Organizing Committee
- Michael Buice
Allen Institute - Carina Curto
The Pennsylvania State University - Brent Doiron
University of Chicago - Zachary Kilpatrick
University of Colorado Boulder - Konstantin Mischaikow
Rutgers University - Katie Morrison
University of Northern Colorado
Abstract
One of the fundamental questions in neuroscience is to understand how network connectivity shapes neural activity. Over the last 10 years, tremendous progress has been made in collecting neural activity and connectivity data, but theoretical advances have lagged behind. This workshop will focus on identifying mathematical challenges that arise in studying the dynamics of learning, memory, plasticity, decision-making, sequence generation, and central pattern generator circuits. Mathematical ideas and approaches from dynamical systems, statistical mechanics, linear algebra, graph theory, topology, and traditional areas of applied mathematics are all expected to play an important role.
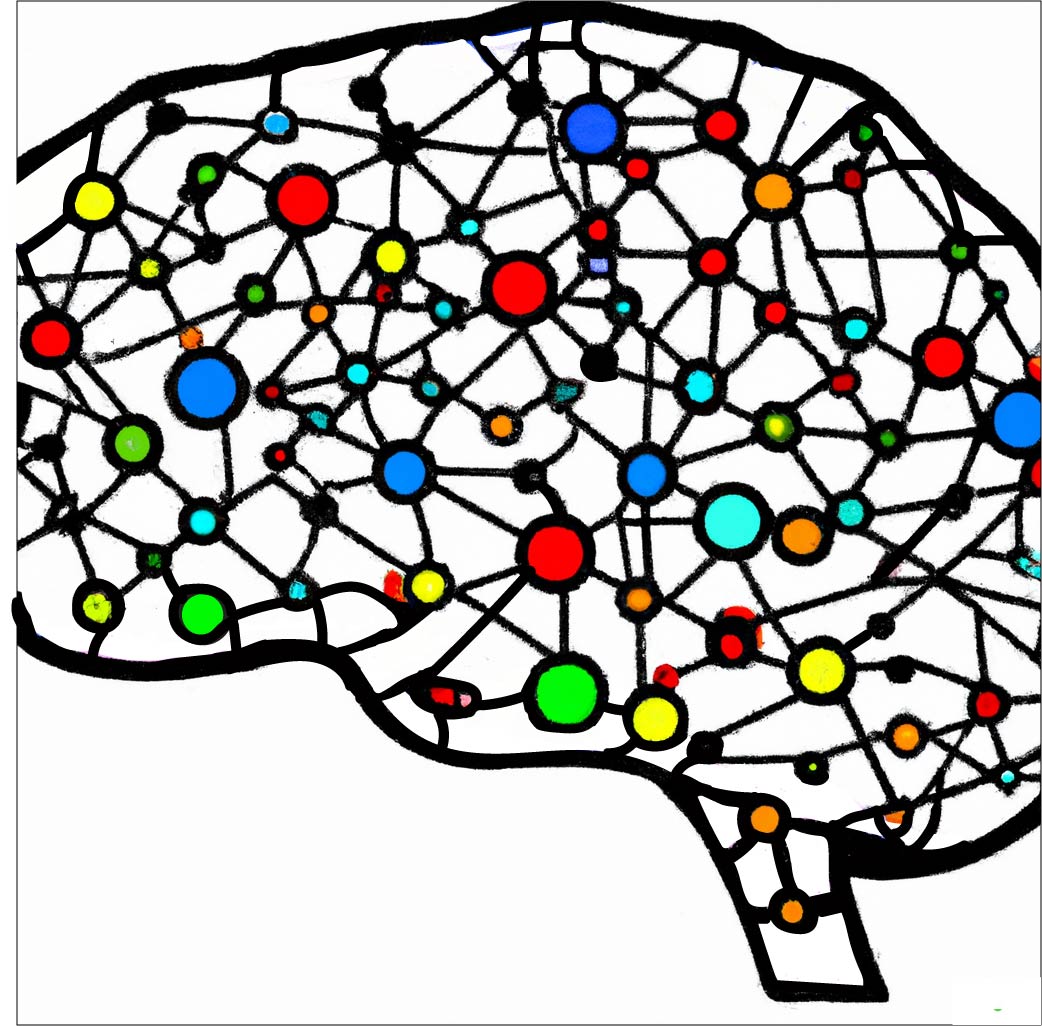
Confirmed Speakers & Participants
Talks will be presented virtually or in-person as indicated in the schedule below.
- Speaker
- Poster Presenter
- Attendee
- Virtual Attendee
-
Yashar Ahmadian
Cambridge University
-
Daniele Avitabile
Vrije Universiteit Amsterdam
-
Demba Ba
Harvard University
-
Andrea Barreiro
Southern Methodist University
-
Robin Belton
Smith College
-
Marcus Benna
UC San Diego
-
Prianka Bose
New Jersey Institute of Technology
-
Amitabha Bose
New Jersey Institute of Technology
-
Robyn Brooks
University of Utah
-
Peter Bubenik
University of Florida
-
Michael Buice
Allen Institute
-
Thomas Burns
ICERM
-
Carlos Castañeda Castro
Brown University
-
Rishidev Chaudhuri
University of California, Davis
-
Sehun Chun
Yonsei University
-
Heather Cihak
University of Colorado Boulder
-
Giovanna Citti
university of Bologna
-
Carina Curto
The Pennsylvania State University
-
Rodica Curtu
The University of Iowa
-
Steve Damelin
Mathematical Scientist, Ann Arbor MI
-
Anda Degeratu
University of Stuttgart
-
Darcy Diesburg
Brown University
-
Fatih Dinc
Stanford University
-
Brent Doiron
University of Chicago
-
Sean Escola
Columbia University
-
Matthew Farrell
Harvard University
-
Richard Foster
Virginia Commonwealth University
-
Michael Frank
Brown University
-
Marcio Gameiro
Rutgers University
-
Tomas Gedeon
Montana State University
-
Chad Giusti
University of Delaware
-
Harold Xavier Gonzalez
Stanford University
-
Chengcheng Huang
University of Pittsburgh
-
Vladimir Itskov
The Pennsylvania State University
-
Jonathan Jaquette
New Jersey Institute of Technology
-
Kresimir Josic
University of Houston
-
Sameer Kailasa
University of Michigan Ann Arbor
-
Gabriella Keszthelyi
Alfréd Rényi Institute of Mathematics
-
Zachary Kilpatrick
University of Colorado Boulder
-
Christopher Kim
National Institutes of Health
-
Soon Ho Kim
Georgia Institute of Technology
-
Hyunjoong Kim
University of Houston
-
Leo Kozachkov
Massachusetts Institute of Technology
-
Maxwell Kreider
Case Western Reserve University
-
Ankit Kumar
UC Berkeley
-
Yao Li
University of Massachusetts Amherst
-
Zelong Li
Penn State University
-
Caitlin Lienkaemper
Boston University
-
Kathryn Lindsey
Boston College
-
Vasiliki Liontou
ICERM
-
David Lipshutz
Flatiron Institute
-
Jessica Liu
CUNY Graduate Center
-
Sijing Liu
Brown University
-
Simon Locke
Johns Hopkins University
-
Laureline Logiaco
Massachusetts Institute of Technology
-
Juliana Londono Alvarez
Penn State
-
James MACLAURIN
New Jersey Institute of Technology
-
Matilde Marcolli
California Institute of Technology
-
Marissa Masden
ICERM
-
Nikola Milicevic
Pennsylvania State University
-
Federica Milinanni
KTH - Royal Institute of Technology
-
Konstantin Mischaikow
Rutgers University
-
Katie Morrison
University of Northern Colorado
-
Noga Mudrik
The Johns Hopkins University
-
Audrey Nash
Florida State University
-
matt nassar
Brown University
-
Gabe Ocker
Boston University
-
Choongseok Park
NC A&T State University
-
Ross Parker
Center for Communications Research – Princeton
-
Caitlyn Parmelee
Keene State College
-
Cengiz Pehlevan
Harvard University
-
Jose Perea
Northeastern University
-
Mason Porter
UCLA
-
Allan Raventos
Stanford University
-
Antonio Rieser
Centro de Investigación en Matemáticas
-
Jason Ritt
Brown University
-
Robert Rosenbaum
University of Notre Dame
-
Horacio Rotstein
New Jersey Institute of Technology
-
Safaan Sadiq
Pennsylvania State University
-
Nicole Sanderson
Penn State University
-
Hannah Santa Cruz
Penn State
-
Daniel Scott
Brown University
-
Thomas Serre
Brown University
-
Sage Shaw
University of Colorado Boulder
-
Nimrod Sherf
University of Houston
-
Farshad Shirani
Georgia Institute of Technology
-
Michael Shub
The City College of New York
-
Paramjeet Singh
Thapar Institute of Engineering & Technology
-
Dane Taylor
University of Wyoming
-
Peter Thomas
Case Western Reserve University
-
Tobias Timofeyev
University of Vermont
-
Nicholas Tolley
Brown University
-
Magnus Tournoy
Flatiron Institute
-
Wilson Truccolo
Brown University
-
Ka Nap Tse
University of Pittsburgh
-
Yangyang Wang
Brandeis University
-
Bin Wang
University of California, San Diego
-
Xinyi Wang
Michigan State University
-
Zhuo-Cheng Xiao
New York University
-
Iris Yoon
Wesleyan University
-
Ryeongkyung Yoon
University of Houston
-
Kei Yoshida
Brown University
-
Lai-Sang Young
Courant Institute
-
Nora Youngs
Colby College
-
Zhuojun Yu
Case Western Reserve University
-
Gexin Yu
College of William and Mary
-
Wenhao Zhang
UT Southwestern Medical Center
-
Ling Zhou
ICERM
-
Robert Zielinski
Brown University
Workshop Schedule
Monday, September 18, 2023
-
8:50 - 9:00 am EDTWelcome11th Floor Lecture Hall
- Session Chair
- Brendan Hassett, ICERM/Brown University
-
9:00 - 9:45 am EDTNeural dynamics on sparse networks—pruning, error correction, and signal reconstruction11th Floor Lecture Hall
- Speaker
- Rishidev Chaudhuri, University of California, Davis
- Session Chair
- Carina Curto, The Pennsylvania State University
Abstract
Many networks in the brain are sparsely connected, and the brain eliminates connections during development and learning. This talk will focus on questions related to computation and dynamics on these sparse networks. We will first focus on pruning redundant network connections while preserving dynamics and function. In a recurrent network, determining the importance of a connection between two neurons is a difficult computational problem, depending on the role that both neurons play and on all possible pathways of information flow between them. Noise is ubiquitous in neural systems, and often considered an irritant to be overcome. We suggest that noise could instead play a functional role in pruning, allowing the brain to probe network structure and determine which connections are redundant. We construct a simple, local, unsupervised rule that either strengthens or prunes synapses using only connection weight and the noise-driven covariance of the neighboring neurons. For a subset of linear and rectified-linear networks, we adapt matrix concentration of measure arguments from the field of graph sparsification to prove that this rule preserves the spectrum of the original matrix and hence preserves network dynamics even when the fraction of pruned connections asymptotically approaches 1. The plasticity rule is biologically-plausible and may suggest a new role for noise in neural computation. Time permitting, we will then discuss the application of sparse expander graphs to modeling dynamics on neural networks. Expander graphs combine the seemingly contradictory properties of being sparse and well-connected. Among other remarkable properties, they allow efficient communication, credit assignment and error correction with simple greedy dynamical rules. We suggest that these applications might provide new ways of thinking about neural dynamics, and provide several proofs of principle.
-
10:00 - 10:15 am EDTCoffee Break11th Floor Collaborative Space
-
10:15 - 11:00 am EDTLocal breakdown of the balance of excitation and inhibition accounts for divisive normalization11th Floor Lecture Hall
- Speaker
- Yashar Ahmadian, Cambridge University
- Session Chair
- Carina Curto, The Pennsylvania State University
Abstract
Excitatory and inhibitory (E & I) inputs to cortical neurons remain balanced across different conditions. This is captured in the balanced network model in which neural populations dynamically adjust their rates to yield tightly balanced E and I inputs and a state in which all neurons are active at levels observed in cortex. But global tight E-I balance predicts linear stimulus dependence for population responses, and does not account for systematic cortical response nonlinearities such as divisive normalization, a canonical brain computation. However, when necessary connectivity conditions for global balance fail, states arise in which a subset of neurons are inhibition dominated and inactive. Here, we show analytically that the emergence of such localized balance states robustly leads to normalization, including sublinear integration and winner-take-all behavior. An alternative model that exhibits normalization is the Stabilized Supralinear Network (SSN), in which the E-I balance is generically loose, but becomes tight asymptotically for strong inputs. However, an understanding of the causal relationship between E-I balance and normalization in SSN are lacking. Here we show that when tight E-I balance in the asymptotic, strongly driven regime of SSN is not global, the network does not exhibit normalization at any input strength; thus, in SSN too, significant normalization requires the breakdown of global balance. In summary, we causally and quantitatively connect a fundamental feature of cortical dynamics with a canonical brain computation.
-
11:15 - 11:45 am EDTOpen Problems DiscussionProblem Session - 11th Floor Lecture Hall
- Session Chairs
- Carina Curto, The Pennsylvania State University
- Katie Morrison, University of Northern Colorado
-
11:45 am - 1:30 pm EDTLunch/Free Time
-
1:30 - 2:15 pm EDTDiscovering dynamical patterns of activity from single-trial neural data11th Floor Lecture Hall
- Speaker
- Rodica Curtu, The University of Iowa
- Session Chair
- Carina Curto, The Pennsylvania State University
Abstract
In this talk I will discuss a data-driven method that leverages time-delayed coordinates, diffusion maps, and dynamic mode decomposition, to identify neural features in large scale brain recordings that correlate with subject-reported perception. The method captures the dynamics of perception at multiple timescales and distinguishes attributes of neural encoding of the stimulus from those encoding the perceptual states. Our analysis reveals a set of latent variables that exhibit alternating dynamics along a low-dimensional manifold, like trajectories of attractor-based models. I will conclude by proposing a phase-amplitude-coupling-based model that illustrates the dynamics of data.
-
2:30 - 2:35 pm EDTSynaptic mechanisms for resisting distractors in neural fieldsLightning Talks - 11th Floor Lecture Hall
- Speaker
- Heather Cihak, University of Colorado Boulder
- Session Chair
- Carina Curto, The Pennsylvania State University
Abstract
Persistent neural activity has been observed in the non-human primate cortex when making delayed estimates. Organized activity patterns according to cell feature preference reveals "bumps" that represent analog variables during the delay. Continuum neural field models support bump attractors whose stochastic dynamics can be linked to response statistics (estimate bias and error). Models often ignore the distinct dynamics of bumps in both excitatory/inhibitory population activity, but recent neural and behavioral recordings suggest both play a role in delayed estimate codes and responses. In past work, we developed new methods in asymptotic and multiscale analyses for stochastic and spatiotemporal systems to understand how network architecture determines bump dynamics in networks with distinct E/I populations and short term plasticity. The inhibitory bump dynamics as well as facilitation and diffusion impact the stability and wandering motion of the excitatory bump. Our current work moves beyond studying ensemble statistics like variance to examine potential mechanisms underlying the robustness of working memory to distractors (irrelevant information) presented during the maintenance period wherein the relative timescales of the E/I populations, synaptic vs activity dynamics, as well as short term plasticity may play an important role.
-
2:35 - 2:40 pm EDTConvex optimization of recurrent neural networks for rapid inference of neural dynamicsLightning Talks - 11th Floor Lecture Hall
- Speaker
- Fatih Dinc, Stanford University
- Session Chair
- Carina Curto, The Pennsylvania State University
Abstract
Advances in optical and electrophysiological recording technologies have made it possible to record the dynamics of thousands of neurons, opening up new possibilities for interpreting and controlling large neural populations. A promising way to extract computational principles from these large datasets is to train data-constrained recurrent neural networks (dRNNs). However, existing training algorithms for dRNNs are inefficient and have limited scalability, making it a challenge to analyze large neural recordings even in offline scenarios. To address these issues, we introduce a training method termed Convex Optimization of Recurrent Neural Networks (CORNN). In studies of simulated recordings of hundreds of cells, CORNN attained training speeds ~ 100-fold faster than traditional optimization approaches while maintaining or enhancing modeling accuracy. We further validated CORNN on simulations with thousands of cells that performed simple computations such as those of a 3-bit flip-flop or the execution of a timed response. Finally, we showed that CORNN can robustly reproduce network dynamics and underlying attractor structures despite mismatches between generator and inference models, severe subsampling of observed neurons, or mismatches in neural time-scales. Overall, by training dRNNs with millions of parameters in subminute processing times on a standard computer, CORNN constitutes a first step towards real-time network reproduction constrained on large-scale neural recordings and a powerful computational tool for advancing the understanding of neural computation. My talk focuses on how dRNNs enabled by CORNN can help us reverse engineer the neural code in the mammalian brain.
-
2:40 - 2:45 pm EDTRecall tempo of Hebbian sequences depends on the interplay of Hebbian kernel with tutor signal timingLightning Talks - 11th Floor Lecture Hall
- Speaker
- Matthew Farrell, Harvard University
- Session Chair
- Carina Curto, The Pennsylvania State University
Abstract
Understanding how neural circuits generate sequential activity is a longstanding challenge. While foundational theoretical models have shown how sequences can be stored as memories with Hebbian plasticity rules, these models considered only a narrow range of Hebbian rules. In this talk I introduce a model for arbitrary Hebbian plasticity rules, capturing the diversity of spike-timing-dependent synaptic plasticity seen in experiments, and show how the choice of these rules and of neural activity patterns influences sequence memory formation and retrieval. In particular, I will present a general theory that predicts the speed of sequence replay. This theory lays a foundation for explaining how cortical tutor signals might give rise to motor actions that eventually become ``automatic''. This theory also captures the impact of changing the speed of the tutor signal. Beyond shedding light on biological circuits, this theory has relevance in artificial intelligence by laying a foundation for frameworks whereby slow and computationally expensive deliberation can be stored as memories and eventually replaced by inexpensive recall.
-
2:45 - 2:50 pm EDTModeling human temporal EEG responses subject to VR visual stimuliLightning Talks - 11th Floor Lecture Hall
- Speaker
- Richard Foster, Virginia Commonwealth University
- Session Chair
- Carina Curto, The Pennsylvania State University
Abstract
When subject to visual stimuli flashing at a constant temporal frequency, it is well-known that the EEG response has a sharp peak in the power spectrum at the driving frequency. But the EEG response with random frequency stimuli and corresponding biophysical mechanisms are largely unknown. We present a phenomenological model framework in hopes of eventually capturing these EEG responses and unveiling the biophysical mechanisms. Based on observed heterogeneous temporal frequency selectivity curves in V1 cells (Hawken et al. ‘96, Camillo et al ‘20, Priebe et al. ‘06), we endow individual units with these response properties. Preliminary simulation results show that particular temporal frequency selectivity curves can be more indicative of the EEG response. Future directions include the construction of network architecture with interacting units to faithfully model the EEG response.
-
2:50 - 2:55 pm EDTRNNs of RNNs: Recursive Construction of Stable Assemblies of Recurrent Neural NetworksLightning Talks - 11th Floor Lecture Hall
- Speaker
- Leo Kozachkov, Massachusetts Institute of Technology
- Session Chair
- Carina Curto, The Pennsylvania State University
Abstract
Recurrent neural networks (RNNs) are widely used throughout neuroscience as models of local neural activity. Many properties of single RNNs are well characterized theoretically, but experimental neuroscience has moved in the direction of studying multiple interacting areas, and RNN theory needs to be likewise extended. We take a constructive approach towards this problem, leveraging tools from nonlinear control theory and machine learning to characterize when combinations of stable RNNs will themselves be stable. Importantly, we derive conditions which allow for massive feedback connections between interacting RNNs. We parameterize these conditions for easy optimization using gradient-based techniques, and show that stability-constrained "networks of networks" can perform well on challenging sequential-processing benchmark tasks. Altogether, our results provide a principled approach towards understanding distributed, modular function in the brain.
-
3:15 - 3:45 pm EDTCoffee Break11th Floor Collaborative Space
-
3:45 - 4:30 pm EDTUniversal Properties of Strongly Coupled Recurrent Networks11th Floor Lecture Hall
- Speaker
- Robert Rosenbaum, University of Notre Dame
- Session Chair
- Carina Curto, The Pennsylvania State University
Abstract
Balanced excitation and inhibition is widely observed in cortex. How does this balance shape neural computations and stimulus representations? This question is often studied using computational models of neuronal networks in a dynamically balanced state. But balanced network models predict a linear relationship between stimuli and population responses. So how do cortical circuits implement nonlinear representations and computations? We show that every balanced network architecture admits stimuli that break the balanced state and these breaks in balance push the network into a “semi-balanced state” characterized by excess inhibition to some neurons, but an absence of excess excitation. The semi-balanced state produces nonlinear stimulus representations and nonlinear computations, is unavoidable in networks driven by multiple stimuli, is consistent with cortical recordings, and has a direct mathematical relationship to artificial neural networks.
-
4:30 - 6:00 pm EDTReception11th Floor Collaborative Space
Tuesday, September 19, 2023
-
9:00 - 9:45 am EDTMultilayer Networks in Neuroscience11th Floor Lecture Hall
- Speaker
- Mason Porter, UCLA
- Session Chair
- Brent Doiron, University of Chicago
Abstract
I will discuss multilayer networks in neuroscience. I will introduce the idea of multilayer networks and discuss some uses of multilayer networks in dneuroscience. I will present some interesting challenges.
-
10:00 - 10:15 am EDTCoffee Break11th Floor Collaborative Space
-
10:15 - 11:00 am EDTState modulation in spatial networks of multiple interneuron subtypes11th Floor Lecture Hall
- Speaker
- Chengcheng Huang, University of Pittsburgh
- Session Chair
- Brent Doiron, University of Chicago
Abstract
Neuronal responses to sensory stimuli can be strongly modulated by animal's brain state. Three distinct subtypes of inhibitory interneurons, parvalbumin (PV), somatostatin (SOM), and vasoactive intestinal peptide (VIP) expressing cells, have been identified as key players of flexibly modulating network activity. The three interneuron populations have specialized local microcircuit motifs and are targeted differentially by neuromodulators and top-down inputs from higher-order cortical areas. In this work, we systematically study the function of each interneuron cell type at modulating network dynamics in a spatially ordered spiking neuron network. We analyze the changes in firing rates and network synchrony as we apply static current to each cell population. We find that the modulation pattern by activating E or PV cells is distinct from that by activating SOM or VIP cells. In particular, we identify SOM cells as the main driver of network synchrony.
-
11:15 - 11:45 am EDTOpen Problems DiscussionProblem Session - 11th Floor Lecture Hall
- Session Chairs
- Brent Doiron, University of Chicago
- Zachary Kilpatrick, University of Colorado Boulder
-
11:50 am - 12:00 pm EDTGroup Photo (Immediately After Talk)11th Floor Lecture Hall
-
12:00 - 1:30 pm EDTWorking Lunch11th Floor Collaborative Space
-
1:30 - 2:15 pm EDTPlasticity in balanced neuronal networks11th Floor Lecture Hall
- Speaker
- Kresimir Josic, University of Houston
- Session Chair
- Brent Doiron, University of Chicago
Abstract
I will first describe how to extend the theory of balanced networks to account for synaptic plasticity. This theory can be used to show when a plastic network will maintain balance, and when it will be driven into an unbalanced state. I will next discuss how this approach provides evidence for a novel form of rapid compensatory inhibitory plasticity using experimental evidence obtained using optogenetic activation of excitatory neurons in primate visual cortex (area V1). The theory explains how such activation induces a population-wide dynamic reduction in the strength of neuronal interactions over the timescale of minutes during the awake state, but not during rest. I will shift gears in the final part of the talk, and discuss how community detection algorithms can help uncover the large scale organization of neuronal networks from connectome data, using the Drosophila hemibrain dataset as an example.
-
2:35 - 2:40 pm EDTQ-Phase reduction of multi-dimensional stochastic Ornstein-Uhlenbeck process networksLightning Talks - 11th Floor Lecture Hall
- Speaker
- Maxwell Kreider, Case Western Reserve University
- Session Chair
- Brent Doiron, University of Chicago
Abstract
Phase reduction is an effective tool to study the network dynamics of deterministic limit-cycle oscillators. The recent introduction of stochastic phase concepts allows us to extend these tools to stochastic oscillators; of particular utility is the asymptotic stochastic phase, derived from the eigenfunction decomposition of the system's probability density. Here, we study networks of coupled oscillatory two-dimensional Ornstein-Uhlenbeck processes (OUPs) with complex eigenvalues. We characterize system dynamics by providing an exact expression for the asymptotic stochastic phase for OUP networks of any dimension and arbitrary coupling structure. Furthermore, we introduce an order parameter quantifying the synchrony of networks of stochastic oscillators, and apply it to our OUP model. We argue that the OUP network provides a new, analytically tractable approach to analysis of large scale electrophysiological recordings.
-
2:40 - 2:45 pm EDTFeedback Controllability as a Normative Theory of Neural DynamicsLightning Talks - 11th Floor Lecture Hall
- Speaker
- Ankit Kumar, UC Berkeley
- Session Chair
- Brent Doiron, University of Chicago
Abstract
Brain computations emerge from the collective dynamics of distributed neural populations. Behaviors including reaching and speech are explained by principles of optimal feedback control, yet if and how this normative description shapes neural population dynamics is unknown. We created dimensionality reduction methods that identify subspaces of dynamics that are most feedforward controllable (FFC) vs. feedback controllable (FBC). We show that FBC and FFC subspaces diverge for dynamics generated by non-normal connectivity. In neural recordings from monkey M1 and S1 during reaching, FBC subspaces are better decoders of reach velocity, particularly during reach acceleration, and that FBC provides a first principles account of the observation of rotational dynamics. Overall, our results demonstrate feedback controllability is a novel, normative theory of neural population dynamics, and reveal how the structure of high dynamical systems shape their ability to be controlled.
-
2:45 - 2:50 pm EDTAdaptive whitening with fast gain modulation and slow synaptic plasticityLightning Talks - 11th Floor Lecture Hall
- Speaker
- David Lipshutz, Flatiron Institute
- Session Chair
- Brent Doiron, University of Chicago
Abstract
Neurons in early sensory areas rapidly adapt to changing sensory statistics, both by normalizing the variance of their individual responses and by reducing correlations between their responses. Together, these transformations may be viewed as an adaptive form of statistical whitening. In this talk, I will present a normative multi-timescale mechanistic model of adaptive whitening with complementary computational roles for gain modulation and synaptic plasticity. Gains are modified on a fast timescale to adapt to the current statistical context, whereas synapses are modified on a slow timescale to learn structural properties of the input statistics that are invariant across contexts.
-
2:50 - 2:55 pm EDTThe combinatorial code and the graph rules of Dale networksLightning Talks - 11th Floor Lecture Hall
- Speaker
- Nikola Milicevic, Pennsylvania State University
- Session Chair
- Brent Doiron, University of Chicago
Abstract
We describe the combinatorics of equilibria and steady states of neurons in threshold-linear networks that satisfy the Dale’s law. The combinatorial code of a Dale network is characterized in terms of two conditions: (i) a condition on the network connectivity graph, and (ii) a spectral condition on the synaptic matrix. We find that in the weak coupling regime the combinatorial code depends only on the connectivity graph, and not on the particulars of the synaptic strengths. Moreover, we prove that the combinatorial code of a weakly coupled network is a sublattice, and we provide a learning rule for encoding a sublattice in a weakly coupled excitatory network. In the strong coupling regime we prove that the combinatorial code of a generic Dale network is intersection-complete and is therefore a convex code, as is common in some sensory systems in the brain.
-
2:55 - 3:00 pm EDTDecomposed Linear Dynamical Systems for Studying Inter and Intra-Region Neural DynamicsLightning Talks - 11th Floor Lecture Hall
- Speaker
- Noga Mudrik, The Johns Hopkins University
- Session Chair
- Brent Doiron, University of Chicago
Abstract
Understanding the intricate relationship between recorded neural activity and behavior is a pivotal pursuit in neuroscience. However, existing models frequently overlook the non-linear and non-stationary behavior evident in neural data, opting instead to center their focus on simplified projections or overt dynamical systems. We introduce a Decomposed Linear Dynamical Systems (dLDS) approach to capture these complex dynamics by representing them as a sparse time-varying linear combination of interpretable linear dynamical components. dLDS is trained using an expectation maximization procedure where the obscured dynamical components are iteratively inferred using dictionary learning. This approach enables the identification of overlapping circuits, while the sparsity applied during the training maintains the model interpretability. We demonstrate that dLDS successfully recovers the underlying linear components and their time-varying coefficients in both synthetic and neural data examples, and show that it can learn efficient representations of complex data. By leveraging the rich data from the International Brain Laboratory’s Brain Wide Map dataset, we extend dLDS to model communication among ensembles within and between brain regions, drawing insights from multiple non-simultaneous recording sessions.
-
3:00 - 3:05 pm EDTCharacterizing Neural Spike Train Data for Chemosensory Coding AnalysisLightning Talks - 11th Floor Lecture Hall
- Speaker
- Audrey Nash, Florida State University
- Session Chair
- Brent Doiron, University of Chicago
Abstract
In this presentation, we explore neural spike train data to discern a neuron's ability to distinguish between various stimuli. By examining both the spiking rate and the temporal distribution of spikes (phase of spiking), we aim to unravel the intricacies of chemosensory coding in neurons. We will provide a concise overview of our methodology for identifying chemosensory coding neurons and delve into the application of metric-based analysis techniques in conjunction with optimal transport methods. This combined approach allows us to uncover emerging patterns in tastant coding across multiple neurons and quantify the respective impacts of spiking rate and temporal phase in taste decoding.
-
3:05 - 3:10 pm EDTInfinite-dimensional Dynamics in a Model of EEG Activity in the NeocortexLightning Talks - 11th Floor Lecture Hall
- Speaker
- Farshad Shirani, Georgia Institute of Technology
- Session Chair
- Brent Doiron, University of Chicago
Abstract
I present key analytical and computational results on a mean field model of electroencephalographic activity in the neocortex, which is composed of a system of coupled ODEs and PDEs. I show that for some sets of biophysical parameter values the equilibrium set of the model is not compact, which further implies that the global attracting set of the model is infinite-dimensional. I also present computational results on generation and spatial propagation of transient gamma oscillations in the solutions of the model. The results identify important challenges in interpreting and modelling the temporal pattern of EEG recordings, caused by low spatial resolution of EEG electrodes.
-
3:10 - 3:15 pm EDTWhat is the optimal topology of setwise connections for a memory network?Lightning Talks - 11th Floor Lecture Hall
- Speaker
- Thomas Burns, ICERM
- Session Chair
- Brent Doiron, University of Chicago
Abstract
Simplicial Hopfield networks (Burns & Fukai, 2023) explicitly model setwise connections between neurons based on a simplicial complex to store memory patterns. Randomly diluted networks -- where only a randomly chosen fraction of the simplices, i.e., setwise connections, have non-zero weights -- show performance above traditional associative memory networks with only pairwise connections between neurons but the same total number of non-zero weighted connections. However, could there be a cleverer choice of connections to weight given known memory patterns we want to store? I suspect so, and in this talk I will to formally pose the problem for others to consider.
-
3:30 - 4:00 pm EDTCoffee Break11th Floor Collaborative Space
-
4:00 - 4:45 pm EDTReliability and robustness of oscillations in some slow-fast chaotic systems11th Floor Lecture Hall
- Speaker
- Jonathan Jaquette, New Jersey Institute of Technology
- Session Chair
- Brent Doiron, University of Chicago
Abstract
A variety of nonlinear models of biological systems generate complex chaotic behaviors that contrast with biological homeostasis, the observation that many biological systems prove remarkably robust in the face of changing external or internal conditions. Motivated by the subtle dynamics of cell activity in a crustacean central pattern generator, we propose a refinement of the notion of chaos that reconciles homeostasis and chaos in systems with multiple timescales. We show that systems displaying relaxation cycles going through chaotic attractors generate chaotic dynamics that are regular at macroscopic timescales, thus consistent with physiological function. We further show that this relative regularity may break down through global bifurcations of chaotic attractors such as crises, beyond which the system may generate erratic activity also at slow timescales. We analyze in detail these phenomena in the chaotic Rulkov map, a classical neuron model known to exhibit a variety of chaotic spike patterns. This leads us to propose that the passage of slow relaxation cycles through a chaotic attractor crisis is a robust, general mechanism for the transition between such dynamics, and we validate this numerically in other models.
-
5:30 - 7:00 pm EDTNetworking event with Carney Institute for Brain ScienceExternal Event - Carney Institute for Brain Science - 164 Angell St, Providence RI, 02906
Wednesday, September 20, 2023
-
9:00 - 9:45 am EDTModeling in neuroscience: the challenges of biological realism and computability11th Floor Lecture Hall
- Speaker
- Lai-Sang Young, Courant Institute
- Session Chair
- Katie Morrison, University of Northern Colorado
Abstract
Biologically realistic models of the brain have the potential to offer insight into neural mechanisms; they have predictive power, the ultimate goal of biological modeling. These benefits, however, come at considerable costs: network models that involve hundreds of thousands of neurons and many (unknown) parameters are unwieldy to build and to test, let alone to simulate and to analyze. Reduced models have obvious advantages, but the farther removed from biology a model is, the harder it is to draw meaningful inferences. In this talk, I propose a modeling strategy that aspires to be both realistic and computable. Two crucial ingredients are (i) we track neuronal dynamics on two spatial scales: coarse-grained dynamics informed by local activity, and (ii) we compute a family of potential local responses in advance, eliminating the need to perform similar computations at each spatial location in each update. I will illustrate this computational strategy using a model of the monkey visual cortex, which is very similar to that of humans.
-
10:00 - 10:15 am EDTCoffee Break11th Floor Collaborative Space
-
10:15 - 11:00 am EDTUncertainty Quantification for Neurobiological Networks.11th Floor Lecture Hall
- Speaker
- Daniele Avitabile, Vrije Universiteit Amsterdam
- Session Chair
- Katie Morrison, University of Northern Colorado
Abstract
This talk presents a framework for forward uncertainty quantification problems in spatially-extended neurobiological networks. We will consider networks in which the cortex is represented as a continuum domain, and local neuronal activity evolves according to an integro-differential equation, collecting inputs nonlocally, from the whole cortex. These models are sometimes referred to as neural field equations. Large-scale brain simulations of such models are currently performed heuristically, and the numerical analysis of these problems is largely unexplored. In the first part of the talk I will summarise recent developments for the rigorous numerical analysis of projection schemes for deterministic neural fields, which sets the foundation for developing Finite-Element and Spectral schemes for large-scale problems. The second part of the talk will discuss the case of networks in the presence of uncertainties modelled with random data, in particular: random synaptic connections, external stimuli, neuronal firing rates, and initial conditions. Such problems give rise to random solutions, whose mean, variance, or other quantities of interest have to be estimated using numerical simulations. This so-called forward uncertainty quantification problem is challenging because it couples spatially nonlocal, nonlinear problems to large-dimensional random data. I will present a family of schemes that couple a spatial projector for the spatial discretisation, to stochastic collocation for the random data. We will analyse the time- dependent problem with random data and the schemes from a functional analytic viewpoint, and show that the proposed methods can achieve spectral accuracy, provided the random data is sufficiently regular. We will showcase the schemes using several examples. Acknowledgements This talk presents joint work with Francesca Cavallini (VU Amsterdam), Svetlana Dubinkina (VU Amsterdam), and Gabriel Lord (Radboud University).
-
11:15 am - 12:00 pm EDTOpen Problems DiscussionProblem Session - 11th Floor Lecture Hall
- Session Chairs
- Konstantin Mischaikow, Rutgers University
- Katie Morrison, University of Northern Colorado
-
12:00 - 2:00 pm EDTLunch/Free Time
-
2:00 - 2:45 pm EDTDynamics of stochastic integrate-and-fire networks11th Floor Lecture Hall
- Speaker
- Gabe Ocker, Boston University
- Session Chair
- Katie Morrison, University of Northern Colorado
-
3:00 - 3:05 pm EDTA Step Towards Uncovering The Structure of Multistable Neural NetworksLightning Talks - 11th Floor Lecture Hall
- Speaker
- Magnus Tournoy, Flatiron Institute
- Session Chair
- Katie Morrison, University of Northern Colorado
Abstract
With the experimental advances in the recording of large populations of neurons, theorists are in the humbling position of making sense of a staggering amount of data. One question that will become more into reach is how network structure relates to function. But going beyond explanatory models and becoming more predictive will require a fundamental approach. In this talk we’ll take the view of a physicist and formulate exact results within a simple, yet general, toy model called Glass networks. Named after its originator Leon Glass, they are the infinite gain limit of well-known circuit models like continuous-time Hopfield networks. We’ll show that, within this limit, stability conditions reduce to semipositivity constraints on the synaptic weight matrix. Having a clear link between structure and function in possession, the consequences of multistability on the network architecture can be explored. One finding is the factorization of the weight matrix in terms of nonnegative matrices. Interestingly this factorization completely identifies the existence of stable states. Another result is the reduction of allowed sign patterns for the connections. A consequence hereof are lower bounds on the number of excitatory and inhibitory connections. At last we will discuss the special case of “sign stability”, where stability is guaranteed by the topology of the network. Derivations of these results will be supplemented by a number of examples.
-
3:05 - 3:10 pm EDTClustering and Distribution of the Adaptation VariableLightning Talks - 11th Floor Lecture Hall
- Speaker
- Ka Nap Tse, University of Pittsburgh
- Session Chair
- Katie Morrison, University of Northern Colorado
Abstract
Brain wave is an important phenomenon in neuroscience. Besides synchronous spiking, excitatory cells with adaptation can spike in clusters to cause a rhythmic activity of the network. In previous works, the adaptation variable is usually eliminated for further analysis. In this talk, a way to study this clustering behaviour through the evolution of the distribution of the adaptation variable will be discussed. We then transform the distribution to the time-to-spike coordinate for further explorations.
-
3:10 - 3:15 pm EDTLow-dimensional manifold of neural oscillations revealed by data-driven model reductionLightning Talks - 11th Floor Lecture Hall
- Speaker
- Zhuo-Cheng Xiao, New York University
- Session Chair
- Katie Morrison, University of Northern Colorado
Abstract
Neural oscillations across various frequency bands are believed to underlie essential brain functions, such as information processing and cognitive activities. However, the emergence of oscillatory dynamics from spiking neuronal networks—and the interplay among different cortical rhythms—has seldom been theoretically explored, largely due to the strong nonlinearity and high dimensionality involved. To address this challenge, we have developed a series of data-driven model reduction methods tailored for spiking network dynamics. In this talk I will present nearly two-dimensional manifolds in the reduced coordinates that successfully capture the emergence of gamma oscillations. Specifically, we find that the initiation phases of each oscillation cycle are the most critical. Subsequent cycles are more deterministic and lie on the aforementioned two-dimensional manifold. The Poincaré mappings between these initiation phases reveal the structure of the dynamical system and successfully explain the bifurcation from gamma oscillations to multi-band oscillations.
-
3:15 - 3:20 pm EDTSensitivity to control signals in triphasic rhythmic neural systems: a comparative mechanistic analysis via infinitesimal local timing response curvesLightning Talks - 11th Floor Lecture Hall
- Speaker
- Zhuojun Yu, Case Western Reserve University
- Session Chair
- Katie Morrison, University of Northern Colorado
Abstract
Similar activity patterns may arise from model neural networks with distinct coupling properties and individual unit dynamics. These similar patterns may, however, respond differently to parameter variations and, specifically, to tuning of inputs that represent control signals. In this work, we analyze the responses resulting from modulation of a localized input in each of three classes of model neural networks that have been recognized in the literature for their capacity to produce robust three-phase rhythms: coupled fast-slow oscillators, near-heteroclinic oscillators, and threshold-linear networks. Triphasic rhythms, in which each phase consists of a prolonged activation of a corresponding subgroup of neurons followed by a fast transition to another phase, represent a fundamental activity pattern observed across a range of central pattern generators underlying behaviors critical to survival, including respiration, locomotion, and feeding. To perform our analysis, we extend the recently developed local timing response curve (lTRC), which allows us to characterize the timing effects due to perturbations, and we complement our lTRC approach with model-specific dynamical systems analysis. Interestingly, we observe disparate effects of similar perturbations across distinct model classes. Thus, this work provides an analytical framework for studying control of oscillations in nonlinear dynamical systems, and may help guide model selection in future efforts to study systems exhibiting triphasic rhythmic activity.
-
3:20 - 3:25 pm EDTModeling the effects of cell-type specific lateral inhibitionLightning Talks - 11th Floor Lecture Hall
- Speaker
- Soon Ho Kim, Georgia Institute of Technology
- Session Chair
- Katie Morrison, University of Northern Colorado
-
3:30 - 4:00 pm EDTCoffee Break11th Floor Collaborative Space
-
4:00 - 4:45 pm EDTComputing the Global Dynamics of Parameterized Families of ODEs11th Floor Lecture Hall
- Speaker
- Marcio Gameiro, Rutgers University
- Session Chair
- Katie Morrison, University of Northern Colorado
Abstract
We present a combinatorial topological method to compute the dynamics of a parameterized family of ODEs. A discretization of the state space of the systems is used to construct a combinatorial representation from which recurrent versus non-recurrent dynamics is extracted. Algebraic topology is then used to validate and characterize the dynamics of the system. We will discuss the combinatorial description and the algebraic topological computations and will present applications to systems of ODEs arising from gene regulatory networks.
Thursday, September 21, 2023
-
9:00 - 9:45 am EDTMultiple timescale respiratory dynamics and effect of neuromodulation11th Floor Lecture Hall
- Speaker
- Yangyang Wang, Brandeis University
- Session Chair
- Zachary Kilpatrick, University of Colorado Boulder
Abstract
Respiration is an involuntary process in all living beings required for our survival. The preBötzinger complex (preBötC) in the mammalian brainstem is a neuronal network that drives inspiratory rhythmogenesis, whose activity is constantly modulated by neuromodulators in response to changes in the environment. In this talk, we will discuss chanllenges involved in the analysis of bursting dynamics in preBötC neurons and how these dynamics change during prenatal development. We will also combine insights from in vitro recordings and dynamical systems modeling to investigate the effect of norepinephrine (NE), an excitatory neuromodulator, on respiratory dynamics. Our investigation employs bifurcation analysis to reveal the mechanisms by which NE differentially modulates different types of preBötC bursting neurons.
-
10:00 - 10:30 am EDTCoffee Break11th Floor Collaborative Space
-
10:30 - 11:15 am EDTEnhancing Neuronal Classification Capacity via Nonlinear Parallel Synapses11th Floor Lecture Hall
- Speaker
- Marcus Benna, UC San Diego
- Session Chair
- Zachary Kilpatrick, University of Colorado Boulder
Abstract
We discuss models of a neuron that has multiple synaptic contacts with the same presynaptic axon. We show that a diverse set of learned nonlinearities in these parallel synapses leads to a substantial increase in the neuronal classification capacity.
-
11:30 am - 1:30 pm EDTWorking Lunch: Open Problems SessionWorking Lunch - 11th Floor Collaborative Space
-
1:30 - 2:15 pm EDTCombinatorial structure of continuous dynamics in gene regulatory networks11th Floor Lecture Hall
- Speaker
- Tomas Gedeon, Montana State University
- Session Chair
- Zachary Kilpatrick, University of Colorado Boulder
Abstract
Gene network dynamics and neural network dynamics face similar challenges of high dimensionality of both phase space and parameter space, and a lack of reliable experimental data to infer parameters. We first describe the mathematical foundation of DSGRN (Dynamic Signatures Generated by Regulatory Networks), an approach that provides a combinatorial description of global dynamics of a network over its parameter space. Finite description allows comparison of parameterized dynamics between hundreds of networks to discard networks that are not compatible with experimental data. We also describe a close connection of DSGRN to Boolean network models that allows us to view DSGRN as a connection between parameterized continuous time dynamics and discrete dynamics of Boolean modets. If time allows, we discuss several applications of this methodology to systems biology.
-
2:30 - 3:15 pm EDTA model of the mammalian neural motor architecture elucidates the mechanisms underlying efficient and flexible control of network dynamics11th Floor Lecture Hall
- Speaker
- Laureline Logiaco, Massachusetts Institute of Technology
- Session Chair
- Zachary Kilpatrick, University of Colorado Boulder
Abstract
One of the fundamental functions of the brain is to flexibly plan and control movement production at different timescales in order to efficiently shape structured behaviors. I will present research investigating how these complex computations are performed in the mammalian brain, with an emphasis on autonomous motor control. Specifically, I will focus on the mechanisms supporting efficient interfacing between 'higher-level' planning commands and 'lower-level' motor cortical dynamics that ultimately drive muscles. I will take advantage of the fact that the anatomy of the circuits underlying motor control is well known. It notably involves the primary motor cortex, a recurrent network that generates learned commands to drive muscles while interacting through loops with thalamic neurons that lack recurrent excitation. Using an analytically tractable model that incorporates these architectural constraints, I will explain how this motor circuit can implement a form of efficient modularity by combining (i) plastic thalamocortical loops that are movement-specific and (ii) shared hardwired circuits. I will show that this modular architecture can balance two different objectives: first, supporting the flexible recombination of an extensible library of re-usable motor primitives; and second, promoting the efficient use of neural resources by taking advantage of shared connections between modules. I will end by mentioning some open avenues for further mathematical analyses related to this framework.
-
3:30 - 4:00 pm EDTCoffee Break11th Floor Collaborative Space
-
4:00 - 4:45 pm EDTLow-rank neural connectivity for the discrimination of temporal patterns.11th Floor Lecture Hall
- Speaker
- Sean Escola, Columbia University
- Session Chair
- Zachary Kilpatrick, University of Colorado Boulder
Friday, September 22, 2023
-
9:00 - 9:45 am EDTMean-field theory of learning dynamics in deep neural networks11th Floor Lecture Hall
- Speaker
- Cengiz Pehlevan, Harvard University
- Session Chair
- Konstantin Mischaikow, Rutgers University
Abstract
Learning dynamics of deep neural networks is complex. While previous approaches made advances in mathematical analysis of the dynamics of two-layer neural networks, addressing deeper networks have been challenging. In this talk, I will present a mean field theory of the learning dynamics of deep networks and discuss its implications.
-
10:00 - 10:45 am EDTMulti-level measures for understanding and comparing biological and artificial neural networks11th Floor Lecture Hall
- Speaker
- SueYeon Chung, New York University
- Session Chair
- Konstantin Mischaikow, Rutgers University
Abstract
I will share recent theoretical advances on how representation's population level properties such as high-dimensional geometries and spectral properties can be used to capture (1) the classification capacity of neural manifolds, and (2) prediction error of neural data from network model representations.
-
11:00 - 11:30 am EDTCoffee Break11th Floor Collaborative Space
-
11:30 am - 12:15 pm EDTA Sparse-coding Model of Category-specific Functional Organization in IT Cortex11th Floor Lecture Hall
- Speaker
- Demba Ba, Harvard University
- Session Chair
- Konstantin Mischaikow, Rutgers University
Abstract
Primary sensory areas in the brain of mammals may have evolved to compute efficient representations of natural scenes. In the late 90s, Olhausen and Field proposed a model that expresses the components of a natural scene, e.g. natural-image patches, as sparse combinations of a common set of patterns. Applied to a dataset of natural images, this so-called sparse coding model learns patterns that resemble the receptive fields of V1 neurons. Recordings from the monkey infero-temporal (IT) cortex suggest the presence, in this region, of a sparse code for natural-image categories. The recordings also suggest that, physically, IT neurons form spatial clusters, each of which preferentially responds to images from certain categories. Taken together, this evidence suggests that neurons in IT cortex form functional groups that reflect the grouping of natural images into categories. My talk will introduce a new sparse-coding model that exhibits this categorical form of functional grouping.
-
12:30 - 2:00 pm EDTLunch/Free Time
-
2:00 - 2:45 pm EDTFinal Open Problems DiscussionProblem Session - 11th Floor Lecture Hall
- Session Chairs
- Carina Curto, The Pennsylvania State University
- Konstantin Mischaikow, Rutgers University
-
3:00 - 3:30 pm EDTCoffee Break11th Floor Collaborative Space
All event times are listed in ICERM local time in Providence, RI (Eastern Daylight Time / UTC-4).
All event times are listed in .
ICERM local time in Providence, RI is Eastern Daylight Time (UTC-4). Would you like to switch back to ICERM time or choose a different custom timezone?
Request Reimbursement
This section is for general purposes only and does not indicate that all attendees receive funding. Please refer to your personalized invitation to review your offer.
- ORCID iD
- As this program is funded by the National Science Foundation (NSF), ICERM is required to collect your ORCID iD if you are receiving funding to attend this program. Be sure to add your ORCID iD to your Cube profile as soon as possible to avoid delaying your reimbursement.
- Acceptable Costs
-
- 1 roundtrip between your home institute and ICERM
- Flights on U.S. or E.U. airlines – economy class to either Providence airport (PVD) or Boston airport (BOS)
- Ground Transportation to and from airports and ICERM.
- Unacceptable Costs
-
- Flights on non-U.S. or non-E.U. airlines
- Flights on U.K. airlines
- Seats in economy plus, business class, or first class
- Change ticket fees of any kind
- Multi-use bus passes
- Meals or incidentals
- Advance Approval Required
-
- Personal car travel to ICERM from outside New England
- Multiple-destination plane ticket; does not include layovers to reach ICERM
- Arriving or departing from ICERM more than a day before or day after the program
- Multiple trips to ICERM
- Rental car to/from ICERM
- Flights on a Swiss, Japanese, or Australian airlines
- Arriving or departing from airport other than PVD/BOS or home institution's local airport
- 2 one-way plane tickets to create a roundtrip (often purchased from Expedia, Orbitz, etc.)
- Travel Maximum Contributions
-
- New England: $350
- Other contiguous US: $850
- Asia & Oceania: $2,000
- All other locations: $1,500
- Note these rates were updated in Spring 2023 and superseded any prior invitation rates. Any invitations without travel support will still not receive travel support.
- Reimbursement Requests
-
Request Reimbursement with Cube
Refer to the back of your ID badge for more information. Checklists are available at the front desk and in the Reimbursement section of Cube.
- Reimbursement Tips
-
- Scanned original receipts are required for all expenses
- Airfare receipt must show full itinerary and payment
- ICERM does not offer per diem or meal reimbursement
- Allowable mileage is reimbursed at prevailing IRS Business Rate and trip documented via pdf of Google Maps result
- Keep all documentation until you receive your reimbursement!
- Reimbursement Timing
-
6 - 8 weeks after all documentation is sent to ICERM. All reimbursement requests are reviewed by numerous central offices at Brown who may request additional documentation.
- Reimbursement Deadline
-
Submissions must be received within 30 days of ICERM departure to avoid applicable taxes. Submissions after thirty days will incur applicable taxes. No submissions are accepted more than six months after the program end.