiers and the exceptions when we would expect worse efficiency. We use simulation to compare finite sample performance across our proposed estimators and existing estimators of the transported ATE, including in the presence of practical violations of the positivity assumption. Lastly, we apply our proposed estimators to a large-scale housing trial.
-
11:10 - 11:40 am ESTUnderstanding effect heterogeneity in observational and randomized studies of causality11th Floor Lecture Hall
- Speaker
- Ivan Diaz, NYU
- Session Chair
- Jon Steingrimsson, Brown University
-
12:00 - 12:05 pm ESTGroup Photo (Immediately After Talk)11th Floor Lecture Hall
-
12:05 - 1:30 pm ESTLunch/Free Time
-
1:30 - 2:00 pm ESTGeneralizing trial evidence to target populations in non-nested designs: Applications to AIDS clinical trials11th Floor Lecture Hall
- Speaker
- Ashley Buchanan, University of Rhode Island
- Session Chair
- Jon Steingrimsson, Brown University
Abstract
Comparative effectiveness evidence from randomized trials may not be directly generalizable to a target population of substantive interest when, as in most cases, trial participants are not randomly sampled from the target population. Motivated by the need to generalize evidence from two trials conducted in the AIDS Clinical Trials Group (ACTG), we consider weighting, regression, and doubly robust estimators to estimate the causal effects of HIV interventions in a specified population of people living with HIV in the USA. We focus on a non-nested trial design and discuss strategies for both point and variance estimation of the target population average treatment effect. Specifically in the generalizability context, we demonstrate both analytically and empirically that estimating the known propensity score in trials does not increase the variance for each of the weighting, regression, and doubly robust estimators. We apply these methods to generalize the average treatment effects from two ACTG trials to specified target populations and operationalize key practical considerations. Finally, we report on a simulation study that investigates the finite-sample operating characteristics of the generalizability estimators and their sandwich variance estimators.
-
2:20 - 2:50 pm ESTExtending Inferences to a Target Population Without Positivity11th Floor Lecture Hall
- Speaker
- Paul Zivich, University of North Carolina at Chapel Hill
- Session Chair
- Jon Steingrimsson, Brown University
Abstract
To draw inferences from a sample to the target population, where the sample is not a random sample of the target population, various generalizability and transportability methods can be considered. Many of these modern approaches rely on a structural positivity assumption, such that all relevant covariate patterns in the target population are also observed in the secondary population of which the data is random sample of. Strict eligibility criteria, particularly in the context of randomized trials, may lead to violations of this positivity assumption. To address this concern, common methods are to restrict the target population, restrict the adjustment set, or extrapolate from a statistical model. Instead of these approaches, which all have concerning limitations, we propose a synthesis, or combination, of statistical (e.g., g-methods) and mathematical (e.g., microsimulation, mechanistic) models. Briefly, a statistical model is fit for the regions of the parameter space where positivity holds, and a mathematical model is used to fill-in, or impute, the nonpositive regions. For estimation, we propose two augmented inverse probability weighting estimators; one based on estimating the parameters of a marginal structural model, and the other based on estimating the conditional average causal effect. The standard approaches and the proposed synthesis method are illustrated with a simulation study and an applied example on the effect of antiretroviral therapy on CD4 cell count. The proposed synthesis method sheds light on a way to address challenges associated with the positivity assumption for transporting and causal inference more generally.
-
3:10 - 3:40 pm ESTCoffee Break11th Floor Collaborative Space
-
3:40 - 4:00 pm ESTPostdoc Talk - Generalizing and transporting inferences about the effects of treatment assignment subject to non-adherence11th Floor Lecture Hall
- Speaker
- Sarah Robertson, Harvard University
- Session Chair
- Jon Steingrimsson, Brown University
Abstract
We describe causal estimands that can be of interest in transportability and generalizability analyses. We examine these estimands both under perfect and imperfect adherence to treatment assignment, and discuss the conditions under which the estimands are identifiable. We consider the common setting for such analyses where the trial data contain information on baseline covariates, assignment at baseline, a baseline intervention or point treatment, and outcomes measured at a fixed time of follow-up; and data from non- randomized individuals only contain information on baseline covariates. We review identification results under perfect adherence and study two examples in which non-adherence severely limits the ability to transport inferences about the effects of treatment assignment to the target population.
-
4:00 - 4:20 pm ESTPostdoc Talk - Who Are You Missing?: A Principled Approach to Characterizing the Underrepresented Population11th Floor Lecture Hall
- Speaker
- Harsh Parikh, Johns Hopkins Bloomberg School of Public Health
- Session Chair
- Jon Steingrimsson, Brown University
Abstract
Treatment effect estimates derived from Randomized Controlled Trials (RCTs) play a pivotal role in informing decision-making in healthcare and various other fields. However, the successful transportability of these estimates to the target population of interest hinges upon several underlying assumptions, such as positivity and external validity of the experimental study. When these assumptions are violated, the resulting target treatment effect estimates may suffer from inaccuracy and imprecision, potentially leading to suboptimal decision outcomes. We introduce a novel framework to identify subpopulations of the target populations for which the estimated target treatment effects may be inaccurate and/or imprecise, and approaches to characterize those subpopulations. This characterization may not only enhance the safety and robustness of decisions based on RCT data but also identifies underrepresented subpopulations within the target population. This knowledge can facilitate the design of more targeted and efficient subsequent trials, thereby optimizing the allocation of resources and improving the overall effectiveness of intervention strategies. Our approach offers a valuable contribution to the field of evidence-based decision-making by addressing the critical issue of assumption violations in treatment effect transportability.
-
4:20 - 4:40 pm ESTPostdoc Talk - Using external data to address measurement error: a transportability perspective11th Floor Lecture Hall
- Speaker
- Rachael Ross, Columbia University
- Session Chair
- Jon Steingrimsson, Brown University
Abstract
Extending inferences to a new target population is typically viewed as a task focused on external validity. However, we may need to extend inference of nuisance parameters between populations to account for systematic errors. For example, approaches to address measurement error rely on validation data to estimate measurement error parameters (e.g., sensitivity and specificity). Due to the difficulties and expenses of collecting validation data, studies may use data external to the main study (i.e., validation data that includes individuals outside of the sample in which we want to estimate our parameter of interest). When data come from different places or time periods, there may be systematic differences (i.e., differing distribution of covariates that modify the measurement error). From a transportability perspective, we need to extend inference of the measurement error parameters from the validation data to the main sample. Despite this, it is often overlooked that use of external validation data implicitly relies on transportability assumptions (i.e., exchangeability and positivity). In this work we focus on the use of validation data to address outcome misclassification, in which our estimands are the natural course risk and the causal risk difference in an external sample. We show how transportability of misclassification parameters can be visualized with causal diagrams and outline identification assumptions for use of external validation data. Finally, we introduce a parametric iterated outcome regression estimator. These methods are motivated by and illustrated in an application to estimate the risk of preterm birth and the effect of maternal HIV infection on preterm birth in Lusaka, Zambia. In the main study, preterm birth was measured by last menstrual period, which has known measurement error; external validation data with preterm birth measured by both last menstrual period and ultrasound are used to account for the misclassification.
-
5:00 - 6:30 pm ESTWelcome ReceptionReception - 11th Floor Collaborative Space
Saturday, November 18, 2023
-
9:45 - 10:15 am ESTMitigating Bias in Treatment Effect Estimation: Strategies for Utilizing External Controls in Randomized Trials11th Floor Lecture Hall
- Speaker
- Shu Yang, Department of Statistics, North Carolina State University
- Session Chair
- Elizabeth Stuart, Johns Hopkins Bloomberg School of Public Health
Abstract
In recent years, real-world external controls (ECs) have gained popularity to enhance the efficacy of randomized controlled trials (RCTs), particularly in scenarios involving rare diseases or situations where equitable randomization is unfeasible or unethical. However, the suitability of ECs compared to RCTs varies, necessitating cautious consideration before utilizing ECs to avoid introducing substantial bias into treatment effect estimation. A central challenge lies in the potential incongruity of outcomes between concurrent controls (CCs) and ECs, even after accounting for covariate disparities, often attributable to latent confounding variables. This talk delves into a range of methodologies designed to mitigate the unknown biases associated with ECs. These methodologies encompass pre-testing, bias function modeling, and selective borrowing, all framed within the context of semiparametric models. These proposed strategies collectively form an essential toolkit for practitioners aiming to incorporate ECs effectively, offering a comprehensive framework to navigate their integration.
-
10:35 - 10:50 am ESTCoffee Break11th Floor Collaborative Space
-
10:50 - 11:20 am ESTImproving Transportability of Randomized Clinical Trial Inference Using Robust Prediction Methods11th Floor Lecture Hall
- Speaker
- Michael Elliott, University of Michigan School
- Session Chair
- Elizabeth Stuart, Johns Hopkins Bloomberg School of Public Health
Abstract
Randomized trials have been the gold standard for assessing causal effects since their introduction by Fisher in the 1920s, since they can eliminate both observed and unobserved confounding. Estimates of causal effects at the population level from randomized controlled trials (RCTs) can still be biased if there are both effect modification and systematic differences between the trial sample and the ultimate population of inference with respect to these modifiers. Recent advances in the survey statistics literature to improve inference in nonprobability samples by using information from probability samples can provide an avenue for improving population causal inference in randomized controlled trials when relevant probability samples of the patient population are available. We propose extending these estimators using either inverse probability weighting (IWPT) or prediction that can accommodate unequal probability of selection in the “benchmark” or population, and use Bayesian additive regression trees (BART) for both IPTW and prediction estimation that do not require specification of functional form or interaction. We also consider how the assumption of ignorability may be assessed from observed data and propose a sensitivity analysis under the failure of this assumption.
-
11:40 am - 12:10 pm ESTUniversal adaptability11th Floor Lecture Hall
- Speaker
- May Frauke Kreuter, University of Maryland
- Session Chair
- Elizabeth Stuart, Johns Hopkins Bloomberg School of Public Health
Abstract
The gold-standard approaches for gleaning statistically valid conclusions from data involve random sampling from the population. Collecting properly randomized data, however, can be challenging, so modern statistical methods, including propensity score reweighting, aim to enable valid inferences when random sampling is not feasible. We put forth an approach for making inferences based on available data from a source population that may differ in composition in unknown ways from an eventual target population. Whereas propensity scoring requires a separate estimation procedure for each different target population, we show how to build a single estimator, based on source data alone, that allows for efficient and accurate estimates on any downstream target data. We demonstrate, theoretically and empirically, that our target-independent approach to inference, which we dub “universal adaptability,” is competitive with target-specific approaches that rely on propensity scoring. Our approach builds on a surprising connection between the problem of inferences in unspecified target populations and the multicalibration problem, studied in the burgeoning field of algorithmic fairness. We show how the multicalibration framework can be employed to yield valid inferences from a single source population across a diverse set of target populations.
-
12:30 - 2:00 pm ESTLunch/Free Time
-
2:00 - 2:20 pm ESTPostdoc Talk - Sensitivity Analysis for Generalizing Experimental Results11th Floor Lecture Hall
- Speaker
- Melody Huang, Harvard University
- Session Chair
- Elizabeth Stuart, Johns Hopkins Bloomberg School of Public Health
Abstract
Randomized controlled trials (RCT’s) allow researchers to estimate causal effects in an experimental sample with minimal identifying assumptions. However, to generalize or transport a causal effect from an RCT to a target population, researchers must adjust for a set of treatment effect moderators. In practice, it is impossible to know whether the set of moderators has been properly accounted for. In the following paper, I propose a two parameter sensitivity analysis for generalizing or transporting experimental results using weighted estimators. The contributions in the paper are three-fold. First, I show that the sensitivity parameters are scale-invariant and standardized, and introduce an estimation approach for researchers to simultaneously account for the bias in their estimates from omitting a moderator, as well as potential changes to their inference. Second, I propose several tools researchers can use to perform sensitivity analysis: (1) different numerical measures to summarize the uncertainty in an estimated effect to unobserved confounding; (2) graphical summary tools for researchers to visualize the sensitivity in their estimated effects, as the confounding strength of the omitted variable changes; and (3) a formal benchmarking approach for researchers to estimate potential sensitivity parameter values using existing data. Finally, I demonstrate that the proposed framework can be easily extended to the class of doubly robust, augmented weighted estimators. The sensitivity analysis framework is applied to a set of Jobs Training Program experiments.
-
2:30 - 3:00 pm ESTGroup discussionProblem Session - 11th Floor Conference Room
-
3:00 - 3:30 pm ESTCoffee Break11th Floor Collaborative Space
-
3:30 - 4:30 pm ESTPanel Discussion11th Floor Lecture Hall
- Panelists
- Issa Dahabreh, Harvard University
- Jon Steingrimsson, Brown University
- Elizabeth Stuart, Johns Hopkins Bloomberg School of Public Health
Sunday, November 19, 2023
-
9:15 - 9:45 am ESTBeyond Generalization: Designing Randomized Experiments to Predict Treatment Effects11th Floor Lecture Hall
- Speaker
- Elizabeth Tipton, Northwestern University
- Session Chair
- Issa Dahabreh, Harvard University
Abstract
Much of the focus of methods for generalizing treatment effects has focused on estimation and hypothesis testing regarding a target population average treatment effect (ATE). But generalization is only an issue if treatment effects vary - and if they vary, why not focus on predicting unit-specific treatment effects instead? In this paper, we consider when prediction may be feasible, with a focus on planning studies for such purposes. We consider both cases in which the sample is from the target population and when it is not, and we focus on the use of a parametric linear regression model for these predictions. Doing so results in closed form expressions of error that can be translated into design parameters for use in study design.
-
10:05 - 10:20 am ESTCoffee Break11th Floor Collaborative Space
-
10:20 - 10:50 am ESTExtending Inferences from a Diverse Collection of Trials11th Floor Lecture Hall
- Speaker
- Eloise Kaizar, Ohio State University
- Session Chair
- Issa Dahabreh, Harvard University
Abstract
Traditional meta-analyses of randomized trials are often thought of as the “gold standard” for scientific evidence. However, accurate and precise interpretation of results of such methods is questionable under many real data circumstances. Recent work in the literature (based on methods to extend single-study results to target populations) allows the extension of multiple studies to make meaningful causally-interpretable conclusions for a target population. By adjusting for differences between sample and target population covariate distributions, such an approach accounts for some study-to-study variability. However, many other sources of between-study variability likely remain. We look to apply the traditional random-effects approach to accounting for this “extra” between-study variability, and explore what features of between-study variability are important to capture. As implemented, our approach has some drawbacks versus proposed methods that do not model between-study variability. We discuss the particular case when covariate distributions vary across studies.
-
11:10 - 11:40 am ESTTransportability of Causal Effects in Principal Strata11th Floor Lecture Hall
- Speaker
- Jared Huling, University of Minnesota
- Session Chair
- Issa Dahabreh, Harvard University
Abstract
Randomized clinical trials (RCTs) are the gold standard for producing evidence of treatment effects with high internal validity. Trial results, however, often impact populations that differ from those who enrolled in the trial. Differences between the trial and so-called target population can limit the relevance of trial findings for the target population. Methods in the generalizability and transportability literature aim to produce a treatment effect estimate that applies to a target population of interest. However, in randomized trials, participant non-adherence to the study medication or intervention can dramatically alter the interpretation of transported treatment effect estimates. If non-adherence patterns are expected to differ between the trial participants and those in the target population, the transported effect may no longer reflect the underlying effect of the treatment in the target population. In this work, we develop methods to address these concerns using a principal stratification approach to define subsets of the target population with distinct latent compliance patterns. These subsets form the basis of a transportability problem that we approach using causal inference techniques: defining scientifically-relevant estimands, clarifying necessary identification assumptions, and specifying theory-based estimation and inference techniques. This work addresses some common limitations of RCT data and thus makes such data more useful to clinicians and patients. Our proposed framework can also handle transportation of effects in any principal strata and thus has applicability beyond dealing with non-adherence.
All event times are listed in ICERM local time in Providence, RI (Eastern Daylight Time / UTC-4).
All event times are listed in .
ICERM local time in Providence, RI is Eastern Daylight Time (UTC-4). Would you like to switch back to ICERM time or choose a different custom timezone?
Request Reimbursement
This section is for general purposes only and does not indicate that all attendees receive funding. Please refer to your personalized invitation to review your offer.
- ORCID iD
- As this program is funded by the National Science Foundation (NSF), ICERM is required to collect your ORCID iD if you are receiving funding to attend this program. Be sure to add your ORCID iD to your Cube profile as soon as possible to avoid delaying your reimbursement.
- Acceptable Costs
-
- 1 roundtrip between your home institute and ICERM
- Flights in economy class to either Providence airport (PVD) or Boston airport (BOS)
- Ground Transportation to and from airports and ICERM.
- Unacceptable Costs
-
- Flights on U.K. airlines
- Seats in economy plus, business class, or first class
- Change ticket fees of any kind
- Multi-use bus passes
- Meals or incidentals
- Advance Approval Required
-
- Personal car travel to ICERM from outside New England
- Multiple-destination plane ticket; does not include layovers to reach ICERM
- Arriving or departing from ICERM more than a day before or day after the program
- Multiple trips to ICERM
- Rental car to/from ICERM
- Arriving or departing from airport other than PVD/BOS or home institution's local airport
- 2 one-way plane tickets to create a roundtrip (often purchased from Expedia, Orbitz, etc.)
- Travel Maximum Contributions
-
- New England: $350
- Other contiguous US: $850
- Asia & Oceania: $2,000
- All other locations: $1,500
- Note these rates were updated in Spring 2023 and superseded any prior invitation rates. Any invitations without travel support will still not receive travel support.
- Reimbursement Requests
-
Request Reimbursement with Cube
Refer to the back of your ID badge for more information. Checklists are available at the front desk and in the Reimbursement section of Cube.
- Reimbursement Tips
-
- Scanned original receipts are required for all expenses
- Airfare receipt must show full itinerary and payment
- ICERM does not offer per diem or meal reimbursement
- Allowable mileage is reimbursed at prevailing IRS Business Rate and trip documented via pdf of Google Maps result
- Keep all documentation until you receive your reimbursement!
- Reimbursement Timing
-
6 - 8 weeks after all documentation is sent to ICERM. All reimbursement requests are reviewed by numerous central offices at Brown who may request additional documentation.
- Reimbursement Deadline
-
Submissions must be received within 30 days of ICERM departure to avoid applicable taxes. Submissions after thirty days will incur applicable taxes. No submissions are accepted more than six months after the program end.