Organizing Committee
- Philipp Hennig
Max Planck Institute for Intelligent Systems - George Karniadakis
Brown University - Michael Osborne
University of Oxford - Houman Owhadi
California Institute of Technology - Paris Perdikaris
Massachusetts Institute of Technology
Abstract
There is an urgent and unmet need to formally analyze, design, develop and deploy advanced methods and algorithms that can scale in statistical and computational efficiency to the size of modern data sets and the complexity of contemporary mathematical models. Addressing this need will require a holistic approach involving new foundational theory, algorithms, and programming language design.
The emerging research theme of Probabilistic Scientific Computing (PSC) or Probabilistic Numerics lies at the nexus of these overlapping directions. It aims to improve statistical quantification of uncertainty, improve computational efficiency, and build more effective and scalable numerical methods for statistical models by leveraging the natural correspondence between computation and inference.
The primary goal of the workshop is to introduce recent results and new directions in probabilistic scientific computing to the US research communities in statistics and machine learning, in numerical analysis, and in theoretical computer science.
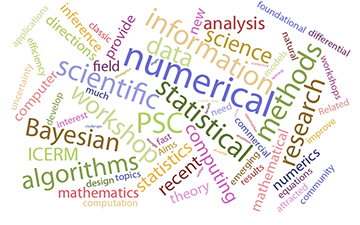
Confirmed Speakers & Participants
Talks will be presented virtually or in-person as indicated in the schedule below.
- Speaker
- Poster Presenter
- Attendee
- Virtual Attendee
-
Mark Ainsworth
Brown University
-
Hessam Babaee
University of Pittsburgh
-
Tom Bertalan
Princeton University
-
Ilias Bilionis
Purdue University
-
Tamara Broderick
Massachusetts Institute of Technology
-
Zhizhong Chen
Brown University
-
Yanlai Chen
University of Massachusetts, Dartmouth
-
Oksana Chkrebtii
The Ohio State University
-
Bastien Chopard
University of Geneva
-
Jon Cockayne
University of Warwick
-
David Cooper
Brown University
-
Andreas Damianou
Amazon.com
-
Jana de Wiljes
University of Potsdam
-
Yuchen Dong
WPI
-
Jeová Farias Sales Rocha Neto
Brown university
-
Guosheng Fu
Brown University
-
Roman Garnett
Washington University in St. Louis
-
Tarsilo Girona
Brown University
-
Javier Gonzalez Hernandez
Amazon.com
-
Mamikon Gulian
Brown University
-
Ling Guo
Shanghai Normal University
-
Stephen Guth
Massachusetts Institute of Technology
-
Matthew Harrison
Brown University
-
Md Mehedi Hasan
Brown University
-
Philipp Hennig
Max Planck Institute for Intelligent Systems
-
Chris Huber
Brown University
-
Ilse Ipsen
North Carolina State University
-
Jiahua Jiang
University of Massachusetts Dartmouth
-
Nianqiao Ju
Harvard Univeristy
-
Andrew Kaluzny
Brown University
-
Hamid Karani
Georgia Institute of Technology
-
George Karniadakis
Brown University
-
Toni Karvonen
Aalto University
-
Hans Kersting
Max Planck Institute for Intelligent Systems
-
Yannis Kevrekidis
Princeton University
-
Julia Kroos
BCAM - Basque Centre for Applied Mathematics
-
Neil Lawrence
University of Sheffield
-
Seungjoon Lee
Brown University
-
Xingjie Li
University of North Carolina at Charlotte
-
Jichun Li
University of Nevada Las Vegas
-
Zhen Li
Brown University
-
Guang Lin
Purdue University
-
Zhiping Mao
Brown University
-
Govind Menon
Brown University
-
Jeff Miller
Harvard T.H. Chan School of Public Health
-
Saviz Mowlavi
Massachusetts Institute of Technology
-
Chris Oates
University of Technology Sydney
-
Paulo Orenstein
Stanford University
-
Houman Owhadi
California Institute of Technology
-
Paris Perdikaris
Massachusetts Institute of Technology
-
Prashant Rai
Sandia National Labs
-
Maziar Raissi
Brown University
-
Florian Schaefer
California Institute Of Technology
-
Daniele Schiavazzi
University of Notre Dame
-
Leila Setayeshgar
Providence College
-
Fangying Song
Brown University
-
Panos Stinis
Pacific National Laboratory
-
Tim Sullivan
Free University of Berlin
-
Kyle Taljan
Case Western Reserve University
-
Molei Tao
Georgia Institute of Technology
-
Tom Trogdon
University of California Irvine
-
Zhong Yi Wan
MIT
-
Grzegorz Wasilkowski
University of Kentucky
-
Nickolas Winovich
Purdue University
-
Xiu Yang
Pacific Northwest National Laboratory
-
Liu Yang
Brown University
-
Alireza Yazdani
Brown Univeristy
-
Zhongqiang Zhang
Worcester Polytechnic Institute
-
Xueyu Zhu
University of Iowa