Organizing Committee
- Marta D'Elia
Pasteur Labs. and Stanford University - George Karniadakis
Brown University - Alexander Lavin
Pasteur Labs & Inst. for Simulation Intelligence
Abstract
Scientific Machine Learning (SciML) is a merger of computational sciences and data-driven machine learning, implemented in software as a set of abstractions to leverage existing domain knowledge and physics models within learning schemes and accelerated computing platforms. Only now are the component technologies becoming mature and integrated, with the purpose of providing robust and reliable ML that encodes domain knowledge and yields interpretable solutions in nearly all sciences at any scale—from particle physics and protein folding, to epidemiology and economics, to climate and astrophysics.
While recent years have shown immense research progress in accelerating classical physics solvers and discovering new governing laws for complex physical systems, SciML methods and applications fall short of real-world utility. Across all physical and life sciences the technical maturity and validations necessary are lacking, failing, or unknown to SciML research. In the context of traditional and advanced industrial settings, this process requires operating in digital-physical environments marked by large-scale, three-dimensional, multi-modal data streams that are confounded with noise, sparsity, irregularities and other complexities that are common with machines and sensors interacting with the real, physical world.
This workshop focuses on the most recent advances in the field of applied SciML by bringing together the world-leading players in its industrialization, those at the forefront of advancing R&D armed with the critical experience necessary for building and scaling SciML in the wild.
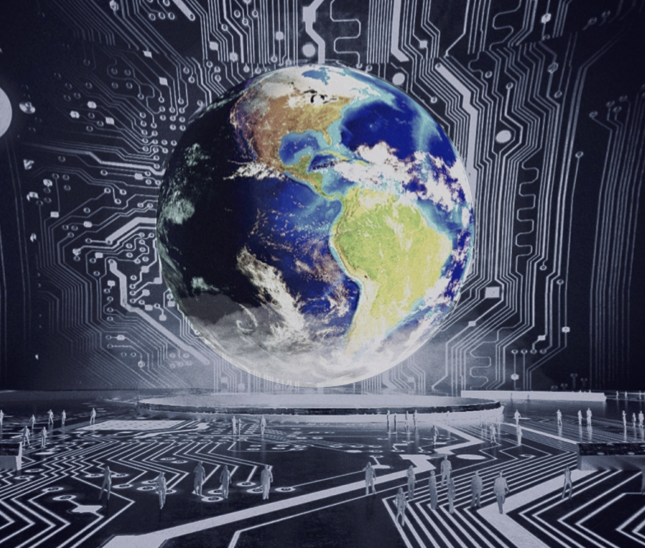
Confirmed Speakers & Participants
Talks will be presented virtually or in-person as indicated in the schedule below.
- Speaker
- Poster Presenter
- Attendee
- Virtual Attendee
-
Jonas Actor
Sandia National Laboratories
-
Richard Ahfeld
Monolith
-
Nazanin Ahmadi
Brown University
-
Elie Alhajjar
RAND
-
Arash Alizadeh
University of Missouri-Columbia
-
Diego Andrade
Pasteur Labs
-
Alessandro Angioi
Pasteur Labs & ISI
-
Eytan Bakshy
Meta
-
Isabel Barrio Sanchez
University of Pittsburgh
-
Dmitry Batenkov
Tel Aviv University
-
Karianne Bergen
Brown University
-
Juan Betts
PredictiveIQ
-
Rabindra Bhattarai
Dassault Systeme's
-
Jing Bi
Dassault Systemes Americas
-
Federica Bragone
KTH Royal Institute of Technology
-
Davide Bray
Harvard University
-
Sam Buercklin
Independent Research Software Engineer
-
SANDEEP REDDY BUKKA
NATIONAL ENERGY TECHNOLOGY LABORATORY
-
Yu-Chin Chan
Siemens Technology
-
Anirban Chandra
Shell
-
Bob Chatham
Slater Technology Fund
-
Jorge Cisneros Paz
The University of Texas at Austin
-
Marta D'Elia
Pasteur Labs. and Stanford University
-
Mario De Florio
Brown University
-
Alireza Doostan
University of Colorado Boulder
-
Karthik Duraisamy
University of Michigan
-
Clara Edmonds
Ørsted
-
Paul Escapil-Inchauspé
Data Observatory Foundation
-
Tiffany Fan
Stanford University
-
Somdatta Goswami
Johns Hopkins University
-
Rad Haghi
Tufts University
-
Matthew Hancock
Veryst Engineering
-
Dirk Hartmann
Siemens Digital Industries Software
-
Quercus Hernandez
University of Pennsylvania
-
Lauryn Holmes
Spelman College
-
Ameya Jagtap
Division of Applied Mathematics
-
Jordan Jalving
Pasteur Labs
-
Balaji Jayaraman
GE Research
-
Ricardo Kabila
University of Massachusetts Dartmouth
-
Adar Kahana
Brown University
-
Erdi Kara
Spelman College
-
George Karniadakis
Brown University
-
Mehdi Khalloufi
Dow Chemical
-
Ehsan Kharazmi
Brown University
-
David Knezevic
Akselos
-
Vijay Kumar
Columbia University
-
Alexander Lavin
Pasteur Labs & Inst. for Simulation Intelligence
-
Xiaoyi Lu
FM Global Insurance Company
-
Tittu Mathew
Siemens Energy Canada Limited
-
Tingwei Meng
UCLA
-
Danyal Mohaddes
FM Global
-
Rambod Mojgani
The University of Chicago
-
Martin Molina Fructuoso
Brandeis University
-
Kateryna MOROZOVSKA
KTH Royal Institute of Technology
-
Shancong Mou
Georgia Institute of Technology
-
Nikhil Muralidhar
Stevens Institute of Technology
-
Saleh Nabi
Schneider Electric
-
Mohammad Nabian
Nvidia
-
Victor Oancea
Dassault Systemes
-
Vivek Oommen
Brown University
-
Ehsan Osloub
Veryst Engineering LLC
-
shaowu pan
Rensselaer Polytechnic Institute
-
James Parr
Trillium Technologies
-
Ahmad Payvan
Brown University
-
Vignesh Perumal
UHV3D, Inc.
-
Adrienne Propp
Stanford University
-
Youcef Rahal
Pasteur Labs
-
Kaveh Rahimzadeh Berenji
Georgia Institute of Technology
-
Rishikesh Ranade
ANSYS
-
Jasmine Ratchford
Carnegie Mellon University Software Engineering Institute
-
Danielle Robinson
Amazon Web Services AI Labs
-
Marco Romanelli
New York University
-
Lauren Sanders
NASA
-
Daniele Schiavazzi
University of Notre Dame
-
Qin Sheng
Baylor University
-
Ankit Shrivastava
Sandia National Laboratory
-
Andrew Spann
Veryst Engineering, LLC
-
Apoorv Srivastava
Stanford University
-
Panos Stinis
Pacific Northwest National Laboratory
-
JINGYE TAN
Cornell University
-
Aikaterini Tavri
Brown University
-
Eleonora Maria Tronci
Northeastern University
-
Mohammad Vaezi
University of Rochester
-
Nick Vasios
Veryst Engineering
-
Edison Vazquez
Schneider Electric
-
Haruko Wainwright
MIT
-
Yi Wang
FM Global
-
Tianshi Xu
Emory University
-
Sangeeta Yadav
University of Wisconsin
-
Yadong Zeng
Altair Engineering Inc
-
Haokai Zhao
Massachusetts Institute of Technology
-
Ming Zhong
Illinois Institute of Technology
-
Quanyan Zhu
New York University
Workshop Schedule
Saturday, March 23, 2024
-
8:50 - 9:00 am EDTWelcome11th Floor Lecture Hall
-
9:00 - 9:30 am EDTData-driven modelling to solve intractable physics11th Floor Lecture Hall
- Speaker
- Richard Ahfeld, Monolith
- Session Chair
- Marta D'Elia, Pasteur Labs. and Stanford University
Abstract
All engineering companies want to build high-quality products as efficiently and quickly as possible. Physics-based simulation or CAE can provide answers virtually and faster without building prototypes or performing tests. However, in a recent survey among 163 engineering leaders conducted by the market research institute Forrester*, 55% of leaders said that they found CAE too inaccurate. Despite years of continuous progress on CAE methods, there are still many problems for which solving PDEs does not provide accurate insights – either because the underlying physics is too complex, or the boundary conditions are insufficiently known. To give an example, calibrating a P2D model for battery simulation requires so much data of a real battery cell prototype, that the battery must already have been manufactured and have undergone a large number of tests before the model becomes useful. In a case like this, where the physics is intractable, data-driven simulation can be a faster and easier way to model and optimise system performs. Intractable physics problems are everywhere in engineering, from materials science to dynamic system behaviour. This talk will look at various industrial case studies in which data-driven modelling was used to accelerate product development, including the Porsche Jota race team who used data-driven models for tire degradation and won Le Mans, Honeywell who found that data-driven methods can beat CFD in ultra-sound flow measurement, Kautex Textron who developed a more accurate method to model fuel sloshing noise, as well as BMW group who managed to predict the result of crash tests without CAE. The talk will end by focusing on battery simulation. In the author’s opinion, this is the most impactful area of engineering where machine learning can help model intractable physics, as the electrochemical relationships of batteries are notoriously hard to model and require a huge of amount of time-consuming testing. *Commissioned by Monolith
-
9:40 - 10:10 am EDTNVIDIA Modulus: An Open-Source Framework for Scientific Machine Learning11th Floor Lecture Hall
- Speaker
- Mohammad Nabian, Nvidia
- Session Chair
- Marta D'Elia, Pasteur Labs. and Stanford University
Abstract
High-fidelity simulations, crucial in science and engineering, are traditionally time-consuming and computationally demanding, hindering rapid iteration in various applications like design analysis and optimization. NVIDIA Modulus, a cutting-edge scientific machine learning platform, revolutionizes these processes by creating surrogate models that significantly outpace conventional methods in speed, maintaining high simulation accuracy. NVIDIA Modulus's surrogate models cater to diverse applications, such as weather prediction, power plant modeling, cardiovascular simulation, additive manufacturing, and aerodynamic evaluation. Modulus is accessible as open-source software under the Apache 2.0 license, fostering the expansion of the scientific machine learning community.
-
10:20 - 10:50 am EDTCoffee Break11th Floor Collaborative Space
-
10:50 - 11:20 am EDTMachine Learning and Reduced Order Modeling for Large-Scale Industrial Digital Twins11th Floor Lecture Hall
- Speaker
- David Knezevic, Akselos
- Session Chair
- Marta D'Elia, Pasteur Labs. and Stanford University
Abstract
Akselos provides Digital Twins of industrial equipment in a range of industries, such energy, renewables, mining, and aerospace. The Akselos platform is based on RB-FEA, which is a unique combination of the Reduced Basis method for fast reduced order modeling of parametrized PDEs, with a domain decomposition framework that enables large-scale component-based analysis. RB-FEA shares many similarities to supervised learning approaches, in which "full order" solutions are used as the "supervisor" during RB-FEA training. In this presentation we will discuss the similarities and differences between RB-FEA and other ML methods, and demonstrate applications of the methodology to industrial Digital Twins.
-
11:30 am - 12:00 pm EDTA local, low-dimensional Machine Learning based PDE Solver for modeling engineering applications11th Floor Lecture Hall
- Speaker
- Rishikesh Ranade, ANSYS
- Session Chair
- Marta D'Elia, Pasteur Labs. and Stanford University
Abstract
In this talk, I will introduce a semi-supervised Machine Learning (ML) approach to model PDEs across a variety of engineering applications. The local, low-dimensional ML-Solver solves PDEs on multi-resolution meshes which are significantly coarser than the computational meshes used in typical engineering simulations. A coarse ML-Solver element, known as a subdomain, is representative of hundreds or even thousands of computational elements. The PDE solutions as well as other PDE conditions such as geometry, source terms and BCs are represented by n-dimensional latent vectors on each subdomain. The transformations between latent vectors and solution or condition fields on computational elements within a subdomain are learnt using field neural networks. Additionally, spatial, and temporal flux relationships between neighboring subdomains are learnt in the latent space using flux conservation neural networks and time integration neural networks. All the NNs are trained using data generated from engineering simulations. This talk will delve further into the nuts and bolts of the ML-Solver and demonstrate it across a variety of engineering use cases from fluids, thermal and mechanical applications. Finally, this talk will also demonstrate a use case of the ML-Solver that shows the potential of development towards a foundation model which can be used across a wide range of applications with consistent physics.
-
12:10 - 2:00 pm EDTLunch/Free Time
-
2:00 - 2:30 pm EDTQuasi Real-Time Simulations with Neural Networks for Industrial Applications11th Floor Lecture Hall
- Speaker
- Yu-Chin Chan, Siemens Technology
- Session Chair
- George Karniadakis, Brown University
Abstract
From reduced order models to PINNs and neural operators, the exponential growth of neural network techniques has shifted industry toward a new paradigm: scientific machine learning (SciML). However, industrial use cases are becoming increasingly complex while demanding faster, near real-time turnaround, necessitating the adaption of SciML to drastically more difficult problems. At Siemens Technology, we are researching the latest machine learning advances to develop solutions for our businesses and products. In this talk, we present an overview of interesting applications of SciML for Siemens, and our recent work on accelerating digital twins for a variety of use cases, including inverse heat exchanger control, quasi real-time prediction of airbag deployment, and neural design optimization in large design spaces. Through these examples, we also share some challenges in applying SciML to industrial applications.
-
2:40 - 3:10 pm EDTEnforcing Structure in Scientific Machine Learning for Computational Decision Support & Digital Twins11th Floor Lecture Hall
- Speaker
- Karthik Duraisamy, University of Michigan
- Session Chair
- George Karniadakis, Brown University
Abstract
This talk will begin with a brief snapshot of two case studies of the use of SciML in an industrial context, followed by a vision for computational decision-support and digital twins. Some recent developments in SciML will be reviewed, and an attempt will be made at providing a structured perspective on the current, somewhat chaotic landscape of modeling approaches. Following this, I will discuss various demands placed on models in scalable industrial applications, and opportunities for integration of structure within machine learning models will be highlighted. Particularly, I will touch upon two aspects: a) Introduction of physics constraints and adaptivity in reduced order models to greatly enhance predictive accuracy in complex problems, and b) Use of conditional parameterization to enforce mathematical structure and to sensitize models to secondary inputs.
-
3:20 - 3:50 pm EDTCoffee Break11th Floor Collaborative Space
-
3:50 - 4:20 pm EDTPhysics Constrained Machine Learning for Scientific Computing11th Floor Lecture Hall
- Virtual Speaker
- Danielle Robinson, Amazon Web Services AI Labs
- Session Chair
- George Karniadakis, Brown University
Abstract
In this talk, we discuss the development of physically-constrained machine learning (ML) models that incorporate techniques from scientific computing for learning dynamical and physical systems with applications in epidemiology and fluid dynamics. We first study the lack of generalization of black-box deep learning models for ODEs with applications to COVID-19 forecasting and the need for incorporation of advanced numerical integration schemes. We then focus on learning a physical model that satisfies conservation laws which are ubiquitous in science and engineering problems ranging from heat transfer to fluid flow. Violation of these well-known physical laws can lead to nonphysical solutions. To address this issue, we propose a framework, which constrains a pre-trained black-box ML model to satisfy conservation by enforcing the integral form from finite volume methods. We provide a detailed analysis of our method on learning with the Generalized Porous Medium Equation (GPME), a widely-applicable parameterized family of PDEs that illustrates the qualitative properties of both easier and harder PDEs. Our model maintains probabilistic uncertainty quantification (UQ), and deals well with shocks and heteroscedasticities. As a result, it achieves superior predictive performance on downstream tasks, e.g., shock location detection. Lastly, we study how to hard-constrain Neural Operator solutions to PDEs to satisfy the physical constraint of boundary conditions on a wide range of problems including Burgers’ and the Navier-Stokes’ equations. Our model improves the accuracy at the boundary and better guides the learning process on the interior of the domain. In summary, we demonstrate that carefully and properly enforcing physical constraints using techniques from numerical analysis results in better model accuracy and generalization in scientific applications.
-
4:30 - 5:00 pm EDTScaling SciML Algorithms for high-dimensional PDEs11th Floor Lecture Hall
- Speaker
- Somdatta Goswami, Johns Hopkins University
- Session Chair
- George Karniadakis, Brown University
Abstract
Predicting real-time complex dynamics in physical systems governed by partial differential equations (PDEs) is challenging due to the computational expense of conventional numerical simulations. Neural operators offer a promising solution by approximating mappings between infinite-dimensional Banach spaces. However, their performance diminishes with increasing system size and complexity when trained on labeled observational data from high-fidelity simulators. In this presentation, I introduce a novel method for learning neural operators in latent spaces, enabling real-time predictions for systems with highly nonlinear and multiscale dynamics on high-dimensional domains. Leveraging the DeepONet architecture, the approach operates on a low-dimensional latent space encoding the dynamics of interest to efficiently and accurately approximate underlying operators. The efficacy of this approach is demonstrated across various real-world problems such as material fracture, stiff chemical kinetics, and global-scale climate modeling. Our method surpasses state-of-the-art techniques in prediction accuracy while significantly enhancing computational efficiency, thus enabling operator learning on previously infeasible scales.
-
5:10 - 6:40 pm EDTReception11th Floor Collaborative Space
Sunday, March 24, 2024
-
9:00 - 9:30 am EDTEfficient optimization for simulation and real-world applications11th Floor Lecture Hall
- Speaker
- Eytan Bakshy, Meta
- Session Chair
- Alireza Doostan, University of Colorado Boulder
Abstract
Simulations and physical experiments are often resource intensive, and may take days or weeks to obtain estimates of a particular design under consideration. Bayesian optimization is a popular approach to solving such problems. I will discuss recent advances in Bayesian optimization and open source software developed at Meta to solve complex scientific and engineering problems, including methods for high-dimensional optimization, combinatorial, multi and many-objective optimization, and targeting long-term outcomes with short-term experiments. I will present these methods in the context of applications to inverse design of optical components, concrete formulations, and machine learning systems.
-
9:40 - 10:10 am EDTPhysics Informed Machine Learning for Optimized Product Performance11th Floor Lecture Hall
- Speaker
- Juan Betts, PredictiveIQ
- Session Chair
- Alireza Doostan, University of Colorado Boulder
Abstract
The maintenance and sustainability profile of machines in industrial applications is of critical importance to their field performance. In many instances machine failure modes are dominated by physics and chemistry-based processes such as fatigue, wear, and corrosion. Moreover, these processes typically have measured data for analytics algorithms to predict these failure events. Physics informed machine learning (PIML) techniques seem ideal for these types of problems given that physics can compensate for the lack of data and the fact the physical-chemical processes dominate these failure mechanics. In this paper, we discuss the development of an engine PIML thermo-mechanical (TMF) PIML model and its corresponding validation with test and field data. This paper also discusses extension of these models to wear and oil health applications.
-
10:20 - 10:50 am EDTCoffee Break11th Floor Collaborative Space
-
10:50 - 11:20 am EDTPerspectives and Practice for Scientific Machine Learning in Process Systems: Dynamic Surrogacy and Causal Discovery11th Floor Lecture Hall
- Speaker
- Jordan Jalving, Pasteur Labs
- Session Chair
- Alireza Doostan, University of Colorado Boulder
Abstract
Advances in Scientific Machine Learning (SciML) are poised to have profound impact within engineering domains that deal with industrial control and process systems (ICPS). Such systems are characterized by the operation of interconnected physical equipment; complex control and data acquisition systems; and sensor data that is nonlinear, noisy, and multi-dimensional. Consequently, maintaining reliable and predictable operation of an ICPS is challenging and often relies on robust advisory systems and expert operator decisions. SciML methods thus offer unique opportunities to develop new emulation and analysis tools that can ultimately enhance and redefine ICPS operation and decision making activities. This talk presents our SciML developments and experiences in using data-driven surrogates and causal discovery methods within the ICPS paradigm. We first discuss perspectives on the use of dynamic surrogate architectures to develop real-time ICPS emulators and how they facilitate effective simulation life-cycle development versus traditional emulator approaches. We further demonstrate how dynamic surrogate models naturally enable state-of-the-art numerical optimization and model predictive control capabilities. Next, we present challenges and opportunities toward utilizing causal discovery methods in the context of monitoring and fault analysis. We discuss how causal discovery methods provide a natural framework to both assist operators in understanding complex system behavior and to help diagnose root causes of faults without requiring a pre-existing historian of faulty operating conditions. We lastly use a benchmark chemical process dataset (the Tennessee Eastman Process) to demonstrate our results.
-
11:30 am - 12:00 pm EDTWhen big networks are not enough: physics, multifidelity and kernels11th Floor Lecture Hall
- Speaker
- Panos Stinis, Pacific Northwest National Laboratory
- Session Chair
- Alireza Doostan, University of Colorado Boulder
Abstract
Modern machine learning has shown remarkable promise in multiple applications. However, brute force use of neural networks, even when they have huge numbers of trainable parameters, can fail to provide highly accurate predictions for problems in the physical sciences. We present a collection of ideas about how enforcing physics, exploiting multifidelity knowledge and the kernel representation of neural networks can lead to significant increase in efficiency and/or accuracy. Various examples are used to illustrate the ideas.
-
12:10 - 2:00 pm EDTLunch/Free Time
-
2:00 - 2:30 pm EDTTowards 3D Interactive Design Exploration via Neural Networks11th Floor Lecture Hall
- Speaker
- Victor Oancea, Dassault Systemes
- Session Chair
- Panos Stinis, Pacific Northwest National Laboratory
Abstract
Physical simulations are performed at multiple scales covering diverse physical domains. Macro scale continuum simulations at part and assembly level, usually employing traditional numerical techniques such as 3D Finite Elements or Finite Volumes, are widely used for physics-based product design because of their high predictiveness value. However, more often than not these simulations are compute-intensive and require minutes/hours/days to execute. Starting from these computationally expensive 3D models, surrogate models massively reduce execution times to seconds (or interactive times), allowing a high number of parameter evaluations in the design space. However, these surrogate models are often of lower fidelity and only provide limited information through a few scaler KPIs (e.g., max stress, max pressure, max intrusion, etc.). To retain the richness of 3D simulation results while significantly reducing executing time, this paper presents a Neural Networks-based approach with the ultimate aim to enable 3D quasi-interactive design exploration . Multiphysics-multiscale traditional simulations [1] are used as the starting point: a) FEA analyses of structural statics, dynamics, manufacturing, packaging and safety, and b) CFD analyses– are used as Design of Experiments (DOEs) to generate the parametric design data. The data is processed and used to train fast executing neural networks as 3D Surrogates. Neural network algorithms and architectures (deep feed forward networks, recurrent and recursive nets, etc.) [2] are chosen depending on the nature of the physics. The trained neural network models can be deployed in a collaborative design environment for interactive design explorations. The proposed approach extends traditional model surrogates to cover both transient physical responses and 3D fields, which could enable an information rich much more productive environment for product design.
-
2:40 - 3:10 pm EDTBiological Research and Space Health Enabled by Machine Learning to Support Deep Space Missions11th Floor Lecture Hall
- Virtual Speaker
- Lauren Sanders, NASA
- Session Chair
- Panos Stinis, Pacific Northwest National Laboratory
Abstract
A goal of the NASA “Moon to Mars” campaign is to understand how biology responds to the Lunar, Martian, and deep space environments in order to advance fundamental knowledge, reduce risk, and support safe, productive human space missions. Through the powerful emerging approaches of artificial intelligence (AI) and machine learning (ML), a paradigm shift has begun in biomedical science and engineered astronaut health systems, to enable Earth-independence and autonomy of mission operations. Here we present an overview of AI/ML architecture support deep space mission goals, developed with leaders in the field. First, we focus on the fundamental biological research that supports our understanding of physiological responses to spaceflight, and we describe current efforts to support AI/ML research including data standardization and data engineering through FAIR (findable, accessible, interoperable, reusable) databases and the generation of AI-ready datasets for reuse and analysis. We also discuss robust remote data management frameworks for research data as well as environmental and health data that are generated during deep space missions. We highlight several research projects that leverage data standardization and management for fundamental biological discovery to uncover the complex effects of space travel on living systems. Next, we provide an overview of cutting-edge AI/ML approaches that can be integrated to support remote monitoring and analysis during deep space missions, including generative models and large language models to learn the underlying biomedical patterns and predict outcomes or answer questions during offworld medical scenarios. We also describe current AI/ML methods to support this research and monitoring through automated cloud-based labs which enable limited human intervention and closed-loop experimentation in remote settings. These labs could support mission autonomy by analyzing environmental data streams, and would be facilitated through in situ analytics capabilities to avoid sending large raw data files through low bandwidth communications. Finally, we describe a solution for integrated, real-time mission biomonitoring across hierarchical levels from continuous environmental monitoring, to wearables and point-of-care devices, to molecular and physiological monitoring. We introduce a precision space health system that will ensure that the future of space health is predictive, preventative, participatory and personalized.
-
3:20 - 3:50 pm EDTCoffee Break11th Floor Collaborative Space
-
3:50 - 4:20 pm EDTPhysics-infused ML for Environmental Monitoring11th Floor Lecture Hall
- Speaker
- Haruko Wainwright, MIT
- Session Chair
- Panos Stinis, Pacific Northwest National Laboratory
Abstract
Environmental monitoring – traditionally relied on collecting point samples – are undergoing transformational changes with new technologies such as remote sensing, in situ sensors and various imaging techniques at different scales. At the same time, environmental simulation capabilities are advancing rapidly, predicting environmental flow and contaminant transport in complex systems and quantifying its uncertainty. However, there are still significant challenges to integrate these multi-type multiscale datasets with model simulations. In particular, these datasets are often indirectly correlated with the variables of interest, and have different scales and accuracies. Simulation results are often not perfect due to natural heterogeneities or fine-scale processes not captured in conceptual models. The Advanced Long-term Environmental Monitoring Systems (ALTEMIS) project aims to establish the new paradigm of long-term monitoring of soil and groundwater by integrating these new technologies through machine learning (ML). This talk highlights the two new developments, involving groundwater flow and contaminant transport simulations. First, I will talk about an emulator based on the Fourier neural operator, considering the uncertainty in subsurface parameters and climate forcing. This emulator aims to enable the off-line assessment of future climate change impacts on residual contaminants. Second, I will introduce a Bayesian hierarchical approach coupled with Gaussian process models to integrate in situ sensor data, groundwater sampling data and ensemble simulations. It enables us to infuse physics –such as flow direction and contaminant mobility – into the spatiotemporal characterization of contaminant plumes. Lastly, I will discuss the pathway to actual deployment with the understanding of environmental regulations and site needs, as well as the feedback mechanism to advance deployable technologies
-
4:30 - 5:00 pm EDTLeveraging Large Language Models for Scientific Machine Learning across Domains11th Floor Lecture Hall
- Speaker
- Adar Kahana, Brown University
- Session Chair
- Panos Stinis, Pacific Northwest National Laboratory
Abstract
Large Language Models (LLMs) have rapidly emerged as transformative tools in the landscape of scientific inquiry, heralding a new era of interdisciplinary innovation. Our presentation at MIT will embark on a journey through the evolution and significance of LLMs, starting with a brief overview to lay the foundational understanding necessary for appreciating their impact. We will then navigate towards the specific application of LLMs within the realm of Scientific Machine Learning (SciML), illuminating how machine learning techniques are revolutionizing scientific research by examining the practical approaches and methodologies adopted by researchers to address complex SciML challenges. A focal point of our discussion will be the role of simulations in Mechanical Engineering, where we will showcase examples and scenarios that highlight the superior solutions enabled by machine learning. This exploration will not only provide a comprehensive insight into the current state of play but also set the stage for considering LLMs as dynamic agents of change, surveying the latest and greatest research articles employing those agents. We will then try to break the ceiling of 'agent'/'assistant' by discussing General AI (GenAI) and its burgeoning potential to further empower SciML applications directly, and not as orchestrators. Concluding our session, we will venture into speculative territory, pondering the future landscape of the industry as it is shaped by the guiding hand of LLMs in steering research and development towards uncharted territories of knowledge and innovation.
All event times are listed in ICERM local time in Providence, RI (Eastern Daylight Time / UTC-4).
All event times are listed in .
ICERM local time in Providence, RI is Eastern Daylight Time (UTC-4). Would you like to switch back to ICERM time or choose a different custom timezone?
Request Reimbursement
This section is for general purposes only and does not indicate that all attendees receive funding. Please refer to your personalized invitation to review your offer.
- ORCID iD
- As this program is funded by the National Science Foundation (NSF), ICERM is required to collect your ORCID iD if you are receiving funding to attend this program. Be sure to add your ORCID iD to your Cube profile as soon as possible to avoid delaying your reimbursement.
- Acceptable Costs
-
- 1 roundtrip between your home institute and ICERM
- Flights in economy class to either Providence airport (PVD) or Boston airport (BOS)
- Ground Transportation to and from airports and ICERM.
- Unacceptable Costs
-
- Flights on U.K. airlines
- Seats in economy plus, business class, or first class
- Change ticket fees of any kind
- Multi-use bus passes
- Meals or incidentals
- Advance Approval Required
-
- Personal car travel to ICERM from outside New England
- Multiple-destination plane ticket; does not include layovers to reach ICERM
- Arriving or departing from ICERM more than a day before or day after the program
- Multiple trips to ICERM
- Rental car to/from ICERM
- Arriving or departing from airport other than PVD/BOS or home institution's local airport
- 2 one-way plane tickets to create a roundtrip (often purchased from Expedia, Orbitz, etc.)
- Travel Maximum Contributions
-
- New England: $350
- Other contiguous US: $850
- Asia & Oceania: $2,000
- All other locations: $1,500
- Note these rates were updated in Spring 2023 and superseded any prior invitation rates. Any invitations without travel support will still not receive travel support.
- Reimbursement Requests
-
Request Reimbursement with Cube
Refer to the back of your ID badge for more information. Checklists are available at the front desk and in the Reimbursement section of Cube.
- Reimbursement Tips
-
- Scanned original receipts are required for all expenses
- Airfare receipt must show full itinerary and payment
- ICERM does not offer per diem or meal reimbursement
- Allowable mileage is reimbursed at prevailing IRS Business Rate and trip documented via pdf of Google Maps result
- Keep all documentation until you receive your reimbursement!
- Reimbursement Timing
-
6 - 8 weeks after all documentation is sent to ICERM. All reimbursement requests are reviewed by numerous central offices at Brown who may request additional documentation.
- Reimbursement Deadline
-
Submissions must be received within 30 days of ICERM departure to avoid applicable taxes. Submissions after thirty days will incur applicable taxes. No submissions are accepted more than six months after the program end.