Organizing Committee
- Lexin Li
University of California, Berkeley - Youssef Marzouk
Massachusetts Institute of Technology - Shari Moskow
Drexel University - Benjamin Peherstorfer
Courant Institute, New York University - Abel Rodriguez
University of California, Santa Cruz - Daniele Venturi
University of California, Santa Cruz - Rachel Ward
University of Texas at Austin
Abstract
The advancement in computing and storage capabilities of modern computational clusters fosters use of novel statistical techniques in machine learning and deep networks. Such data-driven techniques allow one to learn model features and characteristics that are difficult for mathematical methods alone to reveal. Many computational methods achieve model and complexity discovery using methods that lie at the nexus of mathematical, statistical, and computational disciplines. Statistical methods often employ “big data” approaches that glean predictive capability from diverse and enormous databases of information. Emerging methods in machine learning and deep networks can provide impressive results. This workshop gathers researchers at the frontier of large-scale statistical computation, data science, tensor decompositions and approximations, and data-driven model learning, to focus on modern challenges that use data to reduce complexity of models.
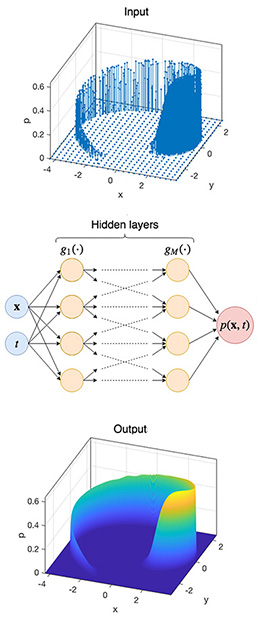
Confirmed Speakers & Participants
Talks will be presented virtually or in-person as indicated in the schedule below.
- Speaker
- Poster Presenter
- Attendee
- Virtual Attendee
-
Faten Alamri
virginia commonwealth university and Princess Nourah Bint Abdul Rahman University
-
Terrence Alsup
Courant Institute of Mathematical Sciences
-
Andrea Arnold
Worcester Polytechnic Institute
-
Mazlum Ferhat Arslan
Middle East Technical University
-
Saed Asaly
Ariel University
-
Yonatan Ashenafi
Rensselaer Polytechnic Institute
-
Karim Azumah
Pan African University for Basic Sciences, Technology and Innovation
-
Zhe Bai
Lawrence Berkeley National Lab
-
Christopher Beattie
Virginia Tech
-
George Biros
ICES
-
Liliana Borcea
University of Michigan
-
Tamara Broderick
Massachusetts Institute of Technology
-
Peter Challenor
University of Exeter
-
Zheng “Leslie” Chen
University of Massachusetts Dartmouth
-
Yanlai Chen
University of Massachusetts, Dartmouth
-
Haiyan Cheng
Willamette University
-
Merlise Clyde
Duke University
-
Paul Constantine
University of Colorado Boulder
-
Keisha Cook
Tulane University
-
Gregory Darnell
ICERM
-
Julio de Lima Nicolini
Ohio State University
-
Anton Dereventsov
Oak Ridge National Laboratory
-
Mingchang Ding
University of Delaware
-
Alireza Doostan
University of Colorado Boulder
-
Kathryn Drake
Boise State University
-
Vladimir Druskin
Worcester Polytechnic Institute
-
Fariba Fahroo
AFOSR
-
Lihong Feng
Max Planck Institute for Dynamics of Complex Technical Systems
-
Guosheng Fu
Brown University
-
Khaled Furati
King Fahd University of Petroleum and Minerals
-
Nathan Gibson
Oregon State University
-
Anna Gilbert
University of Michigan
-
Serkan Gugercin
Virginia Tech
-
Christian Himpe
Max Planck Institute for Dynamics of Complex Technical Systems
-
Jeffrey Hokanson
University of Colorado Boulder
-
Muhammad Izzatullah
King Abdullah University of Science and Technology
-
Lijie Ji
UMass Dartmouth/Shanghai Jiao Tong University
-
Sebastian Kaltenbach
Technical University of Munich - Continuum Mechanics Group
-
Isabelle Kemajou-Brown
Morgan State University
-
Abdul Khaliq
Middle Tennessee State University
-
Misha Kilmer
Tufts University
-
BONGSIK KIM
American University of Ras Al Khaimah
-
Liang Kong
University of Illinois at Springfield
-
Peter Kramer
Rensselaer Polytechnic Institute
-
Christian Kümmerle
Johns Hopkins University
-
J. Nathan Kutz
University of Washington
-
Sanda LEFTERIU
IMT Lille Douai
-
Xingjie Li
University of North Carolina at Charlotte
-
Jichun Li
University of Nevada Las Vegas
-
Fengyan Li
Rensselaer Polytechinic Institute
-
Guang Lin
Purdue University
-
Marcella M Gomez
University of California Santa Cruz
-
Anna Ma
University of California Irvine
-
Mauro Maggioni
Johns Hopkins University
-
Michael Mahoney
University of California, Berkeley
-
Reza Malek-Madani
U.S. Naval Academy
-
Krithika Manohar
California Institute of Technology
-
Youssef Marzouk
Massachusetts Institute of Technology
-
F. Patricia Medina
Yeshiva University
-
Petar Mlinarić
Max Planck Institute for Dynamics of Complex Technical Systems
-
Ryleigh Moore
University of Utah
-
Shari Moskow
Drexel University
-
Akil Narayan
University of Utah
-
Carmeliza Navasca
University of Alabama at Birmingham
-
Nicole Nellesen
RWTH Aachen University
-
Kayode Olumoyin
Middle Tennessee State University
-
Melkior Ornik
University of Illinois at Urbana-Champaign
-
Juming Pan
Rowan University
-
Benjamin Peherstorfer
Courant Institute, New York University
-
Zhichao Peng
Rensselaer Polytechnic Institute
-
Paris Perdikaris
University of Pennsylvania
-
Elizabeth Qian
MIT
-
Jeffrey Regier
University of California, Berkeley
-
Rosemary Renaut
Arizona State University
-
Viktor Reshniak
Oak Ridge National Laboratory
-
Abel Rodriguez
University of California, Santa Cruz
-
Quratulan Sabir
National College of business administration and economics
-
Themistoklis Sapsis
MIT
-
Robert Scheichl
University of Heidelberg
-
Michael Schneier
University of Pittsburgh
-
Babak Shahbaba
University of California - Irvine
-
Yeonjong Shin
Brown University
-
Devin Smith
Rensselaer Polytechnic Institute
-
Sarah Soleiman
Université Paris 1 Panthéon Sorbonne
-
Susanna Spektor
Sheridan college institute of technology
-
Georg Stadler
CIMS NYU
-
Jemima Tabeart
University of Edinburgh
-
Sui Tang
Johns Hopkins University
-
Qi Tao
USTC
-
Shanyin Tong
New York University
-
THOMAS Torku
Middle Tennessee State University
-
Wayne Isaac Uy
Courant Institute of Mathematical Sciences, NYU
-
Daniele Venturi
University of California, Santa Cruz
-
Karen Veroy-Grepl
TU Eindhoven
-
Soledad Villar
NYU
-
Xiaoyu Wang
Florida State University
-
Min Wang
Duke University
-
Zhu Wang
University of South Carolina
-
Rachel Ward
University of Texas at Austin
-
Jonathan Weare
Courant Institute, New York University
-
Jinlong Wu
California Institute of Technology
-
Yiming Xu
University of Utah
-
Yang Yang
Michigan Technological University
-
uzma yasmeen
Comstas Islamabad Lahore campus
-
Mikhail Zaslavskiy
Schlumberger
-
Jia Zhao
Utah State University
-
Shouhao Zhou
Penn State College of Medicine
-
Cong Zhou
Indiana University
-
Xueyu Zhu
University of Iowa
-
Lingjiong Zhu
Florida State University
-
Jorn Zimmerling
University of Michigan