Organizing Committee
- Carina Curto
The Pennsylvania State University - Robert Ghrist
University of Pennsylvania - Kathryn Hess
EPFL - Matilde Marcolli
California Institute of Technology - Elad Schneidman
Weizmann Institute of Science - Tatyana Sharpee
Salk Institute
Abstract
In the last decade or so, applied topology and algebraic geometry have come into their own as vibrant areas of applied mathematics. At the same time, ideas and tools from topology and geometry have infiltrated theoretical and computational neuroscience. This kind of mathematics has shown itself to be a natural and useful language not only for analyzing neural data sets but also as a means of understanding principles of neural coding and computation. This workshop will bring together leading researchers at the interfaces of topology, geometry, and neuroscience to take stock of recent work and outline future directions. This includes a focus on topological data analysis (persistent homology and related methods), topological analysis of neural networks and their dynamics, topological decoding of neural activity, evolving topology of dynamic networks (e.g., networks that are changing as a result of learning), and analysis of connectome data. Related topics may include the geometry and topology of deep learning, as well as low-dimensional projections of trained networks.
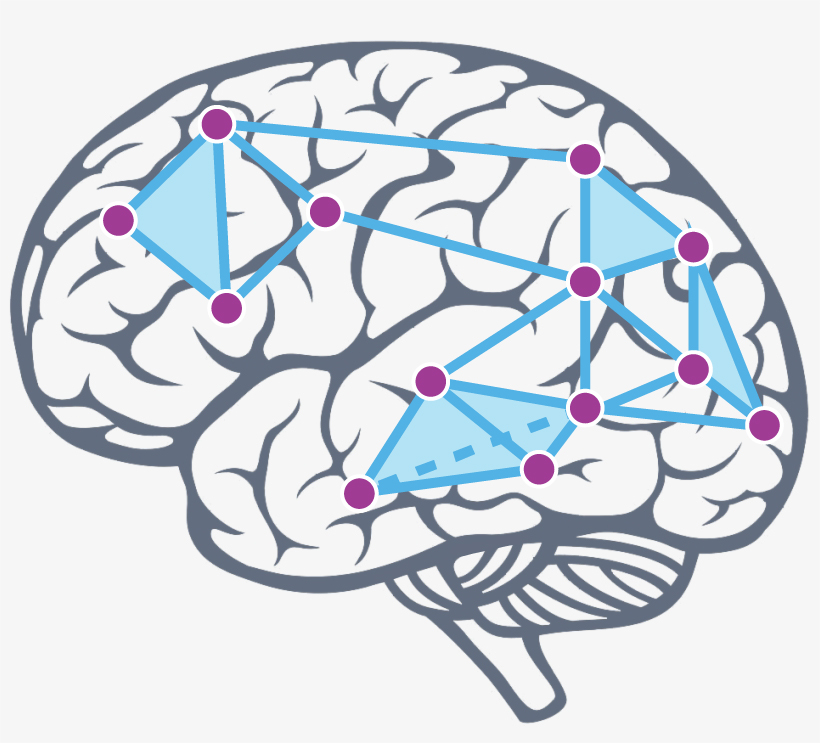
Confirmed Speakers & Participants
Talks will be presented virtually or in-person as indicated in the schedule below.
- Speaker
- Poster Presenter
- Attendee
- Virtual Attendee
-
Daniele Avitabile
Vrije Universiteit Amsterdam
-
Huseyin Ayhan
Florida State University
-
Aishwarya Balwani
Georgia Institute of Technology
-
Andrea Barreiro
Southern Methodist University
-
Dhananjay Bhaskar
Yale University
-
Ginestra Bianconi
Queen Mary University of London
-
Amitabha Bose
New Jersey Institute of Technology
-
Felipe Branco de Paiva
University of Wisconsin-Madison
-
Robyn Brooks
University of Utah
-
Peter Bubenik
University of Florida
-
Thomas Burns
ICERM
-
Johnathan Bush
University of Florida
-
Carlos Castañeda Castro
Brown University
-
Francesca Cavallini
Vrije Universiteit Amsterdam
-
Dmitri Chklovskii
Flatiron Institute & NYU Neuroscience Institute
-
Giovanna Citti
university of Bologna
-
Justin Curry
University at Albany SUNY
-
Carina Curto
The Pennsylvania State University
-
Juan Carlos Díaz-Patiño
Universidad Nacional Autónoma de México
-
Benjamin Dunn
Norwegian University of Science and Technology
-
Sophia Epstein
University of Texas at Austin
-
Julio Esparza Ibanez
Instituto Cajal - CSIC (Spanish National Research Council)
-
Ashkan Faghiri
Georgia state university
-
Michael Frank
Brown University
-
Halley Fritze
University of Oregon
-
Marcio Gameiro
Rutgers University
-
Tomas Gedeon
Montana State University
-
Robert Ghrist
University of Pennsylvania
-
Chad Giusti
Oregon State University
-
Anna Grim
Allen Institute
-
Robert Gütig
Charité Medical School Berlin
-
Todd Hagen
Bernstein Center for Computational Neuroscience
-
Erik Hermansen
Norwegian University of Scienc
-
Abigail Hickok
Columbia University
-
Christian Hirsch
Aarhus University
-
Iris Horng
University of Pennsylvania
-
Ching-Peng Huang
UKE
-
Vladimir Itskov
The Pennsylvania State University
-
Yuchen Jiang
Australian National University
-
Alvin Jin
Berkeley
-
Sameer Kailasa
University of Michigan Ann Arbor
-
Lida Kanari
EPFL/Blue Brain
-
Kevin Knudson
University of Florida
-
Maxwell Kreider
Case Western Reserve University
-
Kishore Kuchibhotla
Johns Hopkins University
-
Giancarlo La Camera
Stony Brook University
-
Kang-Ju Lee
Seoul National University
-
Ran Levi
University of Aberdeen
-
Noah Lewis
Georgia Institute of Technology
-
Yao Li
University of Massachusetts Amherst
-
Zelong Li
Penn State University
-
Johnny Li
UCSD
-
Caitlin Lienkaemper
Boston University
-
Kathryn Lindsey
Boston College
-
Vasiliki Liontou
ICERM
-
Sijing Liu
Brown University
-
Juliana Londono Alvarez
Penn State
-
Caio Lopes
École Polytechnique Fédérale de Lausanne
-
Matilde Marcolli
California Institute of Technology
-
Marissa Masden
ICERM
-
Nikola Milicevic
Pennsylvania State University
-
Federica Milinanni
KTH - Royal Institute of Technology
-
Katie Morrison
University of Northern Colorado
-
matt nassar
Brown University
-
Fernando Nobrega Santos
University of Amsterdam
-
Gabe Ocker
Boston University
-
Ross Parker
Center for Communications Research – Princeton
-
Caitlyn Parmelee
Keene State College
-
alice patania
University of Vermont
-
Cengiz Pehlevan
Harvard University
-
Isabella Penido
Brown University
-
Jose Perea
Northeastern University
-
Giovanni Petri
CENTAI Institute
-
Niloufar Razmi
Brown University
-
Alex Reyes
New York University
-
Antonio Rieser
Centro de Investigación en Matemáticas
-
Dmitry Rinberg
New York University
-
Dario Ringach
University of California, Los Angeles
-
Jason Ritt
Brown University
-
Horacio Rotstein
New Jersey Institute of Technology
-
Jennifer Rozenblit
University of Texas, Austin
-
Safaan Sadiq
Pennsylvania State University
-
Nicole Sanderson
Penn State University
-
Hannah Santa Cruz
Penn State
-
Alessandro Sarti
National Center of Scientific Research, EHESS, Paris
-
Nikolas Schonsheck
University of Delaware
-
David Schwab
City University of New York
-
Daniel Scott
Brown University
-
Thomas Serre
Brown University
-
Patrick Shipman
Colorado State University
-
Bernadette Stolz
EPFL
-
Evelyn Tang
Rice University
-
Dane Taylor
University of Wyoming
-
Peter Thomas
Case Western Reserve University
-
Tobias Timofeyev
University of Vermont
-
Nicholas Tolley
Brown University
-
Magnus Tournoy
Flatiron Institute
-
Wilson Truccolo
Brown University
-
Ka Nap Tse
University of Pittsburgh
-
Junyi Tu
Salisbury University
-
Srinivas Turaga
HHMI - Janelia Research Campus
-
Melvin Vaupel
Norwegian Institute of Science and Technology
-
Jonathan Victor
Weill Cornell Medical College
-
Elizabeth Vidaurre
Molloy University
-
Bradley Vigil
Texas Tech University
-
Zhengchao Wan
University of California San Diego
-
Bin Wang
University of California, San Diego
-
Xinyi Wang
Michigan State University
-
Zhuo-Cheng Xiao
New York University
-
Iris Yoon
Wesleyan University
-
Kisung You
City University of New York
-
Nora Youngs
Colby College
-
Zhuojun Yu
Case Western Reserve University
-
Wenhao Zhang
UT Southwestern Medical Center
-
Ling Zhou
ICERM
-
Robert Zielinski
Brown University
Workshop Schedule
Monday, October 16, 2023
-
8:50 - 9:00 am EDTWelcome11th Floor Lecture Hall
- Session Chair
- Caroline Klivans, Brown University
-
9:00 - 9:45 am EDTThe geometry of perceptual spaces of textures and objects11th Floor Lecture Hall
- Speaker
- Jonathan Victor, Weill Cornell Medical College
- Session Chair
- Carina Curto, The Pennsylvania State University
Abstract
Recent technological advances allow for massive population-level recordings of neural activity, raising the hope of achieving a detailed understanding of the linkage of neurophysiology and behavior. Achieving this linkage relies on the tenet that, viewed in the right way, the mapping between neural activity and behavior preserves similarities. At the behavioral level, these similarities are captured by the topology and geometry of perceptual spaces. With this motivation, I describe some recent studies of the geometry of several perceptual spaces, including “low-level” spaces of visual features, and “higher-level” spaces dominated by semantic content. The experiments use a new, efficient psychophysical paradigm for collecting similarity judgments, and the analysis methods range from seeking Euclidean embeddings via non-metric multidimensional scaling to strategies that make minimal assumptions about the underlying geometry. With these tools, we characterize how the geometry of the spaces vary with semantic content, and the aspects of these geometries that are task-dependent.
-
10:00 - 10:15 am EDTCoffee Break11th Floor Collaborative Space
-
10:15 - 11:00 am EDTTopology protects emergent dynamics and long timescales in biological networks11th Floor Lecture Hall
- Speaker
- Evelyn Tang, Rice University
- Session Chair
- Carina Curto, The Pennsylvania State University
Abstract
Long and stable timescales are often observed in complex biochemical networks, such as in emergent oscillations or memory. How these robust dynamics persist remains unclear, given the many stochastic reactions and shorter time scales of the underlying components. We propose a topological model with parsimonious parameters that produces long oscillations around the network boundary, effectively reducing the system dynamics to a lower-dimensional current. I will demonstrate how this can model the circadian clock of cyanobacteria, with efficient properties such as simultaneously increased precision and decreased cost. Our work presents a new mechanism for emergent dynamics that could be useful for various cognitive and biological functions.
-
11:15 - 11:45 am EDTOpen Problems SessionProblem Session - 11th Floor Lecture Hall
- Session Chair
- Carina Curto, The Pennsylvania State University
-
11:45 am - 1:30 pm EDTLunch/Free Time
-
1:30 - 2:15 pm EDTDiscovering the geometry of neural representations via topological tools.11th Floor Lecture Hall
- Speaker
- Vladimir Itskov, The Pennsylvania State University
- Session Chair
- Katie Morrison, University of Northern Colorado
Abstract
Neural representations of stimulus spaces often comes with a natural geometry. Perhaps the most salient examples of such neural populations are those with convex receptive fields (or tuning curves), such as place cells in hippocampus or neurons in V1. Geometry of neural representations is understood in a very limited number of well-studied neural circuits. It is rather poorly understood in most other parts of the brain. This raises a natural question: can one infer such a geometry, based on the statistics of the neural responses alone? A crucial tool for inferring a geometry is a basis of coordinate functions that "respects" the underlying geometry, while providing meaningful low-dimensional approximations. Eigenfunctions of a Laplacian, derived from the underlying metric, serve as such basis in many scientific fields. However, spike trains, and other derived features of neural activity do not come with a natural metric, while they do come with an "intrinsic" probability distribution of neural activity patterns. Building on the tools from combinatorial topology, we introduce Hodge Laplacians associated with probability distributions on sequential data, such as spike trains. We demonstrate that these Laplacians have desirable properties with respect to the natural null-models, where the underlying neurons are independent. Our results establish a foundation for dimensionality reduction and Fourier analyses of probabilistic models, that are common in theoretical neuroscience and machine-learning.
-
2:30 - 2:40 pm EDTConnections between the topology of tasks, classifying spaces, and learned representationsLightning Talks - 11th Floor Lecture Hall
- Speaker
- Thomas Burns, ICERM
- Session Chair
- Katie Morrison, University of Northern Colorado
Abstract
Modified state complexes (Burns & Tang, 2022) extend the mathematical framework of reconfigurable systems and state complexes due to Abrams, Ghrist & Peterson to study gridworlds -- simple 2D environments inhabited of agents, objects, etc.. Such state complexes represent all possible configurations of a system as a single geometric space, thus making them conducive to study using geometric, topological, or combinatorial methods. Modified state complexes exhibit geometric defects (failure of Gromov's Link Condition) exactly where undesirable or dangerous states appear in the gridworld. We hypothesize that the modified state complex should be a classifying space for the n–strand braid group and that social place cell circuits in mammalian hippocampus use similar principles to represent and avoid danger.
-
2:40 - 2:50 pm EDTEmergence of high-order functional hubs in the human brainLightning Talks - 11th Floor Lecture Hall
- Speaker
- Fernando Nobrega Santos, University of Amsterdam
- Session Chair
- Katie Morrison, University of Northern Colorado
Abstract
Network theory is often based on pairwise relationships between nodes, which is not necessarily realistic for modeling complex systems. Importantly, it does not accurately capture non-pairwise interactions in the human brain, often considered one of the most complex systems. In this work, we develop a multivariate signal processing pipeline to build high-order networks from time series and apply it to resting-state functional magnetic resonance imaging (fMRI) signals to characterize high-order communication between brain regions. We also propose connectivity and signal processing rules for building uniform hypergraphs and argue that each multivariate interdependence metric could define weights in a hypergraph. As a proof of concept, we investigate the most relevant three-point interactions in the human brain by searching for high-order “hubs” in a cohort of 100 individuals from the Human Connectome Project. We find that, for each choice of multivariate interdependence, the high-order hubs are compatible with distinct systems in the brain. Additionally, the high-order functional brain networks exhibit simultaneous integration and segregation patterns qualitatively observable from their high-order hubs. Our work hereby introduces a promising heuristic route for hypergraph representation of brain activity and opens up exciting avenues for further research in high-order network neuroscience and complex systems.
-
2:50 - 3:00 pm EDTTopological feature selection for time series: an example with C. elegans neuronal dataLightning Talks - 11th Floor Lecture Hall
- Speaker
- Johnathan Bush, University of Florida
- Session Chair
- Katie Morrison, University of Northern Colorado
Abstract
Neurons across the brain of the model organism C. elegans are known to share information by engaging in coordinated dynamic activity that evolves cyclically. Takens' theorem implies that a sliding window embedding of time series, such as neuronal activity, will preserve the topology of an orbit of the underlying dynamical system driving the time series. These orbits are then quantifiable by the persistent homology of the sliding window embedding. In this setting, we will describe a method for topological optimization in which each time series (e.g., a single neuron's activity) is assigned a score of its contribution to the global, coordinated dynamics of a collection of time series (e.g., the brain).
-
3:00 - 3:10 pm EDTThe Directed Merge Tree Distance and its ApplicationsLightning Talks - 11th Floor Lecture Hall
- Speaker
- Xinyi Wang, Michigan State University
- Session Chair
- Katie Morrison, University of Northern Colorado
Abstract
Geometric graphs appear in many real-world datasets, such as embedded neurons, sensor networks, and molecules. We investigate the notion of distance between graphs and present a semi-metric to measure the distance between two geometric graphs via the directional transform combined with the labeled merge tree distance. We introduce a way of rotating the sub-level set to obtain the merge trees, and represent the merge trees using a surjective multi-labeling scheme. We then compute the distance between two representative matrices. Our distance is not only reflective of the information from the input graphs, but also can be computed in polynomial time. We illustrate its utility by implementation on a Passiflora leaf dataset.
-
3:10 - 3:20 pm EDTStructure Index: a graph-based method for point cloud data analysisLightning Talks - 11th Floor Lecture Hall
- Speaker
- Julio Esparza Ibanez, Instituto Cajal - CSIC (Spanish National Research Council)
- Session Chair
- Katie Morrison, University of Northern Colorado
Abstract
A point cloud is a prevalent data format found in many fields of science, which involves the definition of points in an arbitrarily high dimensional space. Typically, each of these points is associated with additional values (i.e. features) which require interpretation in the representation space. For instance, in neuroscience, neural activity over time can be pictured as a point cloud in a high-dimensional space. In these so-called neural manifolds, one may project different features onto the point cloud, such as any relevant behavioral variable. In this context, understanding if and how a given feature is structured along a point cloud can provide great insights into the neural representations. Here, I will introduce the Structure Index (SI), a graph-based metric developed to quantify how a given feature is structured along an arbitrarily high-dimensional point cloud. The SI is defined from the overlapping distribution of data points sharing similar feature values in a given neighborhood of the cloud. Using arbitrary data clouds, I will show how the SI provides quantification of the degree of local versus global organization of feature distribution. Moreover, when applied to experimental studies of head-direction cells, the SI is able to retrieve consistent feature structure from both the high- and low-dimensional representations. Overall, the SI provides versatile applications in the neuroscience and data science fields. We look to share the tool with other colleagues in the field, in order to promote community-based testing and implementation.
-
3:20 - 3:30 pm EDTStructure in neural correlations during spontaneous activity: an experimental and topological approachLightning Talks - 11th Floor Lecture Hall
- Speaker
- Nicole Sanderson, Penn State University
- Session Chair
- Katie Morrison, University of Northern Colorado
Abstract
Calcium imaging recordings of ~1000s of neurons in zebrafish larvae optic tectum in the absence of stimulation reveal spontaneous activity of neuronal assemblies that are both functionally coordinated and localized. To understand the functional structure of these assemblies, we study the pairwise correlations of the calcium signals of assembly neurons using techniques from topological data analysis (TDA). TDA can bring new insights when analyzing neural correlations, as many common techniques to do so, like spectral analyses, are sensitive to nonlinear monotonic transformations introduced in measurement. In contrast, a TDA construction called the order complex is invariant under monotonic transformations and can capture higher order structure in a set of pairwise correlations. We find that topological signatures derived from the order complex can identify distinct neural correlation structures during spontaneous activity. Our analyses further suggest a variety of possible assembly dynamics around the onset of spontaneous activation.
-
3:30 - 4:00 pm EDTCoffee Break11th Floor Collaborative Space
-
4:00 - 4:45 pm EDTTopology shapes dynamics of higher-order networks11th Floor Lecture Hall
- Speaker
- Ginestra Bianconi, Queen Mary University of London
- Session Chair
- Katie Morrison, University of Northern Colorado
Abstract
Higher-order networks capture the interactions among two or more nodes and they are raising increasing interest in the study of brain networks. Here we show that higher-order interactions are responsible for new non-linear dynamical processes that cannot be observed in pairwise networks. We reveal how non-linear topolody shapes dynamics, by defining Topological Kuramoto model and Topological global synchronization. These critical phenomena capture the synchronization of topological signals, i.e. dynamical signal defined not only on nodes but also on links, triangles and higher-dimensional simplices in simplicial complexes. In this novel synchronized states for topological signals the dynamics localizes on the holes of the simplicial complexes. Moreover will discuss how the Dirac operator can be used to couple and process topological signals of different dimensions, formulating Dirac signal processing. Finally we will show how non-linear dynamics can shape topology by formulating triadic percolation. In triadic percolation triadic interactions can turn percolation into a fully-fledged dynamical process in which nodes can turn on and off intermittently in a periodic fashion or even chaotically leading to period doubling and a route to chaos of the percolation order parameter. Triadic percolation changes drastically our understanding of percolation and can describe real systems in which the giant component varies significantly in time such as in brain functional networks and in climate.
-
5:00 - 6:30 pm EDTReception11th Floor Collaborative Space
Tuesday, October 17, 2023
-
9:00 - 9:45 am EDTA power law of cortical adaptation in neural populations11th Floor Lecture Hall
- Speaker
- Dario Ringach, University of California, Los Angeles
- Session Chair
- Matilde Marcolli, California Institute of Technology
Abstract
How do neural populations adapt to the time-varying statistics of sensory input? To investigate, we measured the activity of neurons in primary visual cortex adapted to different environments, each associated with a distinct probability distribution over a stimulus set. Within each environment, a stimulus sequence was generated by independently sampling form its distribution. We find that two properties of adaptation capture how the population responses to a given stimulus, viewed as vectors, are linked across environments. First, the ratio between the response magnitudes is a power law of the ratio between the stimulus probabilities. Second, the response directions are largely invariant. These rules can be used to predict how cortical populations adapt to novel, sensory environments. Finally, we show how the power law enables the cortex to signal unexpected stimuli preferentially and to adjust the metabolic cost of its sensory representation to the entropy of the environment.
-
10:00 - 10:15 am EDTCoffee Break11th Floor Collaborative Space
-
10:15 - 11:00 am EDTA geometric model of the visual and motor cortex11th Floor Lecture Hall
- Speaker
- Giovanna Citti, university of Bologna
- Session Chair
- Matilde Marcolli, California Institute of Technology
Abstract
I'll present a geometric model of the motor cortex, joint work with Alessandro Sarti. Each family of cells in the cortex is sensitive to a specific feature and will be described as a sub-Riemannian space. The propagation of the activity along cortical connectivity will be described as a sub-Riemannian differential equation. The stable states of the equation will describe the perceptual units, allowing to validate the model. It can be applied to selectivity of simple features (as for example direction of movement), or to more complex feautures, defined as perceptual units of the previous family of cells. The same instruments can describe both the visual and the motor cortex.
-
11:15 - 11:45 am EDTOpen Problems SessionProblem Session - 11th Floor Lecture Hall
- Session Chair
- Matilde Marcolli, California Institute of Technology
-
11:50 am - 12:00 pm EDTGroup Photo (Immediately After Talk)11th Floor Lecture Hall
-
12:00 - 1:30 pm EDTNetworking LunchWorking Lunch - 11th Floor Lecture Hall
-
1:30 - 2:15 pm EDTTopological analysis of sensory-evoked network activity11th Floor Lecture Hall
- Speaker
- Alex Reyes, New York University
- Session Chair
- Peter Thomas, Case Western Reserve University
Abstract
Sensory stimuli evoke activity in a population of neurons in cortex. In topographically organized networks, activated neurons with similar receptive fields occur within a relatively confined area, suggesting that the spatial distribution and firing dynamics of the neuron population contribute to processing of sensory information. However, inherent variability in neuronal firing, makes it difficult to determine which neurons encode signal and which represent noise. Here, we use simplicial complexes to identify functionally relevant neurons whose activities are likely to be propagated and to distinguish between multiple populations activated during complex stimuli. Moreover, preliminary analyses suggest that changes in the extent and magnitude of network activity can be described abstractly as the movement of points on the surface of a torus.
-
2:30 - 2:40 pm EDTAnalyzing spatiotemporal patterns using geometric scattering and persistent homologyLightning Talks - 11th Floor Lecture Hall
- Speaker
- Dhananjay Bhaskar, Yale University
- Session Chair
- Peter Thomas, Case Western Reserve University
Abstract
I will introduce Geometric Scattering Trajectory Homology (GSTH), a general framework for analyzing complex spatiotemporal patterns that emerge from coordinated signaling and communication in a variety of biological contexts, including Ca2+ activity in the prefrontal visual cortex in response to grating stimuli, and entrainment of theta oscillations in the brain during memory encoding and retrieval tasks. We tested this framework by recovering model parameters, drug treatments and stimuli from simulation and experimental data. Additionally, we show that learned representations in GSTH capture the degree of synchrony, phase transitions, and quasi-periodicity of the underlying signaling pattern at multiple scales, showing promise towards uncovering intricate neural communication mechanisms.
-
2:40 - 2:50 pm EDTMultiple Neural Spike Train Data Analysis Using Persistent HomologyLightning Talks - 11th Floor Lecture Hall
- Speaker
- Huseyin Ayhan, Florida State University
- Session Chair
- Peter Thomas, Case Western Reserve University
Abstract
A neuronal spike train is the recorded sequence of times when a neuron fires action potentials, also known as spikes. Studying the collective activities of neurons as a network of spike trains can help us gain an understanding of how they function. These networks are well-suited for the application of topological tools. In this lightning talk, I will briefly explain how persistent homology, one of the most powerful tools of TDA, can be applied to understand and compare the topology of these networks.
-
2:50 - 3:00 pm EDTVariability of topological features on brain functional networks in precision resting-state fMRI.Lightning Talks - 11th Floor Lecture Hall
- Speaker
- Juan Carlos Díaz-Patiño, Universidad Nacional Autónoma de México
- Session Chair
- Peter Thomas, Case Western Reserve University
Abstract
Nowadays, much scientific literature discusses Topological Data Analysis (TDA) applications in Neuroscience. Nevertheless, a fundamental question in the field is, how different are fMRI in one individual over a short time? Are they similar? What are the changes between individuals? This talk presents the approach used to study resting-state functional Magnetic Resonance Images (fMRI) with TDA methods using the Vietoris-Rips filtration over a weighted network and looking for statistical differences between their Betti Curves and also a vectorization method using the Minimum Spanning Tree.
-
3:00 - 3:10 pm EDTGabor Frames and Contact structures: Signal encoding and decoding in the primary visual cortexLightning Talks - 11th Floor Lecture Hall
- Speaker
- Vasiliki Liontou, ICERM
- Session Chair
- Peter Thomas, Case Western Reserve University
Abstract
Contact structures and Gabor functions have been used, independently, to model the activity of the mammalian primary visual cortex. Gabor functions are also used in signal analysis and in particular in signal encoding and decoding. In particular, a one-dimensional signal, an $L^2$ function of one variable , can be represented in two dimensions, with time and frequency as coordinates. The signal is expanded into a series of Gabor functions (an analog of a Fourier basis), which are constructed from a single seed function by applying time and frequency translations. This talk summarizes the construction of a framework of signal analysis on models of $V_1$, determined by its contact structure and suggests a mathematical model of $V_1$ which allows the encoding and decoding of a signal by a discrete family of orientation and position dependent receptive profiles.
-
3:10 - 3:20 pm EDTHarmonic Analysis of SequencesLightning Talks - 11th Floor Lecture Hall
- Speaker
- Hannah Santa Cruz, Penn State
- Session Chair
- Peter Thomas, Case Western Reserve University
Abstract
The Combinatorial Laplacian is a popular tool in Graph and Network analysis. Recent work has proposed the use of Hodge Laplacians and the Magnetic Laplacian to analyze Simplicial Complexes and Directed Graphs respectively. We continue this work, by interpreting the Hodge Laplacian associated to a weighed simplicial complex, in terms of a weight function which is induced by a probability distribution. In particular, we develop a null hypothesis weighed simplicial complex model, induced by an independent distribution on the vertices, and show that the associated Laplacian is trivial. We extend this work to Sequence Complexes, where we consider the faces to be sequences, allowing for repeated vertices and distinguishing sequences with different orderings. In this setting, we also explore the Laplacian associated to a weight function induced by an independent distribution on the vertices, and completely describe it’s eigen spectrum, which is no longer trivial but still simple. Our analysis and findings contribute to the broader field of spectral graph theory and provide a deeper understanding of Laplacians on simplicial and sequence complexes, paving the way for further exploration and applications of Laplacian operators.
-
3:20 - 3:30 pm EDTGroup symmetry: a designing principle of recurrent neural circuits in the brainLightning Talks - 11th Floor Lecture Hall
- Speaker
- Wenhao Zhang, UT Southwestern Medical Center
- Session Chair
- Peter Thomas, Case Western Reserve University
Abstract
Equivariant representation is necessary for the brain and artificial perceptual systems to faithfully represent the stimulus under some (Lie) group transformations. However, it remains unknown how recurrent neural circuits in the brain represent the stimulus equivariantly, nor the neural representation of abstract group operators. In this talk, I will present my recent attempts to narrow down this gap. We recently used the one-dimensional translation group and the temporal scaling group as examples to explore the general recurrent neural circuit mechanism of the equivariant stimulus representation. We found that a continuous attractor network (CAN), a canonical neural circuit model, self-consistently generates a continuous family of stationary population responses (attractors) that represents the stimulus equivariantly. We rigorously derived the representation of group operators in the circuit dynamics. The derived circuits are comparable with concrete neural circuits discovered in the brain and can reproduce neuronal responses that are consistent with experimental data. Our model for the first time analytically demonstrates how recurrent neural circuitry in the brain achieves equivariant stimulus representation.
-
3:30 - 4:00 pm EDTCoffee Break11th Floor Collaborative Space
-
4:00 - 4:45 pm EDTA Neuron as a Direct Data-Driven Controller11th Floor Lecture Hall
- Speaker
- Dmitri Chklovskii, Flatiron Institute & NYU Neuroscience Institute
- Session Chair
- Peter Thomas, Case Western Reserve University
Abstract
"Efficient coding theories have elucidated the properties of neurons engaged in early sensory processing. However, their applicability to downstream brain areas, whose activity is strongly correlated with behavior, remains limited. Here we present an alternative viewpoint, casting neurons as feedback controllers in closed loops comprising fellow neurons and the external environment. Leveraging the novel Direct Data-Driven Control (DD-DC) framework, we model neurons as biologically plausible controllers which implicitly identify loop dynamics, infer latent states and optimize control. Our DD-DC neuron model accounts for multiple neurophysiological observations, including the transition from potentiation to depression in Spike-Timing-Dependent Plasticity (STDP) with its asymmetry, the temporal extent of feed-forward and feedback neuronal filters and their adaptation to input statistics, imprecision of the neuronal spike-generation mechanism under constant input, and the prevalence of operational variability and noise in the brain. The DD-DC neuron contrasts with the conventional, feedforward, instantaneously responding McCulloch-Pitts-Rosenblatt unit, thus offering an alternative foundational building block for the construction of biologically-inspired neural networks.
Wednesday, October 18, 2023
-
9:00 - 9:45 am EDTObject representation in the brain11th Floor Lecture Hall
- Speaker
- Dmitry Rinberg, New York University
- Session Chair
- Tatyana Sharpee, Salk Institute
Abstract
Animals can recognize sensory objects that are relevant to their behavior, such as familiar sounds, faces, or the smell of specific fruits. This ability relies on the sensory system performing two key computational tasks: first, distinguishing a particular object from all other objects, and second, generalizing across some range of stimuli. The latter implies that objects have some range of variability in the stimulus space - a smell of an apple may be attributed to multiple different apple varieties with similar chemical composition. Additionally, as the presented stimuli become more different from what's expected or familiar, the ability to correctly identify them decreases. Such computational requirements set up constrains for the geometry of the neural space of object representation in the brain. In this presentation, I will delve into our efforts to investigate object representation in the brain, employing optogenetic pattern stimulation of the peripheral olfactory system to create highly controllable synthetic odor stimuli. We have developed a behavioral paradigm that enables us to address both essential computational prerequisites: discriminating between and generalizing across stimuli. Furthermore, we have quantified both behavioral responses and neural activity. Our findings have revealed that the neural space governing stimulus responses conforms closely to the criteria for effective object representation, closely mirroring behavioral outcomes.
-
10:00 - 10:15 am EDTCoffee Break11th Floor Collaborative Space
-
10:15 - 11:00 am EDTThe developmental timeline of the grid cell torus and how we are studying it11th Floor Lecture Hall
- Speaker
- Benjamin Dunn, Norwegian University of Science and Technology
- Session Chair
- Tatyana Sharpee, Salk Institute
-
11:15 - 11:45 am EDTOpen Problems SessionsProblem Session - 11th Floor Lecture Hall
- Session Chair
- Tatyana Sharpee, Salk Institute
-
12:00 - 1:30 pm EDTLunch/Free Time
-
1:45 - 2:30 pm EDTInformational and topological signatures of individuality and age11th Floor Lecture Hall
- Speaker
- Giovanni Petri, CENTAI Institute
- Session Chair
- Tatyana Sharpee, Salk Institute
Abstract
Network neuroscience is a dominant paradigm for understanding brain function.Functional Connectivity (FC) encodes neuroimaging signals in terms of the pairwise correlation patterns of coactivations between brain regions. However, FC is by construction limited to such pairwise relations. In this seminar, we explore functional activations as a topological space via tools from topological data analysis. In particular, we analyze the resting fMRI data of populations of healthy subjects across ages, and demonstrate that algebraic-topological features extracted from brain activity are effective for brain fingerprinting. By computing persistent homology and constructing topological scaffolds, we show that these features outperform FC in discriminating between individuals and ages. That is, the topological structures are more similar for the same individual across different recording sessions than across individuals. Similarly, we find that topological observables improve discrimination of individuals of different ages. Finally, we show that the regions highlighted by our topological methods are characterized by characteristic patterns of information redundancy and synergy which are not share by regions that are topologically unimportant, hence establishing a first direct link between topology and information theory in neuroscience.
-
2:45 - 2:55 pm EDTEphemeral Persistence Features and the Stability of Filtered Chain ComplexesLightning Talks - 11th Floor Lecture Hall
- Speaker
- Ling Zhou, ICERM
- Session Chair
- Tatyana Sharpee, Salk Institute
Abstract
We strengthen the usual stability theorem for Vietoris-Rips persistent homology of finite metric spaces by building upon constructions due to Usher and Zhang in the context of filtered chain complexes. The information present at the level of filtered chain complexes includes ephemeral points, i.e. points with zero persistence, which provide additional information to that present at homology level. The resulting invariant, called verbose barcode, which has a stronger discriminating power than the usual barcode, is proved to be stable under certain metrics which are sensitive to these ephemeral points. In the case of degree zero, we provide an explicit formula to compute this new metric between verbose barcodes.
-
2:55 - 3:05 pm EDTHomotopy and singular homology groups of finite graphsLightning Talks - 11th Floor Lecture Hall
- Speaker
- Nikola Milicevic, Pennsylvania State University
- Session Chair
- Tatyana Sharpee, Salk Institute
Abstract
We verify analogues of classical results for higher homotopy groups and singular homology groups of (Cech) closure spaces. Closure spaces are a generalization of topological spaces that also include graphs and directed graphs and are thus a bridge that connects classical algebraic topology with the more applied side of topology, such as digital topology. More specifically, we show the existence of a long exact sequence for homotopy groups of pairs of closure spaces and that a weak homotopy equivalence induces isomorphisms for homology groups. Our main result is the construction of a weak homotopy equivalences between the geometric realizations of (directed) clique complexes and their underlying (directed) graphs. This implies that singular homology groups of finite graphs can be efficiently calculated from finite combinatorial structures, despite their associated chain groups being infinite dimensional. This work is similar to the work McCord did for finite topological spaces, but in the context of closure spaces. Our results also give a novel approach for studying (higher) homotopy groups of discrete mathematical structures such as digital images.
-
3:05 - 3:15 pm EDTHebbian learning of cyclic structures of neural codeLightning Talks - 11th Floor Lecture Hall
- Speaker
- Nikolas Schonsheck, University of Delaware
- Session Chair
- Tatyana Sharpee, Salk Institute
Abstract
Cyclic structures are a class of mesoscale features ubiquitous in both experimental stimuli and the activity of neural populations encoding them. Important examples include encoding of head direction, grid cells in spatial navigation, and orientation tuning in visual cortex. The central question of this short talk is: how does the brain faithfully transmit cyclic structures between regions? Is this a generic feature of neural circuits, or must this be learned? If so, how? While cyclic structures are difficult to detect and analyze with classical methods, tools from algebraic topology have proven to be particularly effective in understanding cyclic structures. Recently, work of Yoon et al. develops a topological framework to match cyclic coding patterns in distinct populations that encode the same information. We leverage this framework to show that, beginning with a random initialization, Hebbian learning robustly supports the propagation of cyclic structures through feedforward networks. This is joint work with Chad Giusti.
-
3:15 - 3:25 pm EDTThe bifiltration of a relation and unsupervised inference of neural representationsLightning Talks - 11th Floor Lecture Hall
- Speaker
- Melvin Vaupel, Norwegian Institute of Science and Technology
- Session Chair
- Tatyana Sharpee, Salk Institute
Abstract
To neural activity one may associate a space of correlations and a space of population vectors. These can provide complementary information. Assume the goal is to infer properties of a covariate space, represented by the recorded neurons. Then the correlation space is better suited if multiple neural modules are present, while the population vector space is preferable if neurons have non-convex receptive fields. In this talk I will explain how to coherently combine both pieces of information in a bifiltration using Dowker complexes and their total weight filtrations.
-
3:30 - 4:00 pm EDTCoffee Break11th Floor Collaborative Space
-
4:00 - 4:45 pm EDTAn application of neighbourhoods in directed graphs in the classification of binary dynamics11th Floor Lecture Hall
- Speaker
- Ran Levi, University of Aberdeen
- Session Chair
- Tatyana Sharpee, Salk Institute
Abstract
A binary state on a graph means an assignment of binary values to its vertices. For example, if one encodes a network of spiking neurons as a directed graph, then the spikes produced by the neurons at an instant of time is a binary state on the encoding graph. Allowing time to vary and recording the spiking patterns of the neurons in the network produces an example of a binary dynamics on the encoding graph, namely a one-parameter family of binary states on it. The central object of study in this talk is the neighbourhood of a vertex v in a graph G, namely the subgraph of G that is generated by v and all its direct neighbours in G. We present a topological/graph theoretic method for extracting information out of binary dynamics on a graph, based on a selection of a relatively small number of vertices and their neighbourhoods. As a test case we demonstrate an application of the method to binary dynamics that arises from sample activity on the Blue Brain Project reconstruction of cortical tissue of a rat.
Thursday, October 19, 2023
-
9:00 - 9:45 am EDTHow to simulate a connectome?11th Floor Lecture Hall
- Speaker
- Srinivas Turaga, HHMI - Janelia Research Campus
- Session Chair
- Carina Curto, The Pennsylvania State University
Abstract
We can now measure the connectivity of every neuron in a neural circuit, but we are still blind to other biological details, including the dynamical characteristics of each neuron. The degree to which connectivity measurements alone can inform understanding of neural computation is an open question. We show that with only measurements of the connectivity of a biological neural network, we can predict the neural activity underlying neural computation. Our mechanistic model makes detailed experimentally testable predictions for each neuron in the connectome. We found that model predictions agreed with experimental measurements of neural activity across 24 studies. Our work demonstrates a strategy for generating detailed hypotheses about the mechanisms of neural circuit function from connectivity measurements. https://www.biorxiv.org/content/10.1101/2023.03.11.532232
-
10:00 - 10:15 am EDTCoffee Break11th Floor Collaborative Space
-
10:15 - 11:00 am EDTFrom single neurons to complex networks using algebraic topology11th Floor Lecture Hall
- Speaker
- Lida Kanari, EPFL/Blue Brain
- Session Chair
- Carina Curto, The Pennsylvania State University
Abstract
Topological Data Analysis has been successfully used in a variety of applications including protein study, cancer detection, and study of porous materials. Based on algebraic topology, we created a robust topological descriptor of neuronal morphologies and used it to classify and cluster neurons and microglia. But what can topology tell us about the functional roles of neurons in the brain? In this talk, I will focus on focus on the study of the human brain, delving deeper into the fundamental question of neuroscience “whether dendritic structures hold the key to enhanced cognitive abilities”. Starting from the topological differences of mouse and human neurons, we create artificial networks for both species. We show that topological complexity leads to highly inter-connected pyramidal-to-pyramidal and higher-order networks, which is unexpected in view of reduced neuronal density in humans compared to the mouse neocortex. We thus present robust evidence that increased topological complexity in human neurons ultimately leads to highly interconnected cortical networks despite reduced neuronal density. https://www.biorxiv.org/content/10.1101/2023.09.11.557170v1
-
11:30 am - 1:30 pm EDTOpen Problems LunchWorking Lunch
-
1:30 - 2:15 pm EDTRapid emergence of latent knowledge in the sensory cortex drives learning11th Floor Lecture Hall
- Speaker
- Kishore Kuchibhotla, Johns Hopkins University
- Session Chair
- Horacio Rotstein, New Jersey Institute of Technology
Abstract
Large-scale neural recordings provide an opportunity to better understand how the brain implements critical behavioral computations related to goal-directed learning. Here, I will argue that re-visiting our understanding of the shape of the learning curve and its underlying cognitive drivers is essential for uncovering its neural basis. Rather than thinking about learning as either ‘slow’ or ‘sudden’, I will argue that learning is better interpreted as a combination of the two. I will provide behavioral evidence that goal-directed learning can be dissociated into two parallel processes: knowledge acquisition which is rapid with step-like improvements and behavioral expression, which is slower and more variable, with animals exhibiting rudimentary forms of hypothesis testing. This behavioral approach has allowed us to isolate the associative (knowledge-related) and non-associative (performance-related) components that influence learning. I will present probabilistic optogenetic and longitudinal two-photon imaging results that neural dynamics in the auditory cortex are crucial for auditory guided, goal-directed learning. Conjoint representations of sensory and non-sensory variables in the same auditory cortical network evolve in a structured and dynamic manner, actively integrating multimodal signals via dissociable neural ensembles. Our data suggest that the sensory cortex is an associative engine with the cortical network shifting from being largely stimulus-driven to one that is optimized for behavioral needs.
-
2:30 - 3:15 pm EDTMargin learning in spiking neurons11th Floor Lecture Hall
- Speaker
- Robert Gütig, Charité Medical School Berlin
- Session Chair
- Horacio Rotstein, New Jersey Institute of Technology
Abstract
Learning novel sensory features from few examples is a remarkable ability of humans and other animals. For example, we can recognize unfamiliar faces or words after seeing or hearing them only a few times, even in different contexts and noise levels. Previous work has shown that spiking neural networks can learn to detect unknown features in unsegmented input streams using multi-spike tempotron learning. However, this method requires many training patterns and the learned solutions can be sensitive to noise. In this work, we use multi-spike tempotron learning to implement margin learning in spiking neurons. Specifically, we introduce regularization terms that enable leaky-integrate-and-fire neurons to learn to detect recurring features using orders of magnitude less training data and converge to robust solutions. We test the novel learning rule on unsegmented spoken digit sequences contained in the TIDIGITS speech data set and find a twofold improvement in detection probability over the original learning algorithm. Our work shows how neurons can learn to detect embedded features from a limited number of unsegmented samples, provides fundamental bounds for the noise robustness of the leaky integrate-and-fire model and ties mathematically principled gradient-based optimization to biologically plausible learning in spiking neurons.
-
3:30 - 4:00 pm EDTCoffee Break11th Floor Collaborative Space
-
4:00 - 4:45 pm EDTMetastable dynamics in cortical circuits11th Floor Lecture Hall
- Speaker
- Giancarlo La Camera, Stony Brook University
- Session Chair
- Horacio Rotstein, New Jersey Institute of Technology
Abstract
I will discuss recent results on metastable dynamics in cortical circuits, characterized by seemingly random switching among a finite number of discrete states. Single states and their metastable dynamics can reflect abstract features of external stimuli as well as internal deliberations, and have been proposed as supporting a role in a variety of functions including sensory coding, expectation, decision making and behavioral accuracy. Many results in this context have been captured by spiking network models with a clustered architecture. I will review data and models while trying to provide a model-inspired unitary view of the phenomena discussed. If time permits, I will present a model of how this type of dynamics can emerge from (and coexist with) experience-dependent plasticity in a network of spiking neurons.
Friday, October 20, 2023
-
9:00 - 9:45 am EDTLearning topological structure in neural population codes11th Floor Lecture Hall
- Speaker
- Chad Giusti, Oregon State University
- Session Chair
- Vladimir Itskov, The Pennsylvania State University
Abstract
The stimulus space model for neural population activity describes the activity of individual neurons as points localized in a metric stimulus space, with firing rate falling off with distance to individual stimuli. We will briefly review this model, and discuss how methods from topological data analysis allow us to extract qualitative structure and coordinate systems for such spaces from measures of neural population activity. We will briefly explore challenges that arise when studying whether and how multiple neural populations encode the same topological structure, and discuss recent experiments involving Hebbian learning for circular coordinate systems in feed-forward networks. No prior knowledge of topological methods will be assumed.
-
10:00 - 10:45 am EDTTopological tracing of encoded circular coordinates between neural populations11th Floor Lecture Hall
- Speaker
- Iris Yoon, Wesleyan University
- Session Chair
- Vladimir Itskov, The Pennsylvania State University
Abstract
Recent developments in in vivo neuroimaging in animal models have made possible the study of information coding in large populations of neurons and even how that coding evolves in different neural systems. Topological methods, in particular, are effective at detecting periodic, quasi-periodic, or circular features in neural systems. Once we detect the presence of circular structures, we face the problem of assigning semantics: what do the circular structures in a neural population encode? Are they reflections of an underlying physiological activity, or are they driven by an external stimulus? If so, which specific features of the stimulus are encoded by the neurons? To address this problem, we introduced the method of analogous bars (Yoon, Ghrist, Giusti 2023). Given two related systems, say a stimulus system and a neural population, or two related neural populations, we utilize the dissimilarity between the two systems and Dowker complexes to find shared features between the two systems. We then leverage this information to identify related features between the two systems. In this talk, I will briefly explain the mathematics underlying the analogous bars method. I will then present applications of the method in studying neural population coding and propagation on simulated and experimental datasets. This work is joint work with Gregory Henselman-Petrusek, Lori Ziegelmeier, Robert Ghrist, Spencer Smith, Yiyi Yu, and Chad Giusti.
-
11:00 - 11:30 am EDTCoffee Break11th Floor Collaborative Space
-
11:30 am - 12:15 pm EDTThe neurogeometry of the visual cortex11th Floor Lecture Hall
- Speaker
- Alessandro Sarti, National Center of Scientific Research, EHESS, Paris
- Session Chair
- Vladimir Itskov, The Pennsylvania State University
Abstract
I will consider a model of the primary visual cortex in terms of Lie groups equipped with a sub-Riemannian metric. The shape of receptive profiles as well as the patterns of short range and long range connectivity will have a precise geometric meaning. After showing examples of contour completion in the sub-Riemannian structure, I will consider the coupling of heterogeneous cells to model amodal completion (Kanitza triangle) as well as contrast-costancy image reconstruction in V1. The reconstruction involves a new type of Poisson problem with heterogeneous differential operators. (Joint work with Giovanna Citti)
-
12:30 - 2:00 pm EDTLunch/Free Time
-
2:00 - 2:45 pm EDTFinal Open Problems SessionProblem Session - 11th Floor Lecture Hall
- Session Chair
- Carina Curto, The Pennsylvania State University
-
3:30 - 4:00 pm EDTCoffee Break11th Floor Collaborative Space
All event times are listed in ICERM local time in Providence, RI (Eastern Daylight Time / UTC-4).
All event times are listed in .
ICERM local time in Providence, RI is Eastern Daylight Time (UTC-4). Would you like to switch back to ICERM time or choose a different custom timezone?
Request Reimbursement
This section is for general purposes only and does not indicate that all attendees receive funding. Please refer to your personalized invitation to review your offer.
- ORCID iD
- As this program is funded by the National Science Foundation (NSF), ICERM is required to collect your ORCID iD if you are receiving funding to attend this program. Be sure to add your ORCID iD to your Cube profile as soon as possible to avoid delaying your reimbursement.
- Acceptable Costs
-
- 1 roundtrip between your home institute and ICERM
- Flights on U.S. or E.U. airlines – economy class to either Providence airport (PVD) or Boston airport (BOS)
- Ground Transportation to and from airports and ICERM.
- Unacceptable Costs
-
- Flights on non-U.S. or non-E.U. airlines
- Flights on U.K. airlines
- Seats in economy plus, business class, or first class
- Change ticket fees of any kind
- Multi-use bus passes
- Meals or incidentals
- Advance Approval Required
-
- Personal car travel to ICERM from outside New England
- Multiple-destination plane ticket; does not include layovers to reach ICERM
- Arriving or departing from ICERM more than a day before or day after the program
- Multiple trips to ICERM
- Rental car to/from ICERM
- Flights on a Swiss, Japanese, or Australian airlines
- Arriving or departing from airport other than PVD/BOS or home institution's local airport
- 2 one-way plane tickets to create a roundtrip (often purchased from Expedia, Orbitz, etc.)
- Travel Maximum Contributions
-
- New England: $350
- Other contiguous US: $850
- Asia & Oceania: $2,000
- All other locations: $1,500
- Note these rates were updated in Spring 2023 and superseded any prior invitation rates. Any invitations without travel support will still not receive travel support.
- Reimbursement Requests
-
Request Reimbursement with Cube
Refer to the back of your ID badge for more information. Checklists are available at the front desk and in the Reimbursement section of Cube.
- Reimbursement Tips
-
- Scanned original receipts are required for all expenses
- Airfare receipt must show full itinerary and payment
- ICERM does not offer per diem or meal reimbursement
- Allowable mileage is reimbursed at prevailing IRS Business Rate and trip documented via pdf of Google Maps result
- Keep all documentation until you receive your reimbursement!
- Reimbursement Timing
-
6 - 8 weeks after all documentation is sent to ICERM. All reimbursement requests are reviewed by numerous central offices at Brown who may request additional documentation.
- Reimbursement Deadline
-
Submissions must be received within 30 days of ICERM departure to avoid applicable taxes. Submissions after thirty days will incur applicable taxes. No submissions are accepted more than six months after the program end.