Organizing Committee
- Carlotta Domeniconi
George Mason University - Ellen Gasparovic
Union College - Giseon Heo
University of Alberta - Kathryn Leonard
California State University, Channel Islands - Regina Liu
Rutgers University - Julie Mitchell
University of Wisconsin - Deanna Needell
UCLA - Linda Ness
Rutgers University - Emina Soljanin
Rutgers University - Sibel Tari
Middle East Technical University - Xu Wang
Wilfrid Laurier University
Abstract
Research Collaboration Workshop for Women in Data Science and Mathematics (WiSDM). This program will bring together women at all stages of their careers, from graduate students to senior researchers, to collaborate on problems in data science. The scientific focus will be on cutting edge problems in the areas of predictive modeling, multi-scale representation and feature selection, statistical and topological learning, and related areas. Data science is a cross-disciplinary field relying on statistics, computer science and mathematics and driven by problems in many other disciplines. While data science has emerged as a prominent new field enrolls record numbers and attracts research talents from many scientific disciplines, the role of theoretical and applied mathematics has not been highly visible. Mathematics provides many structured representations that can be in the analysis of data arising from such diverse fields as geometric measure theory, classical analysis, computational topology, shape theory, algebraic statistics, and spectral graph theory. Furthermore, mathematics may enable more classes of data sets to be represented as measures and distributions which could then leverage classical statistical techniques.
Meanwhile, mathematics and computer science are two of three disciplines with the lowest percentage of women attaining PhDs (28% and 24%, respectively). Creating explicit research bridges between these groups will provide networks of women with similar research interests, and will also create pathways for the female-friendly culture in statistics to make its way into mathematics and computer science. This workshop will generate research collaborations, and highlight mathematics as a primary contributor. Successful applicants will be assigned to a research problem based on their expertise. Each group will aim to include a more senior person in each of statistics, machine learning, and mathematics.
Partially supported by NSF-HRD 1500481 - AWM ADVANCE grant. Additional support for some participant travel will be provided by DIMACS in association with its Special Focus on Information Sharing and Dynamic Data Analysis. Co-sponsored by Brown's Data Science Initiative.
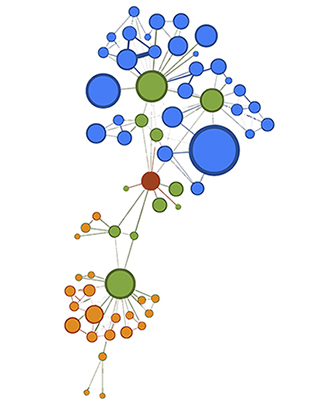
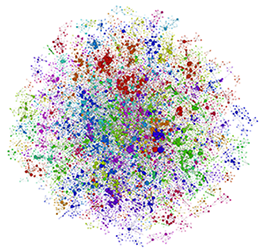
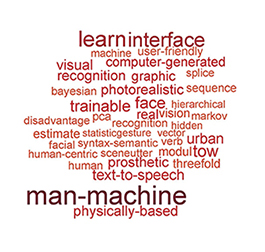
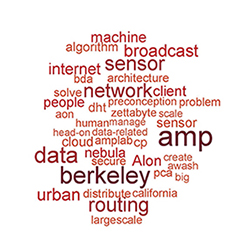
Confirmed Speakers & Participants
Talks will be presented virtually or in-person as indicated in the schedule below.
- Speaker
- Poster Presenter
- Attendee
- Virtual Attendee
-
Sarah Anderson
University of St. Thomas
-
Gülce Bal
Middle East Technical University
-
Elizabeth Beer
Institute for Defense Analyses
-
Yang Chen
University of Michigan
-
Haiyan Cheng
Willamette University
-
Carlotta Domeniconi
George Mason University
-
Natalie Durgin
Spiceworks
-
Julia E Grigsby
Boston College
-
Hillary Fairbanks
University of Colorado Boulder
-
Brie Finegold
Rincon Research Corporation
-
Alyson Fox
University of Colorado Boulder
-
Anna Grim
Brown University
-
Rachel Grotheer
Goucher College
-
Giseon Heo
University of Alberta
-
Chenxi Huang
Yale/YNHH Center for Outcomes Research and Evaluation
-
Aarti Jajoo
Baylor College of Medicine
-
Ann Johnston
Penn State University
-
Gauri Joshi
IBM T. J. Watson Research Center
-
Nianqiao Ju
Harvard Univeristy
-
Fatemeh Kazemikordasiabi
Rutgers University
-
Christine Kelley
University of Nebraska-Lincoln
-
Soojeong Kim
Yonsei University
-
Katherine Kinnaird
Brown University
-
Fiona Knoll
Clemson University
-
Alona Kryshchenko
California State University of Channel Islands
-
Kathryn Leonard
California State University, Channel Islands
-
Rachel Levanger
Rutgers University
-
Shuang Li
Colorado School of Mines
-
Anna Little
Jacksonville University
-
Anna Ma
Claremont Graduate University
-
Priya Mani
George Mason University
-
Gretchen Matthews
Clemson University
-
Carolyn Mayer
University of Nebraska - Lincoln
-
Melissa McGuirl
Brown University
-
F. Patricia Medina
Worcester Polytechnic Institute
-
Jesse Metcalf-Burton
Department of Defense
-
Julie Mitchell
University of Wisconsin
-
Deanna Needell
UCLA
-
Linda Ness
Rutgers University
-
Melissa Ngamini
Morehouse College
-
Megan Owen
Lehman College, City University of New York
-
Jing Qin
Montana State University
-
Franziska Seeger
University of Washington
-
Emina Soljanin
Rutgers University
-
Sui Tang
Johns Hopkins University
-
Sibel Tari
Middle East Technical University
-
Marilyn Vazquez Landrove
George Mason University
-
Xu Wang
Wilfrid Laurier University
-
Melanie Weber
Princeton University
-
Tina Woolf
Claremont Graduate University
-
Karamatou Yacoubou Djima
Amherst College
-
Lori Ziegelmeier
Macalester College
Workshop Schedule
Monday, July 17, 2017
Time | Event | Location | Materials |
---|---|---|---|
9:00 - 9:30am EDT | Registration | 121 South Main Street Providence RI 11th Floor Collaborative Space | |
9:30 - 10:00am EDT | Welcome to WiSDM | 11th Floor Lecture Hall | |
10:00 - 10:15am EDT | Project 1 Introduction - Julie Mitchell, University of Wisconsin | 11th Floor Lecture Hall | |
10:15 - 10:30am EDT | Project 2 Introduction - Linda Ness, Rutgers University | 11th Floor Lecture Hall | |
10:30 - 10:45am EDT | Project 3 Introduction - Giseon Heo, University of Alberta | 11th Floor Lecture Hall | |
10:45 - 11:15am EDT | Coffee Break | 11th Floor Collaborative Space | |
11:15 - 11:30am EDT | Project 4 Introduction - Deanna Needell, UCLA | 11th Floor Lecture Hall | |
11:30 - 11:45am EDT | Project 5 Introduction - Carlotta Domeniconi, George Mason University | 11th Floor Lecture Hall | |
11:45 - 12:00pm EDT | Project 6 Introduction - Emina Soljanin, Rutgers University | 11th Floor Lecture Hall | |
12:00 - 1:30pm EDT | Break for Lunch/ Free Time | ||
1:30 - 2:00pm EDT | Form Groups | 11th Floor Lecture Hall | |
2:00 - 3:30pm EDT | Group Work | 11th Floor Lecture Hall | |
3:30 - 4:00pm EDT | Coffee Break | 11th Floor Collaborative Space | |
4:00 - 5:00pm EDT | Group Work | 11th Floor Lecture Hall | |
5:00 - 6:00pm EDT | Welcome Reception | 11th Floor Collaborative Space |
Tuesday, July 18, 2017
Time | Event | Location | Materials |
---|---|---|---|
9:00 - 10:30am EDT | Group Work | 11th Floor Lecture Hall | |
10:30 - 11:00am EDT | Coffee Break | 11th Floor Collaborative | |
11:00 - 12:30pm EDT | Group Work | 11th Floor Lecture Hall | |
12:30 - 2:00pm EDT | Break for Lunch/ Free Time | ||
2:00 - 3:30pm EDT | Group Work | 11th Floor Lecture Hall | |
3:30 - 4:00pm EDT | Coffee Break | 11th Floor Collaborative Space | |
4:00 - 5:00pm EDT | Group Work | 11th Floor Lecture Hall | |
5:00 - 6:00pm EDT | WiSDM Panel | 11th Floor Lecture Hall |
Wednesday, July 19, 2017
Time | Event | Location | Materials |
---|---|---|---|
9:00 - 10:30am EDT | Group Check-Ins | 11th Floor Lecture Hall | |
10:30 - 10:40am EDT | Group and Project Photos | 11th Floor Lecture Hall | |
10:40 - 11:10am EDT | Coffee Break | 11th Floor Collaborative | |
11:00 - 12:30pm EDT | Group Work | 11th Floor Lecture Hall | |
12:30 - 2:00pm EDT | Break for Lunch/ Free Time | ||
2:00 - 3:30pm EDT | Group Work | 11th Floor Lecture Hall | |
3:30 - 4:00pm EDT | Coffee Break | 11th Floor Collaborative Space | |
4:00 - 5:00pm EDT | Group Work | 11th Floor Lecture Hall | |
5:00 - 6:15pm EDT | Jeff Brock & Sohini Ramachandran | 11th Floor Lecture Hall |
Thursday, July 20, 2017
Time | Event | Location | Materials |
---|---|---|---|
9:00 - 10:30am EDT | Group Work | 11th Floor Lecture Hall | |
10:30 - 11:00am EDT | Coffee Break | 11th Floor Collaborative | |
11:00 - 12:30pm EDT | Group Work | 11th Floor Lecture Hall | |
12:30 - 2:00pm EDT | Break for Lunch/ Free Time | ||
2:00 - 3:30pm EDT | Group Work | 11th Floor Lecture Hall | |
3:30 - 4:00pm EDT | Coffee Break | 11th Floor Collaborative Space | |
4:00 - 5:00pm EDT | Group Work | 11th Floor Lecture Hall |
Friday, July 21, 2017
Time | Event | Location | Materials |
---|---|---|---|
9:00 - 9:15am EDT | Group 6 Presentation | 11th Floor Lecture Hall | |
9:30 - 9:45am EDT | Group 2 Presentation | 11th Floor Lecture Hall | |
10:00 - 10:15am EDT | Group 3 Presentation | 11th Floor Lecture Hall | |
10:15 - 10:45am EDT | Coffee Break | 11th Floor Collaborative | |
10:45 - 11:00am EDT | Group 4 Presentation | 11th Floor Lecture Hall | |
11:15 - 11:30am EDT | Group 5 Presentation | 11th Floor Lecture Hall | |
11:45 - 12:00pm EDT | Group 1 Presentation | 11th Floor Lecture Hall | |
2:30 - 3:00pm EDT | Coffee Break | 11th Floor Collaborative Space |
Project Descriptions
Project 1: Predictive Models for Molecular Data
Using data generated in past molecular modeling projects, participants will be encouraged to apply a range of machine learning and informatics techniques to analyze the data and build/optimize predictive models. Some prior models have been built with a hundred or so experimental data points, while other bimolecular models have utilized over 50,000 data points.
Through these exercises, participants will learn the applicability of varied machine learning methods to datasets of different sizes (both the number of data points and the length of feature vectors). In addition, best practices in cross-validation will be discussed, so as to give participants a sense of how to organize their data in a way that is most rigorous when existing relationships among the data points are known. It may be possible to include deep learning methods as well.
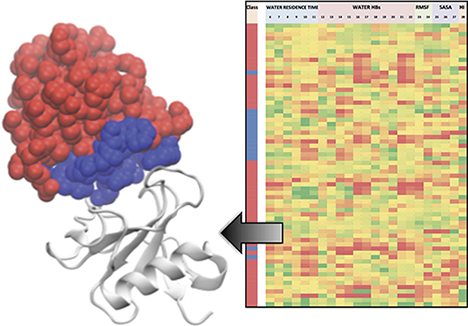
Project 2: Representation of Data as Multi-Scale Features and Measures
Recently, multi-scale representation theorems from harmonic analysis and geometric measure theory due to Fefferman, Kenig and Pipher, Peter W. Jones, Coifman and Lafon, etc. have been exploited to compute canonical multi-scale representations of data samples. The representations have been exploited for multiple purposes, including for supervised machine learning (where they provide automatically constructed features), for unsupervised learning of regimes and anomalies, for statistical fusion and construction of confidence measures, and for data visualization.
The methods are very general and have been demonstrated on network and sensor data sets. Multi-resolution inference has been proposed by X. Meng as an important new research challenge in statistics. This research collaboration would enable assessment of the applicability of multiscale representation approaches to other types of data (e.g., molecular modeling data used to study obstructive sleep apnea, and possibly a cyber-security related data set). It would also serve the purpose of introducing this approach to statistical researchers who may be interested in statistical fusion, data depth, and confidence measures. In addition, new multi-scale methods for representation of data as measures characterizing mathematical properties of the data (e.g. geometric properties) could be developed and applied.
Project 3: Inferential Models Founded in Statistical and Topological Learning
Pediatric obstructive sleep apnea (OSA) is a form of sleep-disordered breathing characterised by recurrent episodes of partial or complete airway obstruction during sleep, and is prevalent in one to five percent of school-aged children. While the gold standard for pediatric OSA diagnosis is an overnight polysomnography (PSG), the high cost of this procedure and the lack of sleep clinics often precludes children from receiving necessary treatment and ultimately has a significant impact on overall future quality of life through numerous OSA-associated sequelae.
A systematic review and meta-analysis of pediatric OSA literature reveals a link between craniofacial morphology and OSA prevalence in pediatric patients. The presence of this relationship has led to the hypothesis that experienced dentists and orthodontists may be able to identify children at risk of developing OSA simply by observing a child’s craniofacial characteristics.
In this project, we propose a study of real-word pediatric OSA datasets in order to (1) develop a statistical and topological learning (STL) model that can accurately predict OSA severity, and (2) verify whether OSA severity measurements given by orthodontists are comparable to those given by sleep specialists via PSG. To tackle the substantial number of variables inherent in OSA data—including time series data (e.g.: EOG, EMG, and ECG), three dimensional images of the face and upper airway, medical history, dental measurements, various questionnaires, blood and urine samples, and other sleep-disordered breathing risk factors—we propose a review of existing STL methods in order to achieve the above research goals. In particular, we will incorporate techniques from various fields, including time series analysis, shape analysis, persistent homology, zigzag persistence, graphical LASSO, tensor regression, as well as numerous clustering techniques from statistics and machine learning.
Project 4: Stochastic signal processing for high dimensional data (Deanna Needell)
In today's world, data is exploding at a faster rate than computer architectures can handle. For that reason, mathematical techniques to analyze large-scale objects must be developed. One mathematical method that has gained a lot of recent attention is the use of sparsity and stochastic designs. Sparsity captures the idea that high dimensional signals often contain a very small amount of intrinsic information. Often, through randomized designs, signals can be captured using a very small number of measurements. On the recovery side, stochastic methods can accurately estimate signals from those measurements in the underdetermined setting, as well as solve large-scale systems in the highly overdetermined setting.
Participants will learn the mathematical background to such acquisition and reconstruction approaches, and we will explore the impact on many applications of interest to modern researchers and practitioners. In particular, we will select several applications of interest to the group and design stochastic algorithms for those frameworks. The participants will run experiments on synthetic data from those applications, and work on theoretical guarantees for the methods.
Project 5: The Hubness Phenomenon in High Dimensional Spaces
Recent studies have established the emergence of an interesting phenomenon in high dimensional data, known as hubness. Hubness causes certain data examples to appear more often than others as neighbors of points, thus generating a skewed distribution of nearest neighbor counts.
High dimensional data are ubiquitous, e.g. text, images, and biological data can easily contain tens of thousands of features. Often, though, data have an intrinsic dimensionality that is embedded within the full dimensional space.
In this project we'll investigate the relationship between the hubness phenomenon and the intrinsic dimensionality of data, with the ultimate goal of recovering the subspaces data lie within. We are particularly interested in the scenario where the relevant subspace depends on the location within the input space. The findings of this study may enable effective subspace clustering of data, as well as outlier identification.
Project 6: Codes for Data Storage with Queues for Data Access
Large volumes of data, which are being collected for the purpose of knowledge extraction, have to be reliably, efficiently, and securely stored. Retrieval of large data files from storage has to be fast (and often anonymous and private). This project is concerned with big data storage and access, and its relevant mathematical disciplines include algebraic coding and queueing theory. Large-scale cloud data storage and distributed file systems, e.g., Amazon EBS and Google FS, have become the backbone of many applications such as web searching, e-commerce, and cluster computing.
Cloud services are implemented on top of a distributed storage layer that acts as a middleware to the applications, and also provides the desired content to the users, whose interests range from performing data analytics to watching movies. Coding theory has been essential in providing solutions for reliable, efficient, and secure telecommunications, but these solutions are inadequate when storing and moving very large files across networks is necessary. Many new deep problems that arise in such circumstances simultaneously belong to both fundamental coding and queueing theory, but have so far been mostly separately addressed.
Participants of this project will, according to their preferences regarding combinatorics, algebra and probability, learn about and work on some coding and/or queueing problems in the era of big data. The hope is that some would take interest in both of these interwoven and indispensable aspects of big data storage and access. Undergraduates are welcome.
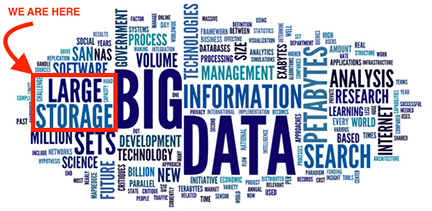