Organizing Committee
- Ellen Gasparovic
Union College - Kathryn Leonard
Occidental College - Linda Ness
Rutgers University
Abstract
WiSDM 2019 is a research collaboration workshop targeted toward people working in data science and mathematics. This program will bring together researchers at all stages of their careers, from graduate students to senior researchers, to collaborate on problems in data science.
Data science is typically characterized as work at the intersection of mathematics, computer science, statistics, and an application domain. The scientific focus will be on cutting-edge problems in network analysis for gene detection, group dynamics, graph clustering, novel statistical and topological learning algorithms, tensor product decompositions, reconciliation of assurance of anonymity and privacy with utility measures for data transfer and analytics, as well as efficient and accurate completion, inference and fusion methods for large data and correlations.
Applications are now open. Applicants should rank their top 3 choices of projects in their personal statement. Project descriptions can be found below.
Application deadline extended to March 31, 2019.
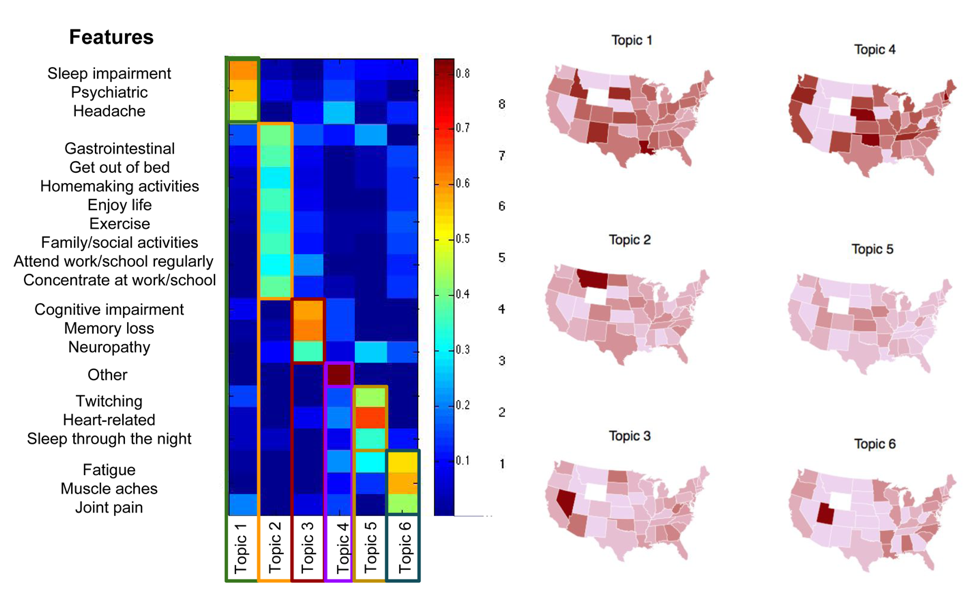
Group Leads
- Andrea Bertozzi
UCLA - Carlotta Domeniconi
George Mason University - Giseon Heo
University of Alberta - Misha Kilmer
Tufts University - Deanna Needell
UCLA - Umut Ozbek
Icahn School of Medicine at Mount Sinai - Emina Soljanin
Rutgers University
Confirmed Speakers & Participants
Talks will be presented virtually or in-person as indicated in the schedule below.
- Speaker
- Poster Presenter
- Attendee
- Virtual Attendee
-
Miju Ahn
Southern Methodist University
-
Loulwah Alsumait
Kuwait University
-
Elena Balashova
Princeton University
-
Allison Beemer
New Jersey Institute of Technology
-
Andrea Bertozzi
UCLA
-
Haripriya Chakraborty
The Graduate Center, CUNY
-
Jocelyn Chi
NC State
-
Julia Chuang
Boston College
-
Carlotta Domeniconi
George Mason University
-
Sanghamitra Dutta
Carnegie Mellon University
-
Nicole Eikmeier
Grinnell College
-
Noha El-Zehiry
Siemens
-
Emily Evans
Brigham Young University
-
Amrina Ferdous
Boise State University
-
Asli Genctav
Middle East Technical University
-
Rachel Grotheer
Goucher College
-
Weihong Guo
Case Western Reserve University
-
Jamie Haddock
University of California, Los Angeles
-
Giseon Heo
University of Alberta
-
Genesis Islas
Arizona State University
-
Haewon Jeong
Carnegie Mellon University
-
Lara Kassab
Colorado State University
-
Misha Kilmer
Tufts University
-
Anna Konstorum
Center for Computing Sciences, Institue for Defense Analyses
-
Alona Kryshchenko
California State University of Channel Islands
-
Esther Lamken
Independent Researcher
-
Harlin Lee
Carnegie Mellon University
-
Kathryn Leonard
Occidental College
-
Anna Little
Michigan State University
-
Yifei Lou
The University of Texas at Dallas
-
Anna Ma
University of California, San Diego
-
Priya Mani
George Mason University
-
F. Patricia Medina
Yeshiva University
-
Denali Molitor
University of California, Los Angeles
-
Anarina Murillo
Arizona State University and Brown University
-
Deanna Needell
UCLA
-
Linda Ness
Rutgers University
-
Umut Ozbek
Mount Sinai
-
Brenda Praggastis
Pacific Northwest National Laboratory
-
Emilie Purvine
Pacific Northwest National Laboratory
-
Elizabeth Qian
MIT
-
Jing Qin
University of Kentucky
-
Anusha Madushani Rajapaksha Wasala Mudiyanselage
Boston Medical Center
-
Cynthia Rush
Columbia University
-
Kritika Singhal
Ohio State University
-
Emina Soljanin
Rutgers University
-
Mansi Sood
Carnegie Mellon University, Pittsburgh
-
Melissa Stockman
Grabango
-
Kaisa Taipale
University of Minnesota
-
Sibel Tari
Middle East Technical University
-
Sarah Tymochko
Michigan State University
-
Marilyn Vazquez Landrove
ICERM
-
Xu Wang
Wilfrid Laurier University
-
Chuntian Wang
The University of Alabama
-
Li Wang
University of Texas at Arlington
-
Emily Winn
Brown University
-
Karamatou Yacoubou Djima
Amherst College
Workshop Schedule
Monday, July 29, 2019
Time | Event | Location | Materials |
---|---|---|---|
8:30 - 8:55am EDT | Registration - ICERM 121 South Main Street, Providence RI 02903 | 11th Floor Collaborative Space | |
8:55 - 9:00am EDT | Welcome - ICERM Director | 11th Floor Lecture Hall | |
9:00 - 9:30am EDT | Organizer Welcome - Ellen Gasparovic, Kathryn Leonard, and Linda Ness | 11th Floor Lecture Hall | |
9:30 - 10:10am EDT | Project Introductions | 11th Floor Lecture Hall | |
10:15 - 10:45am EDT | Coffee/Tea Break | 11th Floor Collaborative Space | |
10:45 - 12:00pm EDT | Group Work | 11th Floor Lecture Hall | |
12:00 - 1:30pm EDT | Break for Lunch / Free Time | ||
1:30 - 3:00pm EDT | Group Work | 11th Floor Lecture Hall | |
3:00 - 3:30pm EDT | Coffee/Tea Break | 11th Floor Collaborative Space | |
3:30 - 5:00pm EDT | Group Work | 11th Floor Lecture Hall | |
5:00 - 6:30pm EDT | Welcome Reception | 11th Floor Collaborative Space |
Tuesday, July 30, 2019
Time | Event | Location | Materials |
---|---|---|---|
9:00 - 10:30am EDT | Group Work | 11th Floor Lecture Hall | |
10:30 - 11:00am EDT | Coffee/Tea Break | 11th Floor Collaborative Space | |
11:00 - 12:00pm EDT | Group Work | 11th Floor Lecture Hall | |
12:00 - 1:30pm EDT | Working Lunch - Food provided by ICERM | 11th Floor Collaborative Space | |
1:30 - 3:00pm EDT | Group Work | 11th Floor Lecture Hall | |
3:00 - 3:30pm EDT | Coffee/Tea Break | 11th Floor Collaborative Space | |
3:30 - 4:30pm EDT | WiSDM Panel | 11th Floor Lecture Hall | |
4:30 - 6:00pm EDT | Informal Group Updates | 11th Floor Lecture Hall |
Wednesday, July 31, 2019
Time | Event | Location | Materials |
---|---|---|---|
9:00 - 10:00am EDT | Group Check-ins | 11th Floor Lecture Hall | |
10:00 - 10:15am EDT | Group and Project Photos | 11th Floor Lecture Hall | |
10:15 - 10:45am EDT | Coffee/Tea Break | 11th Floor Collaborative Space | |
10:45 - 12:00pm EDT | Group Work | 11th Floor Lecture Hall | |
12:00 - 1:30pm EDT | Break for Lunch / Free Time | ||
1:30 - 3:30pm EDT | Group Work | 11th Floor Lecture Hall | |
3:30 - 4:00pm EDT | Coffee/Tea Break | 11th Floor Collaborative Space | |
4:00 - 4:50pm EDT | Informal Group Updates | 11th Floor Lecture Hall | |
5:00 - 7:00pm EDT | Group Outing TBD (Optional, Self-Paid) | 11th Floor Lecture Hall |
Thursday, August 1, 2019
Time | Event | Location | Materials |
---|---|---|---|
9:00 - 10:30am EDT | Group Work | 11th Floor Lecture Hall | |
10:30 - 11:00am EDT | Coffee/Tea Break | 11th Floor Collaborative Space | |
11:00 - 12:00pm EDT | Group Work | 11th Floor Lecture Hall | |
12:00 - 1:30pm EDT | Break for Lunch / Free Time | ||
1:30 - 3:30pm EDT | Group Work | 11th Floor Lecture Hall | |
3:30 - 4:00pm EDT | Coffee/Tea Break | 11th Floor Collaborative Space | |
4:00 - 5:00pm EDT | Informal Group Updates | 11th Floor Lecture Hall |
Friday, August 2, 2019
Time | Event | Location | Materials |
---|---|---|---|
9:00 - 9:30am EDT | Group Work | 11th Floor Lecture Hall | |
9:30 - 10:30am EDT | Group Presentations | 11th Floor Lecture Hall | |
10:30 - 11:00am EDT | Coffee/Tea Break | 11th Floor Collaborative Space | |
11:00 - 12:00pm EDT | Group Presentations | 11th Floor Lecture Hall | |
12:00 - 1:30pm EDT | Break for Lunch / Free Time | ||
1:30 - 3:30pm EDT | Group Presentations | 11th Floor Lecture hall | |
3:30 - 4:00pm EDT | Coffee/Tea Break | 11th Floor Collaborative Space | |
4:00 - 4:30pm EDT | Group Presentations | 11th Floor Lecture Hall | |
4:30 - 5:00pm EDT | Closing Remarks | 11th Floor Lecture Hall |
Project Descriptions
Project 1: Graph regularization of high dimensional data
Leadership: Andrea Bertozzi (UCLA), Yifei Lou (UT Dallas)
There has been a large volume of mathematical models to process signals and/or images that are defined on a regular domain. As for irregular or unsorted data graph modeling often provides a flexible representation to capture the underlying structures. However, some key notions in image processing, such as translation, convolution, and dilation, are not straightforward on graphs. This project aims to develop a graph-regularized framework in data analysis, to address key challenges regarding both theoretical and computational aspects in graph representation, and to demonstrate its capacity in various applications. More specifically, given a graph, the graph Fourier transform is defined in terms of the eigenvectors of graph Laplacian. As a result, aforementioned image processing operators can be carried out on the graph frequency domain. This approach offers a possible way to process data on the graph, but computational efficiency remains an open question. The project will be supplemented with prototypical applications in data science, such as social networks, electric power grids, and hyperspectral imaging.
Project 2: Tensor Tools for Multiway Data Analysis
Leadership: Misha Kilmer (Tufts University)
Many problems in scientific settings involve operators or data that are inherently multidimensional: consider the storage of digital video data referenced by frame number, color band, and spatial dimensions; data on gene responses to different chemical combinations; discrete PDE snapshot data according to three spatial dimensions and a time dimension. It makes sense to treat multiway (aka tensor) data in its natural format. However, it is in this regime that traditional results from linear algebra break down, necessitating the development of new constructs and algorithms to treat multiway data.
Recent work has shown that with the right tensor tools, processing data in tensor format rather than matrix format can definitively provide additional structural information that allows for better compression and analysis of the data, for example in facial recognition. However, different data sets involve different structural characteristics, and some tensor decompositions are better suited than others to reveal the corresponding latent features. On the other hand, for large datasets, the computational time for the decompositions must also be a consideration.
In this project, participants with learn about some of the state-of-the-art tensor techniques from both a mathematical and computational viewpoint for compressing and mining of multiway data. Particular attention will be given to one such approach using tensor-tensor products whose associated algebraic framework permits a computationally efficient extension of linear algebraic and data analytic concepts such as PCA, dictionary learning, clustering and neural nets. In addition to investigating the use of some of these tensor algorithms on real data, we will also consider open questions in the theoretical understanding of the data analysis tools built on this new mathematical framework.
Project 3: Inferences on Incomplete and Multi-Modal Data with Applications to Medical Data
Leadership: Deanna Needell (UCLA)
Recent technological and scientific advances have allowed the acquisition of vast amounts of various types of data, including medical and medically related survey data. Such an abundance of information should lead to new scientific understanding in the mechanism of disease, diagnosis, and treatment. However, the large-scale nature of this data requires novel mathematical techniques in order to effectively extract and analyze the information. This project will address three main existing challenges in analyzing this type of data. Our goals focus on (i) analyzing large-scale but highly incomplete data, (ii) the need for computationally efficient methods that still provide very accurate inferential results, (iii) data fusion techniques for analyzing a wide array of data types in one cohesive framework. We will use recently acquired Lyme disease data as a motivating example in the design and testing of our methods.
Project 4: Modeling Spatial and Temporal Dynamics in Networks
Leadership: Carlotta Domeniconi (George Mason University), Sibel Tari (Middle East Technical University)
Humans are social beings that organize and form groups, or communities. Groups are defined as a set of densely-connected nodes relative to the rest of the network. Some groups are short-lived and survive for a fraction of their members' life while others exist over many lifetimes. A group brings together a set of nodes, but these nodes may serve different roles within the group. As an example, the figure shows a subgraph from a Facebook snapshot network. Nodes are colored by their primary role and sized according to their in-degree. Edges are sized according to the number of interactions they represent.
The purpose of this project is to improve our understanding of group dynamics while avoiding having to model the behavior of individuals. Instead, we will consider groups as first-class entities in a network and identify useful features, some of which may be derived from group members, which indicate the current status and predict the future outcome of a group.
Potential approaches to be explored include nonlinear dynamic systems modeling, linear algebra techniques, and embedding techniques via deep learning. Initial studies indicate that some roles may fit a prey-predator temporal dynamic model. We will also consider modeling both temporal dynamics and spatial configurations (e.g. via reaction-diffusion models). Hyper graphs that capture multi-way interactions among individuals may also be considered. We anticipate using networks of collaborators (e.g. Scratch users), social networks (e.g. Facebook), and possibly financial networks for the detection of anomalous trends.
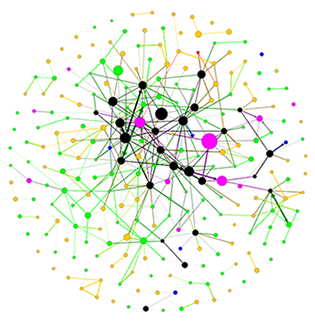
Project 5: Development of a Statistical Topological Learning Algorithm
Leadership: Giseon Heo (University of Alberta), Xu Wang (Laurier University)
The analysis and interpretation of high dimensional data has become increasingly more challenging, requiring sophisticated analytic techniques. Thus, it may no longer be effective to independently apply data analysis methods from specific scientific disciplines such as statistics, mathematics, or computing science to solve a complex problem. We aim to develop a novel statistical and topological learning (STL) algorithm which will be used for analyzing high-dimensional data based upon persistent homology from computational topology and geometry, neural networks from deep learning, as well as classical and advanced methods in statistics and machine learning. The STL algorithm will be applied to chronic diseases in order to aid clinicians to create the most optimal treatment plan for each patient.
Project 6: User Anonymity and Data Privacy
Leadership: Emina Soljanin (Rutgers University)
Simultaneous knowledge extraction by multiple institutions from large volumes of data has to honor the demand for privacy and anonymity from individuals. This project will explore recon- ciling assurance of anonymity and privacy with the various utility measures in data transfer and data analytics. We have done some preliminary work on anonymity mixes which are, in some form, a building block of many practical anonymity systems.
An anonymity (threshold) Mix is a sophisticated message router that receives and holds packets from message sources and forwards them in a batch to their respective destinations only when it accumulates messages from some prescribed number of sources. Because of such simultaneous transmissions of messages, the identities of communicating pairs remain hidden to possible adversaries that seek to link message sources and destinations. The price of achieving anonymity in this way is delay, because messages are held at the Mix until a batch of a certain size is formed. This talk will describe two promising ideas about how to compute the delay, and present some preliminary results. One idea is to model batch mixes as generalized assembly-like queues and develop an approximate queuing analysis of these objects. The other idea is to model the source/destination channels as urns and messages as balls, and compute the channels' queues occupancy, and the time it takes to accumulate enough messages to have a departure, as a variant of the coupon collection problem.
Project 7: Comparing/Combining Clustering Techniques for Omics Data Integration
Leadership: Umut Ă–zbek (Icahn School of Medicine at Mount Sinai)
Network analysis, detecting modules and pathway enrichment have been widely used to identify candidate genes, which would be used as targets for drug development and outcome prediction. Using the comprehensive and multi-dimensional data generated by The Cancer Genome Atlas (TCGA), which is a collaboration between the National Cancer Institute and the National Human Genome Research Institute, participants will be encouraged to build networks using a conditional graphical model and investigate different clustering techniques to select genes that are potentially associated with the disease. Through these exercises, participants will learn a novel statistical technique to construct a network, visualize their network using online tools and statistical software, applying clustering algorithms to create modules and interpret the results statistically, clinically and biologically.