Organizing Committee
- Ronen Basri
Weizmann Institute of Science - Alex Berg
University of North Carolina, Chapel Hill - David Jacobs
University of Maryland
Abstract
Recent advances in machine learning have had a profound impact on computer vision. Simultaneously, success in computer vision applications has rapidly increased our understanding of some machine learning techniques, especially their applicability. This workshop will bring together researchers who are building a stronger theoretical understanding of the foundations of machine learning with computer vision researchers who are advancing our understanding of machine learning in practice.
Much of the recent growth in the use of machine learning in computer vision has been spurred by advances in deep neural networks. At the same time, new advances in other areas of machine learning, including reinforcement learning, generative models, and optimization methods, hold great promise for future impact. These raise important fundamental questions, such as understanding what influences the ability of learning algorithms to generalize, understanding what causes optimization in learning to converge to effective solutions, and understanding how to make optimization more efficient.
The workshop will include machine learning researchers who are addressing these foundational questions. It will also include computer vision researchers who are applying machine learning to a host of problems, such as visual categorization, 3D reconstruction, event and activity understanding, and semantic segmentation.
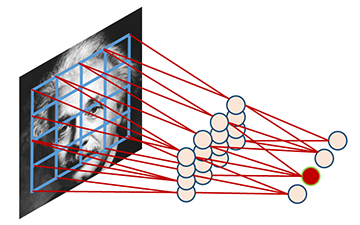
Confirmed Speakers & Participants
Talks will be presented virtually or in-person as indicated in the schedule below.
- Speaker
- Poster Presenter
- Attendee
- Virtual Attendee
-
Ben Abbatematteo
Brown University
-
Manuchehr Aminian
Colorado State University
-
Yali Amit
University of Chicago
-
Saed Asaly
Ariel University
-
Yuval Bahat
Technion - Israel Institute of Technology
-
Richard Baraniuk
Rice University
-
Alessandro Barone
Emory University
-
Theresa Barton
Brown University
-
Ronen Basri
Weizmann Institute of Science
-
Muzammil Behzad
University of Oulu
-
mouhacine Benosman
MERL
-
Alex Berg
University of North Carolina, Chapel Hill
-
Yuri Boykov
University of Waterloo
-
Nathan Cahill
Rochester Institute of Technology
-
Jorio Cocola
Rice University
-
Nadav Cohen
Institute for Advanced Study
-
Eva Comino
University of Souhern Mississippi
-
Matt Corsaro
Brown University
-
Joseph Daws
University of Tennessee, Knoxville
-
Tali Dekel
Google
-
Eren Derman
Brown University
-
Deshana Desai
New York University
-
Keiko Dow
D'Youville College
-
Hasan Eruslu
University of Delaware
-
Zhou Fang
Brown University
-
Jeová Farias Sales Rocha Neto
Brown university
-
Pedro Felzenszwalb
Brown University
-
Rob Fergus
Courant Institute of Mathematical Sciences, New York University
-
Katerina Fragkiadaki
Carnegie Mellon
-
Tingran Gao
The University of Chicago
-
Davi Geiger
Courant Institute, NYU
-
Stuart Geman
Brown University
-
Basilis Gidas
Brown University
-
Tom Goldstein
University of Maryland
-
Ming Gong
University of Dayton
-
Nakul Gopalan
Brown University
-
Anna Grim
Brown University
-
Sofia Guzzetti
Emory University
-
Benjamin Haeffele
Johns Hopkins University
-
Shermin Hamzehei
University of Massachusetts, Amherst
-
Tal Hassner
Open University of Israel
-
Kathryn Heal
Harvard University
-
Fumi Honda
Brown University
-
Furong Huang
University of Maryland
-
Shao Ran Huang
Brown University
-
Zhishen Huang
University of Colorado Boulder
-
Sorin Istrail
Brown University
-
David Jacobs
University of Maryland
-
Numair Khan
Brown University
-
Benjamin Kimia
Brown University
-
Kathlén Kohn
ICERM
-
Shahar Kovalsky
Duke University
-
Shira Kritchman
The Weizmann Institute of Science
-
Henry Kvinge
Colorado State University
-
Svetlana Lazebnik
University of Illinois, Urbana-Champaign
-
Triet Le
The National Geospatial-Intelligence Agency
-
Taehee Lee
Brown University
-
Han Cheng Lee
Brown University
-
Florencia Leonardi
University of Sao Paulo
-
Simin Li
Tsinghua University
-
Drew Linsley
Brown University
-
Karen Livescu
Toyota Technological Institute at Chicago
-
Ziyin Ma
Brown University
-
Subhransu Maji
University of Massachusetts, Amherst
-
James Martens
Google DeepMind
-
Michael Patrick Martin
Johns Hopkins University
-
Jacob McCord
National Geospatial-Intelligence Agency
-
F. Patricia Medina
Worcester Polytechnic Institute
-
Sara Motlaghian
Georgia State University
-
Reshma Munbodh
Alpert Medical School of Brown University
-
Michael Northington
Georgia Institute of Technology
-
Tomas Pajdla
Czech Technical University in Prague
-
Ambar Pal
Johns Hopkins University
-
Mario Parente
University of Massachusetts Amherst
-
Andrew Peterson
Brown University
-
Armenak Petrosyan
Oak Ridge National Laboratory
-
Dimitris Pinotsis
University of London — City and MIT
-
Farhad Pourkamali Anaraki
University of Massachusetts Lowell
-
Qing Qu
New York University
-
Viktor Reshniak
Oak Ridge National Laboratory
-
Kate Saenko
Boston University
-
Ali Salehi
University of Memphis
-
Thomas Serre
Brown University
-
Hanieh Shabanian
University of Memphis
-
Mahdi Soltanolkotabi
University of Southern California
-
Ömer Sümer
University of Tubingen
-
Amara Tariq
Forman Christian College (A Chartered University)
-
Gabriel Taubin
Brown University
-
Matus Telgarsky
University of Illinois Urbana-Champaign
-
Naftali Tishby
Hebrew University
-
James Tompkin
Brown University
-
Shubhendu Trivedi
ICERM
-
Eli Upfal
Brown University
-
Marilyn Vazquez Landrove
ICERM
-
Olga Veksler
University of Waterloo
-
Rene Vidal
Johns Hopkins University
-
Ying Wang
University of Oklahoma
-
Haixu Wang
Florida State University
-
Daphna Weinshall
Hebrew University of Jerusalem
-
Tomer Weiss
Wayfair
-
Buren Wells
University of Alabama in Huntsville
-
Neda Yazdianpour
Georgia state university
-
Rujie Yin
Verily
-
Rich Zemel
University of Toronto
-
Jian-Zhou Zhang
Sichuan University
-
Zhengwu Zhang
University of Rochester
-
Fan Zhang
Harvard Medical School
-
Guangyao Zhou
Brown University
-
Hao Zhou
University of Maryland
-
Lingjiong Zhu
Florida State University