Organizing Committee
- Elham Azizi
Columbia University - Andrew Blumberg
Columbia University - Lorin Crawford
Brown University - Bianca Dumitrascu
Columbia University - Antonio Moretti
Barnard College - Itsik Pe'er
Columbia University
Abstract
Single-cell assays provide a tool for investigating cellular heterogeneity and have led to new insights into a variety of biological processes that were not accessible with bulk sequencing technologies. Assays generate observations of many different molecular types and a grand mathematical challenge is to devise meaningful strategies to integrate data gathered across a variety of different sequencing modalities. The first-order approach to do this is to analyze the projected data by clustering. Keeping more refined shape information about the data enables more meaningful and accurate analysis. Geometric methods include (i) Manifold learning: Whereas classical approaches (PCA, metric MDS) assume projection to a low-dimensional Euclidean subspace, manifold learning finds coordinates that lie on a not necessarily flat or contractible manifold. (ii) Topological data analysis: Algebraic topology provides qualitative descriptors of global shape. Integrating these descriptors across feature scales leads to the notion of “persistence” and a new family of geometric invariants that vary continuously with the data. (iii) Optimal transport: Generalizations of the “earthmover” distances that have been popular in object matching and computer graphics have been used extensively to match and align data sets from different modalities. This workshop will introduce mathematicians and biologists to these powerful computational tools as well as the theory behind them, and highlight open questions and new advances in these areas.
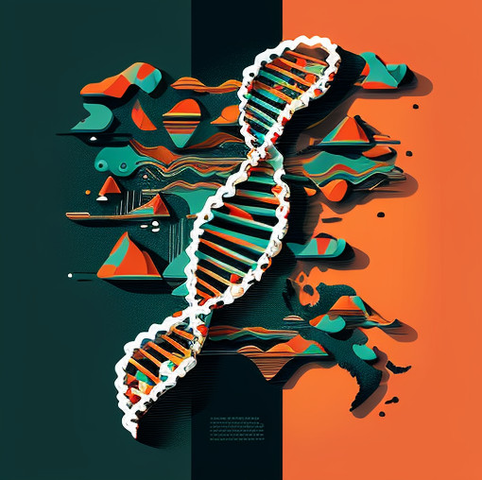
Confirmed Speakers & Participants
Talks will be presented virtually or in-person as indicated in the schedule below.
- Speaker
- Poster Presenter
- Attendee
- Virtual Attendee
-
Laura Bagamery
Harvard Medical School
-
Dhananjay Bhaskar
Yale University
-
Maria Brbic
EPFL
-
Pablo Camara
University of Pennsylvania
-
Carlo Cannistraci
Tsinghua
-
Yuyang Chen
Harvard University
-
Lorin Crawford
Brown University
-
Bianca Dumitrascu
Columbia University
-
Hongsong Feng
Michigan State University
-
Elana Fertig
Johns Hopkins University
-
Anna Gilbert
Yale University
-
Aleksandrina Goeva
Broad Institute
-
Nicolas Gort Freitas
Harvard Medical School
-
Yuta Hozumi
Michigan State University
-
Saiful Islam
State University of New York at Buffalo
-
Yuqi Lei
Brown University
-
Sivan Leviyang
Georgetown University
-
Ying Ma
Brown University
-
Katherine Moore
Amherst College
-
Junalyn Navarra-Madsen
TEXAS WOMAN'S UNIVERSITY
-
Stanley Nicholson
Brown University
-
Mor Nitzan
The Hebrew University of Jerusalem
-
Lior Pachter
Caltech
-
Itsik Pe'er
Columbia University
-
Benjamin Raphael
Princeton University
-
Bjorn Sandstede
Brown University
-
Ritambhara Singh
Brown University
-
Whitney Sloneker
Brown University
-
Elias Ventre
The University of British Columbia
-
Joshua Welch
University of Michigan
-
Ian Wong
Brown University
-
Guanao Yan
University of California Los Angeles
-
Jiaqi Zhang
Brown University
-
Yu Zhong
Brown University
-
Peijie Zhou
Peking University
-
Yu Zhu
Brown University
Workshop Schedule
Monday, December 11, 2023
-
8:50 - 9:00 am ESTWelcome11th Floor Lecture Hall
- Session Chair
- Brendan Hassett, ICERM/Brown University
-
9:00 - 9:45 am ESTRecovering hidden layers of information in single-cell data11th Floor Lecture Hall
- Virtual Speaker
- Mor Nitzan, The Hebrew University of Jerusalem
- Session Chair
- Itsik Pe'er, Columbia University
Abstract
Gene expression profiles of a cellular population, generated by single-cell RNA sequencing, contain rich, 'hidden' information about biological state and collective multicellular behavior that is lost during the experiment or not directly accessible, including cell type, cell cycle phase, gene regulatory patterns, cell-cell communication, and location within the tissue-of-origin. In this talk I will discuss several methods, based on a combination of spectral, machine learning, and dynamical systems approaches, to disentangle and enhance particular spatiotemporal signals that cellular populations encode and interpret their manifestation across space and time in tissues.
-
10:00 - 10:30 am ESTCoffee Break11th Floor Collaborative Space
-
10:30 - 11:15 am ESTDiscovering cell types across tissues, disease states and species11th Floor Lecture Hall
- Speaker
- Maria Brbic, EPFL
- Session Chair
- Itsik Pe'er, Columbia University
Abstract
Biomedical data poses multiple hard challenges that break conventional machine learning assumptions. In this talk, I will present machine learning methods that have the ability to bridge heterogeneity of scRNA-seq and spatial single cell datasets by transferring cell type annotations across tissues, disease states and species. I will discuss the findings and impact these methods have for annotating comprehensive single-cell atlas datasets and discovery of novel cell types.
-
11:30 am - 1:30 pm ESTLunch/Free Time
-
1:30 - 2:15 pm ESTPhysics of life via spatial reconstruction of single-cells: from network geometry to coalescent embedding of transcriptomic networks11th Floor Lecture Hall
- Speaker
- Carlo Cannistraci, Tsinghua
- Session Chair
- Itsik Pe'er, Columbia University
Abstract
Physics of life aims to reveal physical principles and develop concepts that explain the dynamic self-organization of living active matter, encompassing topics at different scales, from flocking birds to the dynamic activation of the actomyosin cortex. The spatial organization of single cells or small groups of cells in a tissue remains an open question in the physics of life and Single-cell RNA sequencing (scRNA-seq) has emerged as a powerful tool whose transcriptomic data are rich in information but difficult to interpret without leveraging nonlinear topological machine learning methods for latent space manifold analysis. To address this problem, we start from a discovery in a new field of physics of complexity called network geometry. This is a machine intelligence theory for nonlinear embedding of networks of complex interconnected systems in a geometrical space, which is called coalescent embedding (CE) because it relies on a phenomenon that in physics of complexity takes the name of angular coalescence. This phenomenon states that for a network that derives from a complex interconnected system, whose connections between its parts (nodes) emerge in a latent geometrical space, the network embedding in a 2D or 3D visualization space will display a typical pattern of node aggregation that respects the intrinsic geometry of the system in the latent geometrical space in terms of both congruence and navigability. Building upon this theory, we developed a novel algorithm called De Novo Coalescent Embedding (D-CE) which unveils single-cell mesoscale spatial organization, where densely interacting network neighborhoods or communities are associated with spatial domains. D-CE generates accurate landmark-free and model-free 3D spatial reconstructions of single cells in a tissue from their gene expressions and nominates spatial marker genes to guide template-based reconstruction. Comprehensive comparisons of existing reconstruction methods have demonstrated the advantage of D-CE, incorporating additional optional steps of specimen shape-template fitting and marker-based one-to-one position mapping to enhance the visual clarity and evaluation of its reconstructions. D-CE can reveal previously underappreciated regulators or morphogens associated with molecular spatial gradients ruling pattern formation during biological processes.
-
2:30 - 3:00 pm ESTCoffee Break10th Floor Collaborative Space
-
3:00 - 3:45 pm ESTA label-refinement method for detection of disease-relevant cells in case-control single-cell transcriptomics data11th Floor Lecture Hall
- Speaker
- Aleksandrina Goeva, Broad Institute
- Session Chair
- Itsik Pe'er, Columbia University
Abstract
Leveraging single-cell transcriptomics to characterize how cells change across conditions, e.g. how cells respond to disease, is an important task that can broaden our understanding of illnesses and pave the way to new treatments. Some cross-condition datasets, e.g. case-control single-cell studies, come with a particular 'mislabeling' problem -- namely, only a fraction of the cells in the case condition may actually be disease-affected, while the rest may be unperturbed and indistinguishable from control cells, despite being labeled as case. I will demonstrate that, in this scenario, the standard single-cell clustering routine can fail to identify the subset of perturbed cells. To address this limitation, I will present a novel computational framework that refines the condition labels to accurately reflect the perturbation status of each cell. I will show applications of the method to human multiple myeloma precursor conditions and a mouse model of demyelination. Finally, I will discuss the framework's modular components and the flexibility it provides in choosing amongst a range of dimensionality reductions and prediction models to best match the problem.
-
4:00 - 5:30 pm ESTReception10th Floor Collaborative Space
Tuesday, December 12, 2023
-
9:30 - 10:15 am ESTBayesian Inference of RNA Velocity from Multi-Lineage Single-Cell Data11th Floor Lecture Hall
- Speaker
- Joshua Welch, University of Michigan
- Session Chair
- Ritambhara Singh, Brown University
Abstract
Experimental approaches for measuring single-cell gene expression can observe each cell at only one time point, requiring computational approaches for reconstructing the dynamics of gene expression during cell fate transitions. RNA velocity is a promising computational approach for this problem, but existing inference methods fail to capture key aspects of real data, limiting their utility. To address these limitations, we developed VeloVAE, a Bayesian model for RNA velocity inference. VeloVAE uses variational Bayesian inference to estimate the posterior distribution of latent time, latent cell state, and kinetic rate parameters for each cell. Our approach addresses key limitations of previous methods by inferring a global time and cell state value for each cell; explicitly modeling the emergence of multiple cell types; incorporating prior information such as time point labels; using scalable minibatch optimization; and quantifying parameter uncertainty. These improvements allow VeloVAE to accurately model gene expression dynamics in complex biological systems, including hematopoiesis, induced pluripotent stem cell reprogramming, the entire developing brain, neurogenesis, and the entire developing mouse.
-
10:30 - 11:00 am ESTCoffee Break11th Floor Collaborative Space
-
11:30 am - 12:15 pm ESTClustering-independent estimation of cell abundances in bulk tissues using single-cell RNA-seq data11th Floor Lecture Hall
- Speaker
- Pablo Camara, University of Pennsylvania
- Session Chair
- Ritambhara Singh, Brown University
Abstract
Single-cell RNA sequencing has transformed the study of biological tissues by enabling transcriptomic characterizations of their constituent cell states. Computational methods for gene expression deconvolution use this information to infer the cell composition of related tissues profiled at the bulk level. However, current deconvolution methods are restricted to discrete cell types and have limited power to make inferences about continuous cellular processes like cell differentiation or immune cell activation. In this talk, I will discuss ConDecon, an approach for inferring the likelihood for each cell in a reference single-cell dataset to be present in a tissue that has been profiled at the bulk level, without relying on cluster labels or cell-type specific gene expression signatures. ConDecon makes use of the space of gene rank correlations to approximate the space of cell abundances. We will demonstrate the utility of ConDecon using gene expression data of pediatric ependymal tumors, where we uncover the implication of neurodegenerative microglial inflammatory pathways in the mesenchymal transformation of these tumors.
-
12:25 - 12:30 pm ESTGroup Photo (Immediately After Talk)11th Floor Lecture Hall
-
12:30 - 2:30 pm ESTLunch/Free Time
-
2:30 - 3:30 pm ESTProblem Session11th Floor Lecture Hall
- Session Chair
- Itsik Pe'er, Columbia University
-
3:30 - 4:00 pm ESTCoffee Break11th Floor Collaborative Space
-
4:00 - 5:00 pm ESTMentorship PanelPanel Discussion - 11th Floor Lecture Hall
- Session Chair
- Aleksandrina Goeva, Broad Institute
-
4:00 - 5:00 pm ESTWork/Free Time
Wednesday, December 13, 2023
-
9:30 - 10:15 am ESTMetric representations: Algorithms, Geometry, (and Applications?)11th Floor Lecture Hall
- Speaker
- Anna Gilbert, Yale University
- Session Chair
- Bianca Dumitrascu, Columbia University
Abstract
Given a set of distances amongst points, determining what metric representation is most “consistent” with the input distances or the metric that best captures the relevant geometric features of the data is a key step in many machine learning algorithms. In this talk, we focus on 3 specific metric constrained problems, a class of optimization problems with metric constraints: metric nearness (Brickell et al. (2008)), weighted correlation clustering on general graphs (Bansal et al. (2004)), and metric learning (Bellet et al. (2013); Davis et al. (2007)). The initial motivation for this work comes from scRNA-seq analysis; we will discuss possible applications at the end.
-
10:30 - 11:00 am ESTCoffee Break11th Floor Collaborative Space
-
11:30 am - 12:15 pm ESTForecasting immunotherapy for predictive medicine11th Floor Lecture Hall
- Virtual Speaker
- Elana Fertig, Johns Hopkins University
- Session Chair
- Bianca Dumitrascu, Columbia University
Abstract
Therapeutic response in cancer depends critically on the state of cancer cells and additional cells in the tumor microenvironment, both of which evolve over time. New single-cell and spatial molecular technologies enable unprecedented characterization of these states across molecular and cellular scales, but are challenging to interpret due to the high-dimensional nature of these data. New computational methodologies are essential to interpret these data. We demonstrate how the Bayesian non-negative matrix factorization method, CoGAPS, enables us to learn patterns associated with immunotherapy response and resistance from single cell data. While cellular composition is important, the spatial distribution of cells in the tumor microenvironment further mediate response and resistance to therapies. Emerging spatial molecular technologies provide a powerful tool to model these interactions. We demonstrate how CoGAPS further models intra-tumor heterogeneity of the tumor microenvironment and tumor cells from Visium spatial transcriptomics data. Finally, further integration of the molecular features learned from multi-omics data with mathematical modeling has the power to leverage the intra- and inter-tumor heterogeneity these data uncover to predict mechanisms of immunotherapy response and resistance.
-
12:30 - 2:30 pm ESTLunch/Free Time
-
2:30 - 3:15 pm ESTModeling Tissue Organization using Spatial Transcriptomics11th Floor Lecture Hall
- Speaker
- Benjamin Raphael, Princeton University
- Session Chair
- Bianca Dumitrascu, Columbia University
Abstract
Spatial transcriptomics technologies measure RNA expression at thousands of locations in a tissue sample providing information about the spatial distribution of cell types and the spatial variation in gene expression across a tissue. However, these measurements are typically sparse with high rates of missing data. In this talk, I will present algorithms that address data sparsity by modelling spatial correlations between measurements within and across tissue slices. First, our Belayer algorithm describes variation in gene expression in a single slice using a model of a layered tissue that consists of stacked layers with distinct cell type composition, such as found in the brain and skin. We extend this approach to more general tissue geometries using an interpretable deep learning model that derives a one-dimensional coordinate, the isodepth, that models both discontinuous and continuous variation in gene expression. Finally, our PASTE algorithm aligns and integrates spatial transcriptomics data from multiple slices from the same tissue enabling downstream applications such as differential gene expression and 3D reconstruction of tissues. The advantages of these methods will be illustrated on spatial transcriptomics data from multiple tissue types.
-
3:30 - 4:00 pm ESTCoffee Break11th Floor Collaborative Space
-
4:00 - 4:45 pm ESTA theory of trajectory inference for scRNA-seq and lineage tracing data11th Floor Lecture Hall
- Speaker
- Elias Ventre, The University of British Columbia
- Session Chair
- Bianca Dumitrascu, Columbia University
Abstract
A core challenge for modern biology is how to infer the trajectories of individual cells from population-level time courses of high-dimensional gene expression data. Birth and death of cells present a particular difficulty: existing trajectory inference methods cannot distinguish variability in net proliferation from cell differentiation dynamics, and hence require accurate prior knowledge of the proliferation rate. In this talk, I will first present the core ideas behind Global Waddington-OT (gWOT), a method for trajectory inference from time-courses of scRNA-seq datasets, based on regularized optimal transport, which offers rigorous theoretical guarantees when birth and death can be neglected or are known prior to the observation. I will then show how recent CRISPR-based measurement technologies, by giving access to the lineage tree describing shared ancestry within a population of cells, allow to build on gWOT to disentangle proliferation and differentiation without any prior knowledge. Death and/or subsampling may nevertheless introduce a bias in the inferred trajectories, that we describe explicitly and argue to be inherent to these lineage tracing data.
Thursday, December 14, 2023
-
9:30 - 10:15 am ESTOptimal-transport based algorithms for aligning single cell multi-omics data11th Floor Lecture Hall
- Speakers
- Bjorn Sandstede, Brown University
- Ritambhara Singh, Brown University
- Session Chair
- Pablo Camara, University of Pennsylvania
Abstract
This talk will give an overview of two algorithms for aligning single cell multi-omics data. The first algorithm, SCOT (Single Cell alignment using Optimal Transport), aims to align cells from different multi-omics measurements, such as gene expression, chromatin accessibility, and DNA methylation data. This approach is based on entropy-regularized Gromov-Wasserstein optimal transport and attempts to conserve pairwise distances of nearby data points. We show the efficacy of this algorithm using synthetic data and two experimental co-assay data sets. Next, we will present AGW (Augmented Gromov-Wasserstein), a novel formulation that allows us to align both samples (cells) and features (genes) simultaneously and effectively across different single cell datasets. We show the improved performance of this formulation and its ability to align features and provide supervision on either sample or feature level for challenging single cell alignment tasks.
-
10:30 - 11:00 am ESTCoffee Break11th Floor Collaborative Space
-
11:30 am - 12:15 pm ESTIdentifying gene regulatory networks (GRNs) and predicting gene expression by leveraging temporal single cell experiments11th Floor Lecture Hall
- Speakers
- Bjorn Sandstede, Brown University
- Ritambhara Singh, Brown University
- Session Chair
- Pablo Camara, University of Pennsylvania
Abstract
In this talk, we will first discuss the application of optimal-transport-based algorithms to the identification of gene-regulatory networks using temporal single-cell gene expression counts. After demonstrating its effectiveness on simulated data, we apply this method to single-cell gene expression from the human somatic cell population undergoing conversion to induced pluripotent stem cells and developmental timepoints in Drosophila. Our results recover the temporal sequencing of gene expression data and make predictions for the underlying GRNs. Next, we propose a generative model scNODE that can predict realistic in silico single cell gene expression at any time point to enable temporal downstream analyses. scNODE integrates a variational autoencoder (VAE) with neural ordinary differential equations (ODEs) to predict gene expression in a continuous and non-linear latent space. Importantly, scNODE adds a regularization term to integrate the overall dynamics of cell developments to the latent space, such that the learned latent representation is informative and interpretable.
-
12:30 - 2:30 pm ESTLunch/Free Time
-
2:30 - 3:15 pm ESTDeciphering Spatial Landscape of Cell Type and Tissue Structure in Spatial Transcriptomics11th Floor Lecture Hall
- Speaker
- Ying Ma, Brown University
- Session Chair
- Pablo Camara, University of Pennsylvania
Abstract
Spatially resolved transcriptomics (SRT) studies are becoming increasingly common and increasingly large, offering unprecedented opportunities to characterize the spatial and functional organization of complex tissues. In this talk, I will present two methods to address these challenges for dissecting heterogeneity in cell type spatial distribution and tissue structure. I will first introduce CARD for spatially informed cell type deconvolution. CARD takes advantage of the spatial correlation structure to enable accurate and robust deconvolution of spatial transcriptomics across technologies with different spatial resolutions and in the presence of mismatched scRNA-seq references. I will also introduce IRIS, for reference-informed integrative spatial domain detection. IRIS integrates multiple SRT tissue slices jointly, while explicitly considering correlation both within and across slices. This approach produces biologically interpretable spatial domains. We demonstrate the advantages of IRIS through in-depth analysis of six SRT datasets from different technologies across various tissues, species, and spatial resolutions. As a result, IRIS uncovers the fine-scale structures of brain regions, reveals the spatial heterogeneity of distinct tumor microenvironments, and characterizes the structural changes of the seminiferous tubes in the testis associated with diabetes. This is achieved with a speed and accuracy that existing approaches cannot match.
-
3:30 - 4:00 pm ESTCoffee Break11th Floor Collaborative Space
-
4:00 - 4:45 pm ESTLearning dynamic regulatory networks from single-cell data11th Floor Lecture Hall
- Speaker
- Dhananjay Bhaskar, Yale University
- Session Chair
- Pablo Camara, University of Pennsylvania
Abstract
Complex systems, such a gene regulatory networks and neuronal networks, are characterized by intricate interactions between entities that evolve dynamically over time. Accurate inference of these dynamic relationships is crucial for understanding and predicting system behavior. In this talk, I will describe a novel framework, called RiTINI, for inferring time-varying interaction graphs in complex systems using a novel combination of space-and-time attention and graph neural ODEs. The graph attention mechanism in RiTINI allows the model to adaptively focus on the most relevant interactions in time and space, while the graph neural ODEs enable continuous-time modeling of the system's dynamics. I will demonstrate RiTINI performance on various simulated and real-world single-cell datasets.
Friday, December 15, 2023
-
9:00 - 9:45 am ESTThe systems biology of a single cell11th Floor Lecture Hall
- Speaker
- Lior Pachter, Caltech
- Session Chair
- Sivan Leviyang, Georgetown University
Abstract
I will discuss the rationale for a systems biology approach for single-cell genomics as motivated by questions arising in functional genomics and developmental biology.
-
10:00 - 10:30 am ESTCoffee Break11th Floor Collaborative Space
-
10:30 - 11:15 am ESTCross-species alignment of dynamic processes from single-cell expression data11th Floor Lecture Hall
- Speaker
- Laura Bagamery, Harvard Medical School
- Session Chair
- Sivan Leviyang, Georgetown University
Abstract
A wealth of single-cell expression data is now available for a range of phylogenetically diverse species. Comparative analysis of such data can offer valuable insight into the fundamental features of individual cell types and tissues as well as the relationship between changes in gene expression and the generation of novel phenotypes. Here, we discuss challenges associated with cross-species transcriptomic analysis and highlight molecular mechanisms of developmental evolution which are masked in conventional dataset integration techniques. We present a novel framework for mapping gene expression across species by generating coupled yet independent genewise alignments, which we formalize as an optimization problem. We apply this method to detect evolutionarily conserved and divergent features associated with erythropoietic differentiation.
All event times are listed in ICERM local time in Providence, RI (Eastern Daylight Time / UTC-4).
All event times are listed in .
ICERM local time in Providence, RI is Eastern Daylight Time (UTC-4). Would you like to switch back to ICERM time or choose a different custom timezone?
Request Reimbursement
This section is for general purposes only and does not indicate that all attendees receive funding. Please refer to your personalized invitation to review your offer.
- ORCID iD
- As this program is funded by the National Science Foundation (NSF), ICERM is required to collect your ORCID iD if you are receiving funding to attend this program. Be sure to add your ORCID iD to your Cube profile as soon as possible to avoid delaying your reimbursement.
- Acceptable Costs
-
- 1 roundtrip between your home institute and ICERM
- Flights on U.S. or E.U. airlines – economy class to either Providence airport (PVD) or Boston airport (BOS)
- Ground Transportation to and from airports and ICERM.
- Unacceptable Costs
-
- Flights on non-U.S. or non-E.U. airlines
- Flights on U.K. airlines
- Seats in economy plus, business class, or first class
- Change ticket fees of any kind
- Multi-use bus passes
- Meals or incidentals
- Advance Approval Required
-
- Personal car travel to ICERM from outside New England
- Multiple-destination plane ticket; does not include layovers to reach ICERM
- Arriving or departing from ICERM more than a day before or day after the program
- Multiple trips to ICERM
- Rental car to/from ICERM
- Flights on a Swiss, Japanese, or Australian airlines
- Arriving or departing from airport other than PVD/BOS or home institution's local airport
- 2 one-way plane tickets to create a roundtrip (often purchased from Expedia, Orbitz, etc.)
- Travel Maximum Contributions
-
- New England: $350
- Other contiguous US: $850
- Asia & Oceania: $2,000
- All other locations: $1,500
- Note these rates were updated in Spring 2023 and superseded any prior invitation rates. Any invitations without travel support will still not receive travel support.
- Reimbursement Requests
-
Request Reimbursement with Cube
Refer to the back of your ID badge for more information. Checklists are available at the front desk and in the Reimbursement section of Cube.
- Reimbursement Tips
-
- Scanned original receipts are required for all expenses
- Airfare receipt must show full itinerary and payment
- ICERM does not offer per diem or meal reimbursement
- Allowable mileage is reimbursed at prevailing IRS Business Rate and trip documented via pdf of Google Maps result
- Keep all documentation until you receive your reimbursement!
- Reimbursement Timing
-
6 - 8 weeks after all documentation is sent to ICERM. All reimbursement requests are reviewed by numerous central offices at Brown who may request additional documentation.
- Reimbursement Deadline
-
Submissions must be received within 30 days of ICERM departure to avoid applicable taxes. Submissions after thirty days will incur applicable taxes. No submissions are accepted more than six months after the program end.